#5: Identity Hijacking: The fight against AI fraud - Vijay Balasubramaniyan
Vijay Balasubramanian:
Generative AI is completely upended because of how good you can create a likeness of someone's voice, face, video. Crazy how quickly the landscape has changed. So when we started PinDrop, we were focused of solving the right human problem. You need technologies like this with other platforms too, social media, right? Like where you see a lot of this content. You need the clear ability to say that this was AI generated.
Ian Krietzberg:
Welcome back to the Deepview Conversations. I am your host, Ian Kreitzberg, and we've got a really fun episode for you today. My guest is Vijay Balasubramanian. He is the CEO and co-founder of Pindrop. Now, Pindrop is a cybersecurity company. They've been around long before generative AI, but of course, today we're talking about them and their relationship to generative AI. Now, when Pindrop first got started, their whole focus was on vocal authentication, right? So if I were to call my bank, their MO was figuring out that I was the right person, that I was in. that I was who I said I was. The reality that generative AI kind of kicked off is one of deepfakes, one where you can't trust what you see. And since we live in this kind of digital world, things to the human ear, to the human eye, are often hard to distinguish from synthetic generation versus organic digital reconstruction, right? Is this a video of a real person? Or is this a deepfake generated by any number of deepfake companies? And so in that kind of environment where you can't really trust what you're seeing, and that distrust is especially paramount in certain enterprises, financial and kind of banking institutions are a prominent one that come to mind there where you have to know Not only is this person the right person, but are they a real person? And that's the kind of switch that Pindrop made where, you know, through deep learning technologies, they have leveraged their expertise in authentication and brought it over to this age of, can we now use this technology to determine whether or not a human said these words, whether or not a human is on the other end of the phone, or is it an artificial intelligence, a generative artificial intelligence system. So what we get into is this really fascinating breakdown of the kind of core of artificial intelligence research, which has to do with linguistics, and the the minute but highly impactful differences between the the way that you and I speak, and the way that a digital synthetic chatbot generates sounds, generates voices, the way these deep fakes work. And it's not something that's discernible to the human ear, but it's very clear, specific, distinct anomalies that are discernible to PinDrop's models. So all that to say, This was a fascinating conversation. I think we'll get a lot out of it. Let's get into it. Vijay, it's so good to see you. Thank you so much for joining us today.
Vijay Balasubramanian:
Absolutely happy to be here, Ian.
Ian Krietzberg:
Great. So to me, there's so much to get into, right? This is all so interesting. The place I want to start. right, is Pindrop is a cybersecurity company. You guys have been around long before the kind of boom of generative AI that we've been seeing the past couple of years, but there was a change, right? About two years ago, you know, ChatGPT launches, it sets off this huge AI race from the public's perspective, and all of a sudden we have generative AI. We have a mix of open source, we have closed source, we have models that create output in the form of audio, and video and images and texts. And to a degree, it's fun to play with, right? But I think immediately and probably from your perspective, there's an immediate implication on the cybersecurity side and what this means for fraud. And so I'm wondering, right, how that landscape from your perspective has kind of changed and evolved in just the past couple of years is these things have seemingly gotten more powerful and by that really I mean just more realistic and more kind of all over the place, right?
Vijay Balasubramanian:
Yeah, it's crazy how quickly, you know, the landscape has changed. So just to give you some sense, right, like for the longest time, you know, when we started PinDrop, we were focused in a remote first world of solving the right human problem. So, you know, Ian, you're trying to, you know, talk to your bank, to your healthcare provider, buying a laptop off Amazon like whatever the situation may be when you have a remote interaction we're trying to make sure it's Ian and not you know someone else trying to be you maybe not your family members and so on. Two years back what changed is from that being the most important problem, it quickly changed to the right human problem changed to the real human problem. So is it even a real human that you're interacting with? And the reason for that is that When we started, and we've been doing deepfake detection work for a long time, but when we started about eight years back, there was one tool on the voice cloning side. It was called Liarbird. It was a great tool, but it would take a significant amount of audio. So it would take hours of recording. And even, for example, when John Legend became the voice of Google Home, They required like 20 hours of him recording his voice, plus things that they already had of him, but 20 hours of content to actually allow Google Home to, in John Legend's lilting voice, say the weather in San Francisco is a balmy 36 degrees. All of that changed to now you have from that one tool at the end of last year, there were 120 tools that could clone your voice. And then from the end of last year to March of this year, there are 358 tools that can clone your voice. So one, just the sheer amount of choice changed. But also the speed, what used to take 20 hours to clone your voice, now you need anywhere between 3 to 5 seconds to clone your voice. And the tools, if you need a really high quality version of your voice, you need 15 seconds. So you've gone from 20 hours and one tool to 350 tools. You can record your voice in many, many different languages, over 40 different languages. And all it requires is three seconds of your voice.
