#20: The origins of artificial intelligence - Aaron Andalman
Ian Krietzberg:
I think I like confounding myself. You know, the questions that don't really have good answers. You know, here I'm talking about neuroscience, psychology, cognitive science. Areas that we've learned enough in to know how little we actually know. But in the context of artificial intelligence, these confounding areas of study are more than vitally important in a way that kind of goes in a bunch of different directions. And part of it is that artificial intelligence, as we know it, the foundation of that field, not just inspired by neuroscience, and as we talk about the race to artificial general intelligence, the kind of hypothetical goal that's being pursued by actors, including OpenAI and Anthropic, The nuances and the depth of and lack of understanding about these neurological systems that we're trying to artificially replicate, they become a lot more important. And that brings us back to this kind of confounding nature of these questions. And we're going to get into that today. My guest is Dr. Aaron Andelman. Now, Aaron is the chief science officer and co-founder of Cognitive AI, and he spent quite some time studying biological neural systems, specifically, as we get into, in songbirds. It is as confounding as it is fascinating. I am, as always, your host, Ian Kreitzberg, and this is The Deep View Conversations. Aaron, thanks so much for joining me today.
Aaron Andalman:
It's a pleasure to be here. Thanks for inviting me on.
Ian Krietzberg:
Absolutely. So there's a lot to talk about. I feel like I say that all the time. And I'm very excited to jump in to all of it. And there's all these different kind of things and to try and pick your brain from the neuroscience perspective. And I guess the place to start, or the place that I'm just going to choose to jump in, is the kind of biological nature of a lot of what we're dealing with. And so, you know, as a field, machine learning kind of came about as a means of helping people attempt to better understand human cognition. But I know, since we've talked about it, that the roots are kind of in biology. And I know it has something to do with giant squids. So if you want to kind of start there and talk to me about this kind of very interesting, the natural root of this artificial pursuit.
Aaron Andalman:
Yeah, let's see. That's a broad question. But I think there's a couple. There's many ways in which biology has informed our understanding of intelligence. I think you could go all the way back to Pavlov's dogs and understanding their associative learning or operant conditioning, where you sort of put an animal in a box and let it figure out how to escape. Those experiments with animals led scientists to have a framework for learning, which eventually inspired, I'd say, in the 70s, this whole field of reinforcement learning, which tried to take those observations that we've seen in animals and make a computational model of those. So there's the animal research era, and then there's the reinforcement learning era, where we try to model that. And then there's modern reinforcement learning, where we've combined that with neural networks, and something called deep reinforcement learning. And lots of exciting things are happening there. That's sort of the reinforcement learning history. And so the biology is really in the understanding animal learning. On the neuroscience side, what's powered the modern AI moment, I'd say, is this technology called artificial neural networks, which got sort of renamed deep learning in 2012, around the 2012 time, as I'm sure you know. But that neuroscience, our understanding of the brain really isn't that old. We kind of came to understand that the brain was made up of these cells called neurons just 100 years ago, a little more, but in the early 1900s. And then we had no idea how they worked. we started to learn that maybe they're electrical, and there's somehow electricity's involved. And I just think it's so fascinating that someone was curious enough to want to dive into that. So there's a bunch of fortuitous things, which is the giant squid axon, the irreverent thing, which is someone figured out that these squids I can't remember his name, but it wasn't Hodgkin and Huxley. It was a previous scientist, figured out that these squids had these giant axons that were big enough that we could work with them with the technologies we had in the early 1900s. The microscopes weren't that good back then, and the tools we had for measuring electrical signals weren't that good because the transistor hadn't been invented. So we were kind of working in this old, with this less advanced equipment. And so there was this coincidence that this one animal had these giant axons, which is the part of the neuron that sends these electrical pulses from one neuron to the next. And so two very famous scientists who eventually got the Nobel Prize, Hodgkin and Huxley, started just had this curiosity and this scientific drive to want to understand how the brain functioned. And they went on to figure out how neurons fire these action potentials and how they conduct that signal down the axon to the next neuron so that when someone touches your toe, that signal can come up to your brain and you can feel it. So I think it's fascinating that sort of the origins of those basic ideas, and it's not just those scientists, it's many other scientists, led to this early understanding of the brain being a neural network, and that the brain almost served as this existence proof of like, oh, this concept of a neural network, it seems to power animal intelligence. Maybe if we could reproduce that in a computer or understand how that works, we'd be able to produce something intelligent. And to see that pan out over 100 years, thanks to lots of basic research and lots of different people curious about different components of the world, giving rise to this moment where we're like, hey, maybe we have finally, I wouldn't say