Ian Krietzberg:
Yeah, and I know all this, right? And it still mind boggles me every time I hear it. And I've been defaked a couple times in sort of experiments, right, along the way as we're going. And it is remarkable. And it's one of those things that it kind of has to happen to you, hopefully in a controlled environment for you to understand what you're saying where, yeah, A few seconds of a recording, or if you have out there a video that a friend took of you and posted on Facebook, and you might think you don't have any audio to train on, but that is alone enough. that it can be created into this kind of voice clone, which then can be weaponized, and we've seen it weaponized. It's interesting, right, you were saying that when Pinterop got started, it was the kind of, are we authenticating, is this the right person, and now is this a real person? And those two questions still seem very related, right? Is it a fake person, you know, being purported by the wrong person, by some sort of fraudster? But that core of authentication, right? Why did you get into that? What inspired that kind of foundation of authentication is really important. I need to focus on this.
Vijay Balasubramanian:
Yeah, so it's interesting. You know, what started me on this journey was actually, you know, I was a PhD student at Georgia Tech. And once you start getting published, man, you go present. And so you need a suit to present because that's what PhD students do. You want a nice little suit to go present and get out of your lab and actually talk to the world. And so, you know, I couldn't afford a suit here in the US. So when I was traveling back to India, I got a suit because you know, anyways, if you buy a suit here too, it's probably made in India, right? And so, you know, so I went got the suit, gave it for alterations. And then, you know, the next day, I was busy getting ready to fly home. And What ended up happening is that night, the previous night after I'd given the suit for alteration at 3 o'clock in the morning, I get a call from my bank saying, hey, there is this transaction that's a little large. We need to verify it. And they called me at 3 o'clock in the morning because they didn't know I was in India. It was the fraud department. So the phone number that flashes on my screen is a New York-based number. And so I'm looking at it, pick up the phone, it's three o'clock, I'm super groggy, and then they're like, hey, there's this transaction, we need you to verify the transaction. I'm like, okay, tell me what the transaction is. They're like, before we give you more details, we need to verify you, so please tell us your social security number and your date of birth. And I'm like, I'm a security PhD researcher. And I'm like, at 3 o'clock in the morning, somebody is asking for my social security number. And I don't know who it is. I'm definitely not giving this out. And so we spent 30 minutes in this silly cat and mouse game where I was trying to find out who they were. They were trying to find out who I was. And then ultimately, after 30 minutes, I was tired. And I was like, you know what? Go ahead and cancel the transaction. It can't be that important. And I'll figure it out in the morning. And that morning, I was busy packing, shopping, and my suit company kept calling me. I just didn't pick up their phone. So at 12, when I was supposed to go pick up, I was like, hey, I'm heading in to come pick up my suit. And they said, oh, your credit card company declined your transaction. So we're not going. We stopped working on it. So you can't have a suit. So I flew back to the US without a suit. And I was really, really frustrated. And so I was like, man, we have existed for so long, and phone systems have existed for so long, that other than the fact that when your mom or your friends call you, you know who it is. But if it is a random phone call, you have no idea who's on the other end of that phone call. And these phone systems have existed since Alexander Graham Bell. So the fact that something as basic as identity could be established on a voice channel, that became the core of my PhD thesis. And what ended up happening is it was academic to start off with, but then we soon realized that every organization, when they interact with you on the phone, they actually have no clue who you are. They have very, very poor mechanisms to determine who you are. And therefore, it started becoming the core or the beginning of Pindrop's work.
Ian Krietzberg:
I have to imagine that that kind of story that you're telling, right, where your bank calls you, each of you are trying to figure out who the other person is, no one has any capacity to do that. I feel like even though that was, you know, a few years ago, that has to be such a pressing concern for so many enterprises today, even AI aside, but I think the AI component just makes it so much more pressing, the verification, especially in the banking side of things and the finance side of things. you know, is this my actual customer? Is this their actual transaction? Are these the kind of paramount concerns that, you know, when you talk to your enterprise customers and people right now, is this what they're concerned about? Identity verification, are their concerns super, you know, multifold beyond that? Or is that kind of the core of what you're seeing today?
Vijay Balasubramanian:
Yeah, that is, you know, the fundamental concern in a remote first world. You have to identify who's on the other end of an interaction. That could be in the online world. That could be in the voice world. And you know, oftentimes, your most complicated transactions, oh, I'm buying a new house. I don't know how to apply for a mortgage. let me talk to someone, or hey, I finally got a job and I want a life insurance because I'm going to have a kid and I think it's important. You know, these really, really complicated questions, you actually have voice conversations even now. And so in a remote first world, determining identity is the first thing, right? Like, if I don't know who you are, I can't tell you anything about your bank account. I can't tell you anything about your health care records. I can't tell you anything about, you know, your insurance policies that you have. And so it becomes almost a fundamental requirement. And the first requirement that these organizations have. So if you look at financial institutions, for example, 90% plus of their interactions require identity as the first thing that gets established. Unless you're asking for hours of a bank, which is increasingly rare. any any transaction will require identity and so it's become the most fundamental question and the traditional way of asking figuring who you are is by something you know so let me ask you a bunch of questions that my hope is only you know to be able to determine you are right like what was the color of your car what is your date of birth what's your mother's maiden name and so on and so forth but you know with the there were 1800 data breaches last year you know 60 percent of Americans have their data compromised so all of these answers are available or you know you go to meta or one of these things you can get all kinds of answers about you and so they were traditionally you know these knowledge-based authentication questions were the ways for these organizations to determine who you are and With data breaches, it is absolutely impossible to differentiate between you and the fraudster because currently 92% of fraudsters know the answers to these questions. You're catching 8% of super lazy fraudsters who haven't done their homework, and they'll probably do their homework and come back and take your account over. And so it is really the fundamental question every organization grapples with. doesn't have great tools to answer this fundamental question.
Ian Krietzberg:
And it seems as though this is like, it's such a broad thing that you're talking about, right? And the implications, if you think about it for more than 30 seconds, you go, oh boy, right? Because you're right, it is a remote world, it is a digital first, and in some cases, digital only world, where it's hard to get a person on the other end of the phone. Not every institution has an in-person location that you can go to. It is digital first bleeding towards digital only. In the landscape you're talking about where you have people don't even think about their digital footprint and what exists out there that they may not know about that has those knowledge-based questions. answered, right? You know, they have a picture on Instagram of their first car, and that just gets sucked up in a web scrape somewhere and analyzed or something. Is the biggest kind of cybersecurity gap right now when you're thinking about that kind of ever-present need to verify identity? Is it generative AI? Is that a gap that just no one really knows how to plug yet? Or is it just one of many, many things that just are a kind of reality of living in a social media society?
Vijay Balasubramanian:
Yeah, I think it's the two that I highlighted before, right? Like one, how do you ensure it's a real human? which is where generative AI completely upended the issue. And then, is it the right human? Because you also need to establish not just is it human or machine. Once you've established it's human, am I talking to the right human? In which case, you need a whole bunch of mechanisms to determine, I'm really interacting with Ian. And not, for example, your significant other who might have access to a lot of your devices, your information, all kinds of other things, right? Or we see grandparent scams, family scams, first party fraud, and things like that. So it's these two problems. One is, is it a real human which generative AI is completely appended because of how good you could create a likeness of someone's voice, face, video, driver's license, whatever you might think of. And then the second is once you've established it's a real human, is it the right human? Is it really Ian and not someone else?
Ian Krietzberg:
Right. And now diving into that, right? I know that, and I've written about this before, and you and I have spoken about this before, that PinDrop has been working on and has launched solutions to make those determinations, to make those determinations of, is this a synthetic voice? Is this a real voice? And this is called PinDrop Pulse, right? And so I, I have a lot of questions about that that I want to kind of get into. And the first thing is that, you know, I know that this has been the kind of result of about a decade of research. Was this always the plan? Was the focus, was it, or was it like a back burner thing that you realized when AI started coming out? Oh, we have the tools to do this. Are you always thinking this is where it's headed. We we're going to need eventually some way to verify if voice is a human organic voice or if it's a digital synthetic replication.
Vijay Balasubramanian:
Yeah. So, you know, it would be awesome if that was always the plan, man. I would, you know, I'd be Nostradamus or, you know, some really prescient being. But no, that wasn't right. Like at Pindrop, we're always incredibly innovative. So we're always, you know, saying, hey, if this keeps going, what is likely to happen? And it was one of those channels of thought that said, oh, you know, if there's tools like Liarbird could exist, what is the logical end state of this? And, you know, we didn't know it was going to be generative AI, right? But we knew it's going to become more and more likely for people to replicate your voice, your likeness. What has truly been surprising, even before generative AI came, I remember when Anthony Bourdain, there was a documentary of him called Roadrunner. The director used a lot of audio processing tools like Liarbird and a whole bunch of capabilities to actually replicate his voice after he was dead. And they released one of his synthetic voices in a trailer, and he got a lot of flack. And then he decided not to showcase the other pieces of the audio. And then we were brought in to find out the other pieces. And we discovered 50 seconds of audio that was generated and not Anthony Bourdain. And that created a lot of great press cycle for Pindra. But even then, we continued working on this area and building patents in this area. And what just changed or what surprised us is just the speed of these systems. Once you figured out these deep neural networks, these GANs that allow you to iterate so many times to get to either crazy human likeness on voice, video or face, what surprised us is how good they got, how quickly. and just the sheer explosion of them. And then what surprised us even more is the speed with which attackers adopted this technology. So, you know, chat GPT came out two years back, we thought, oh, man, like, you know, there's going to be deep fake attacks. So let's start monitoring our customer interactions to see is deep fake being used. And in all of 2023, we'd see like one attack a month across our entire customer base. So we'd see that one attack and then we'd be like, oh, man, this is interesting. They're slowly getting better. And then this year, it was crazy. Like right now, we're seeing an attack a day per customer. And there are certain customers who are seeing an attack, a deepfake attack every three hours. These are really, really large institutions. And so that has meant a 1,400% increase in deepfake and synthetic attacks just in the first six months of this year as compared to all of last year. So when we actually look at the entire year in totality, that number, the 1400% number will be much, much higher. But right now itself, after the first six months, it's already 14% explosion of these attacks. So, it's not just that these AI tools have exploded, it's the sophistication of attackers using these tools and using it to take over accounts and scale their operations at unimaginable levels that has truly, truly surprised us.
Ian Krietzberg:
Those numbers are insane. It's interesting, I guess, the pace of adoption, right? Where 2023 was more quiet and 2024 is just explosive. And this is what we see, right, when you're talking about the cybersecurity implications and the fraud, and it's not just companies, it's people too, of increased scale where these threat actors can now They've been doing this forever. Fraudsters are going to fraud, right? But now they're capable of doing so much more at such a broader scale. And I think the point that maybe doesn't get enough attention is that it's all hyper-personalized. And that's why it's so dangerous, right? It's specified, the attacks are constructed based on profiles of people that are created so that someone who might be not as susceptible to this kind of attack might be more susceptible if it pretends to be whatever, a banking institution or whatever. So those numbers are crazy. Do you expect that trend to kind of keep moving and in what seems like a semi exponential manner? Yeah, for sure.
Vijay Balasubramanian:
Because, you know, and you've hit the nail on the head, right? Like it's a crazy combination of being able to scale, but also being able to personalize and customize it. So, you know, when you try to customize it for one, it usually means it doesn't scale. And here you have the best of both worlds. And we saw that, you know, so for example, when you saw, you know, the earlier this year, we were the ones who identified the Biden robocall that, you know, affected the Republican primary in New Hampshire. And we're the ones not just to, you know, identify it, but identify the AI application. And that's a case, you know, what worries me is the fact that you could go after, you know, that particular place that was holding primaries and create a, you know, a version of President Biden asking you not to vote. You could do that. in every county and create a message that's very specific to that county and so localize it to that county and scale that and so that is scary but the important aspect of it is why I think it's going to continue is fraudsters love the scaling ability. So, you know, if you come to PinDrop, every conference room is named after a fraudster we've caught. So we have Chicken Man and Pepe and Dava. And one of the rooms is called Williams, right? And so Williams is a fraudster out of West Africa. For the longest time, he used to employ 12 different people. And all those 12 different people in this fraudulent call center in West Africa would do is pick up the phone, call organizations taking over accounts and social engineering call center agents to take over these accounts. And so that's all they would do. And what we started seeing last year, or not last year, but this year, is Williams has started using AI to scale out his operations. So what that means is he no longer needs to employ 12 people. He's combining a large language model with a text-to-speech deepfake engine that every time a call center agent asks him a question, the large language model figures out the answer to the question, and then provides the answer in the voice that he wants. So it's a crazy combination of two different gen AI tools coming together. And the interesting thing is this. The biggest problem with large language models is hallucinations, right? Like they make shit up. But in a fraud use case, it's awesome. Because if you tell the large language model, make whatever reason you want to take over an account, it comes up with these crazy reasons. And the call center agent is sitting there saying, man, this is such a weird reason. This must be true, right? And so goes on and starts helping this person. So here is not only fraudsters using GenAI to scale, they're using bugs in GenAIs as features in their own world and it's crazy that if he can scale out, he is no longer restricted to the 12 people. He can just scale out based on the number of accounts that he wants to compromise.
Ian Krietzberg:
Wow. I mean, the first thing that that brings to mind for me, right, is that there's been this kind of ongoing debate, one of many in this field, about open source versus closed source large language models. And it's really split, where you have meta, who says they're open source, they're not really, they're more so open weights. I think the open source definition, like a lot of things in this field, is not super clear, but meta does not have its source code available. But they're more accessible. OpenAI is very clearly closed off, and other companies are like that as well. When you're talking about the capacity for a threat actor who now doesn't have to be also a computer scientist or a computer coder to just kind of take a large language model and develop that, would a closed source ecosystem across the board even prevent that or is it kind of the cat's out of the bag?
Vijay Balasubramanian:
No, the cat's out of the bag, right? I don't think the closed source versus open source matters here. Obviously, open source, I'm a big fan of open source, but in this particular case, there's a lot of open source tools. I'm not weighing in on the debate of open source versus closed source. I'm just telling facts, which is initially we used to see a lot of deep fake attacks from closed source tools, now we're seeing a lot more open source tools being used, because for a fraudster, that just means, hey, it's cheaper, it's easier access, and that's always the case. But I think open source is actually a good way, it doesn't matter whether it's closed or open source, I think in either of those cases, there is a requirement for Gen AI tools to add a layer of security within their own tools, right, which is, hey, how am I making sure that the person using this tool to generate stuff isn't using it for malicious purposes. So if I am creating a clone of Ian's voice, How am I making sure that I've gotten the right consent and that my own consent mechanisms haven't been beaten by another Gen AI tool? You have to make sure that you really invest a lot in the security of your tools because it's something that you're getting They have a lot of power. With a lot of power comes a lot of responsibility. That responsibility means building security features within your own tools. to avoid misuse. And then you'd still need tools to detect when misuse is happening. And so that's never going to change. But I think a lot of these players need to start getting a whole lot more responsible and a whole lot more secure in the way people are using their tools.
Ian Krietzberg:
And just circling back to a thing that I think is just super interesting that I want to get into, you mentioned how you guys kind of discovered the Biden deepfake in New Hampshire. And I think before that, the work on the Anthony Bourdain show. So When we talk about, and we've talked about a few times already, right? The kind of identification of, is this a real person? Is this a synthetic person? How does your system do that? What's going on in your system that it's able to say with 98% certainty, this is really Vijay speaking versus, you know, this is absolutely a robot.
Vijay Balasubramanian:
Fundamentally, when we're looking at things like audio, what we're looking for is anomalies on the frequency or the time side of things. What I mean by frequency is when you speak, the way you say certain things has certain harmonics and cadences to it. And so looking at those frequencies is one example. So for example, when you say San Francisco, the word San and Francisco, the F and S is actually you channeling noise into actual words. So it's the SH turned into S or F. And so that's something you can do as a human, largely because over 10,000 years of you speaking, during this period in time, you also cultivated soft fruit. So you developed an overbite, which essentially meant your jaws could move one on top of each other. So you could create these kinds of nodes, which is something, you know, when a system doesn't have a physical production, it doesn't care about these and these are, you know, characteristics in the high frequency range. So what ends up happening is they don't pay attention to it because it is not important or it's too much effort to pay attention to these subtle things because these subtle things your ear doesn't pick up on, right? Like it glosses over these things. And so oftentimes what we'll find is the way they're pronouncing these fricatives or other phonemes are very unique to the deep fake engine. And therefore we can pick up on that. An even more interesting characteristic is what is known as a temporal characteristic, which is, how is your voice changing over time? And therefore, what is your physical changes in your vocal tract, in your nasal cavities, in order to enunciate what you're trying to say? So for example, when you say, hello, Paul, my mouth is wide open when I say hello. And then my mouth shuts down when it says Paul. There's only a certain speed with which I can do that. These machines don't have physical limitations, so they don't care about those speeds. And so they do it in all kinds of weird ways. So one of the conference rooms is called Giraffe Man, because every time we analyze the audio of this fraudster, we're like, OK, the only human being that could have produced this kind of sound is someone with a seven foot long neck whose vocal cords are thrashing too rapidly between those seven foot configurations. And so that's why it's called Giraffe Man. But ultimately, all of this is very important, largely because when you're speaking and a digital channel is capturing your voice, even the lowest fidelity channel, which is call centers, has 8000 samples of your voice every single second. And that means there are 8,000 times for a generative AI machine to make a mistake. And that's what we're picking up on. And because there's so many opportunities, you catch a lot of these anomalies. We're on a Riverside podcast. Most of these online systems have 16,000 hertz. So that means there are 16,000 samples of your voice every second. Music has 44,000 hertz. So you have 44,000 samples of your voice. So there's just a lot of information every single second. And the machine gets it wrong very often. And so we are picking up on those. And the fact is that that machine doesn't even care about those mistakes, because those mistakes are not important for it to convince your human ear. and that's where we pick up on a lot of these anomalies. Does that help?
Ian Krietzberg:
Yeah, yeah. It's such an interesting thing, the kind of explanation, right, of the overbite and the kind of organic formation and construction of our speaking mechanisms and the way that humans create the sound that we communicate. When you're talking about the research that you did, that you kind of built on and that you pursued to create these kinds of tools, it seems like there's a big role of linguistics, just pure and simple linguistics of How do we differentiate between what's human and what's not human? You need to understand human linguistics. And that's just interesting to me because the core of artificial intelligence research, a lot of it is just linguistics work, right? So was that the case?
Vijay Balasubramanian:
Yeah, you know, it's super interesting. You're absolutely right. It's linguistics. It's phonemes. And we often find, right, like our ability to catch that, right? Like, we also use deep learning systems within our own end to do deep fake detection. But when we unravel it and start understanding why are we catching certain things, you're absolutely right it is linguistics and it's fundamentally our very own human being right like we have all of these crazy imperfections man which make us human but that also allows us to differentiate between a machine which is often too perfect
Ian Krietzberg:
Right. Human imperfection is what will always set us apart. You mentioned before, right, that these things are improving so quickly, right? The rate of that improvement and that adoption kind of surprised you. How the hell do you guys stay ahead of the curve when it seems like everyone from publicly traded companies to scammers in a basement somewhere are trying to make systems more capable of fooling you?
Vijay Balasubramanian:
So you know, I think it's all based on the asymmetry of generation of deepfakes versus detection of deepfakes. So for us to detect the universe of deepfakes right now, it is four orders of magnitude cheaper than a deepfake creation engine. So that means in order for us to detect a deepfake, it's four times cheaper or four orders of magnitude cheaper to detect that deepfake. And that asymmetry means as the deepfake engines get better, deepfake detection also gets better. And an intuitive way to think about it is for a deepfake generation engine to make you believe that this is real, it has to worry about your intonations, it has to worry about your disfluencies, when do you say um, ahs, when are you taking pauses, how do you take pauses, what is your emotion, what is your tonality, all of that. All we have to do is find one mistake. And so it's that asymmetry between detection. And this asymmetry has always existed in security, right? Like, not that it's always existed. Every time security has won, the asymmetry has existed. So for example, there was a point in time, I remember when I first got into security, the big problem of the day was email security. So there was spam, right? tons of spam. 95% of all emails that you would get was spam. So it was painful. And so then the spam engine started creating spam detection. And there was this race. But then it became so hard to avoid the spam detection engines that they would hide it in images. And then the images won't render and they would do all kinds of things. And ultimately, spam detection became so good. And it's one of the reasons the systems that invested a lot in spam detection, like Gmail and things like that, won out against email behemoths that used to exist before, like Yahoo and Hotmail. Like Gmail, with its big storage, clean inboxes, one out. And so it's something that's always existed. In security, you've always got to find the asymmetry that's going to keep you ahead. You're never going to stay ahead at a moment in time. But as long as that asymmetry exists in the long run, you will always stay ahead. And that's, we think we have found that asymmetry, right, which is detection is so much cheaper than generation and for a very intuitive reason that they're going to improve and so are we, but the cost of our improvement is not going to be quite the cost of theirs. And as long as that's the case, and as long as we continue to sell more customers, have more funding and stuff like that, we'll continue to stay ahead.
Ian Krietzberg:
That's a really interesting point, and that actually answers a question I had for you down the line. So perfect, we're on the same wavelength. Something else that I've been kind of wondering about, the distinction that enables you to identify synthetic versus authentic organic human sound, the way our organic bodies produce the noises that we call words. Is there a world, and I guess this is theoretical, I don't know how much people are working on this, where through robotics and rubber and silicon and timing digital speakers to movements of robotic mouths that they can overcome that? Is that even a realistic, worth it expenditure?
Vijay Balasubramanian:
Yeah, I mean, It's possible, right? Like, and maybe Hollywood studios, when they're trying to recreate, you know, something pretty insane, they are. But that's the point, right? Like, if you don't make it scalable, you've won, because the average attacker is not going to have that. And there's going to be an attack that's cheaper, simpler to execute, rather than getting this crazy equipment in your basement and trying to get it to work, finding legal ways to procure it and do all of that. For sure, there are going to be people who do it and it's going to be incredible. But it's not going to be there for the average attacker. And that's all that matters. Because if it is not an attack that scales, then it means people's bank accounts are not at risk at large. You don't have elder citizens getting scammed every day, like in many, many of them. Any attack that doesn't scale is a win. Right now, the problem is these attacks scale and they allow attackers to get ahead and as long as you can change that dynamic, you've won.
Ian Krietzberg:
Right. Yeah, no, that's a good point. It's all about scale. These guys have to be as efficient as possible to keep their businesses operational. And so a point you mentioned earlier, right, where you would like to see more responsibility in the creation of these tools, open source, open weights, closed source, doesn't matter. You know, a kind of ethos unspoken, perhaps, of Silicon Valley, and I think we've seen a big resurgence of it, is the whole move fast, break things. paradigm where it mattered more and it matters more, I guess, still today to put the stuff out there, to get the applications out there, to get the muscle memory going of people who are using these things than it does to slow it down, to perhaps increase the latency by introducing mechanisms that would make it more secure. When we talk about integrating and building responsibility and security into those systems, Is there, are there technical ways that they could potentially do this? And I guess they're just choosing not to, or is it just very challenging to build cybersecurity into these systems?
Vijay Balasubramanian:
No, it's actually pretty easy because, you know, so for example, in our system, ours is a very simple API. So all you need to call is the API to then determine, okay, are you interacting with a real human or a machine, right? And so it's actually really simple. And, you know, we're deployed in eight of the top 10 banks, five of the top seven insurances, some of the biggest healthcare providers, biggest retailers. And so, you know, they're able to deploy it very easily because, you know, it's just You're calling an API, and in this day and age, calling an API is super simple and streaming audio or streaming video content is super simple. It's not a technical challenge. I think it's a prioritization challenge, which is, hey, when I am debating between – and this is the age-old challenge – do I develop new features, new functionality that gets me more revenue? Or do I take down my security debt? Oh my god, lots of bad people can get into my environment and do all kinds of bad things. But they're also paying customers. So maybe I'm a little bit complicit in that entire thing. OK, they're paying me. So what do I care? I'm not the one losing money. And so there's a little bit of that as well. But I think all of those things actually play out logically. There are certain companies that are doing incredible jobs from the ground up. Trying to be responsible right from the get go. They're saying, Oh, no, what we've created requires a certain amount of security and responsibility. So we're going to start incorporating that into our tools. And so they're doing this in a very responsible fashion. but there are other companies that are prioritizing other things and so but you know this always catches up to you right like any debt security debt catches up to you and It depends on how bad the situation is when it catches up to you. I remember eBay for the longest time was a great platform and fraud on eBay became so bad that they had to address it. And if they didn't address it, they would have... best trust and safety folks come out from eBay, in all these new companies, they run a lot of trust safety in new companies, because they went through the really bad situation that, oh, my God, there's a lot of fraud in our platform. And so each platform will go through that. The question is, can you get and address your security debt well before you get to that point? Or does that point shutter you down?
Ian Krietzberg:
I think a very relevant point that's been going on all the time in the undercurrent of what you're talking about, which is this prioritization of, do I want to focus on this or do I want to focus on the stuff that drives the revenue? A lot of people are going to choose the revenue. There's been these conversations about regulation and regulatory approaches, and it's still very early days, I guess, if you look at the US, right? There's nothing federally yet. I think anything federally that has any sort of attention on it is absolutely related to deepfakes. That's what seems to have grabbed people's attention more than anything else. And then state to state, it's just this kind of patchwork quilt, right? I feel like is the most apt analogy where California has these things, and Texas has these things, and New Jersey has these things, right? Which makes it really hard for compliance. But when we're talking about that side of things, governance and oversight and kind of recognitions of the impacts and the harm. Is there a regulatory approach that you think makes sense or is realistic to apply on kind of a broad
Vijay Balasubramanian:
I think, you know, the cool thing is we've been here before. So I talked about email spam. A great example is the CanSpam Act, which, you know, it was regulation, but it was regulation, it was the right kind of regulation, right? Like it allowed flexibility for creators, but then came down hard on people who were misusing emails, right? you know, in the can spam act, you had to like, you know, there's obviously the good people, right, which you don't have to worry about the gray area, and then the bad guys, right? So the bad guys, you always need tools, right? Like deep fake detection tools like ours, anybody else, right? Like to detect them because they're sitting in West Africa and Eastern Europe and Russia, and they don't care about regulation. So none of this regulation will matter. You have to catch them with tools. There's the gray area, which is like in the case of the operative who created the Biden robocall. Like, hey, I don't know what the law is, but I'm going to start experimenting, right? And that's where you have to have clear rules, right? Like in can spam, it was, you know, you have to allow people to unsubscribe if they don't like your email, right? Like you have to provide all kinds of ways in which, you know, you very clearly say this is a marketing message, and so on and so forth. So you have to have people who adhere to those rules. And if they don't, Then, you know, they're going to be imposed heavy fines, right? And so you need regulation for this gray area where people don't know what is fair use versus, you know, a parody and things like that. Can Spam Act is a great example. I deal a lot with banks. When online, when credit cards became quite a thing, there was a lot of fraud, credit card fraud. In fact, credit card fraud used to be percentage points of the amount of transactions. Now, now it is measured at one hundredth of a percentage point. So it's become really small. And the big change there was what was known as KYC laws or know your customer. That is when you're interacting with them, you as a bank or you as an organization have to have some basic tools in place that allow you to know your customer. Similarly, in the email, you as an email provider have to have some basic email spam filters, and these are the categories of things that you need to do to protect your folks. So I think this regulation is helpful, but I think regulation like the CAN-SPAM Act or KYC is the right kind of regulation. trying to overreach means you know i'm not a big fan of over regulating something because then you avoid the creativity right like it's awesome that we're able to create movies in a second it's awesome that val kilmer gets his voice back disenfranchised politicians are able to speak You know, Fernando Alonso, I saw Fernando Alonso talking to people in all different kinds of languages because he's used a voice clone to be able to do that. So there's a lot of great examples and you want that human creativity at its best. But for people who are using this in gray areas, you need to come up with very clear guardrails. And, you know, if they don't follow those guardrails, go after them in a big way and then use tools to catch the bad guys.
Ian Krietzberg:
Exactly, yeah, and I guess a lot of that, right, AI is often described as a dual-use technology, a double-edged sword, and so it's the kind of constant and sometimes moral philosophical question of, are those use cases that you're describing worth whatever risk that they might also incur? And that kind of leads me right to my last point, which is, so you guys are enterprise solutions, right? And in a lot of ways, I think enterprise is the clear victim or target of these types of attacks. It's an obvious one, right? Go after the banks, go after Credit Karma, you know, this is where people's data are. we're also seeing a different kind of attack as well that's personalized. I feel like at this point, 2024, close to the end of the year, crazily enough, most people probably know someone who knows someone or knows someone themselves who got a phone call from a relative asking for a money wire, right? Or who got an email and clicked on the link So it's impacting people. And so I'm wondering, from a cybersecurity perspective, what can be done? What can people, what should grandmothers be doing, for instance? What should I be telling my grandma to make sure that this doesn't happen?
Vijay Balasubramanian:
Yeah, I think the key is, you know, we have to find ways to disseminate this kind of technology across these platforms. So communication providers, carriers, and things like that, have to find a way to tell your grandmom that they're on a deepfake call, right? Because otherwise, there's no way she's going to figure that out. Humans can detect deepfakes, audio deepfakes with 38% accuracy, right? So two out of three times you're getting it wrong, right? And so your grandma has no chance, right? And so you need technologies like this within the carrier. You need technologies like this with other platforms to social media, right? Like where you see a lot of this content. You need the clear ability to say that this was AI generated. Maybe it was for a parody, but this was clearly AI generated so someone doesn't think this is the real thing. This is where we've started working. We work with this company called True Media. And, you know, they're doing all of this cool stuff, helping media houses determine what is real and what is fake. And 46% of the videos that we get from them are deep faked. Right. And so the fact is that, you know, it's crazy. At one point in time, right, like when they were telling us when media houses used to get like nine times out of 10, the video would be a real video, right? Like it is the only the odd one that would be doctored and all kinds of things. Now the fact that every other video coming from places like Israel-Hamas war and other conflicts is deep faked is crazy. And so being able to have platforms have the ability to detect deep fakes and make it very clear to the consumer. Being able to have carriers tell consumers, hey, you know what, this is a deepfake call that you're on. That's important. For these tools to be able to say, oh, the person that's using me is misusing me. I think those are super important to actually protect the end consumer. And again, this is where I think regulation can help. Because the end consumer in a lot of these situations, unfortunately, the end consumer, because A lot of these organizations aren't necessarily looking out for the end consumer. You need to be able to protect that end consumer and say, hey, if you are providing a particular service, you need to. Either that or carriers and platforms start charging for these features. And that could be another way. And I've seen that work as well. For my own dad and mom, I am totally fine buying an additional package on the carrier that says, hey, they will not get a deepfake call. I will buy it for them. And so I think it's a combination of either the carriers and the platforms, the social media platforms, the news media platforms do this, or you need some kind of regulation to help with this.
Ian Krietzberg:
Absolutely. Well, Vijay, we're going to leave it there. As always, fascinating conversation. I really appreciate your time. Thanks so much for coming on.
Vijay Balasubramanian:
Thank you so much, Ian. This was such a blast.
Creators and Guests
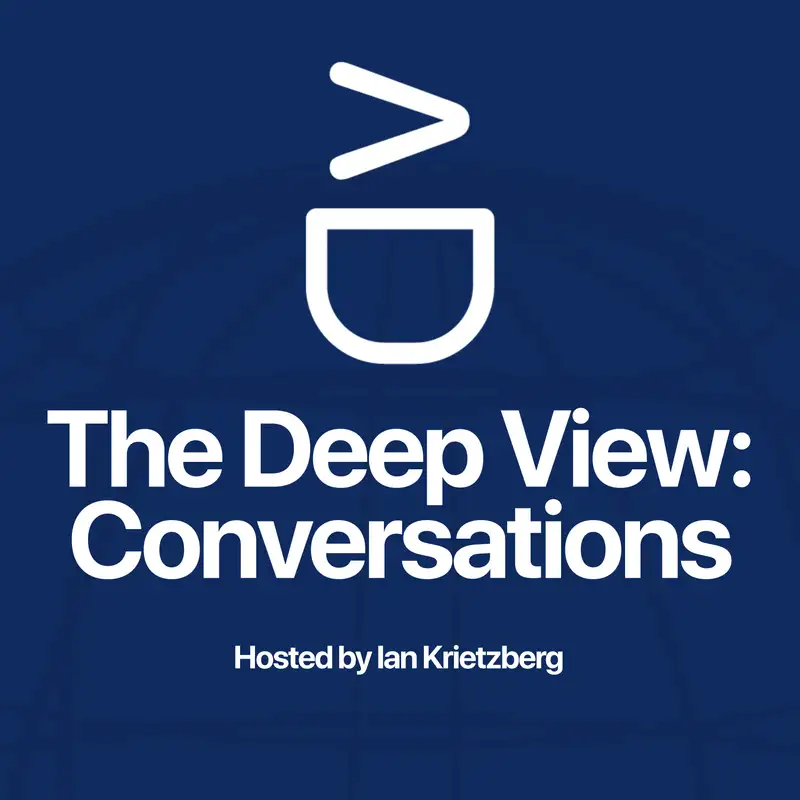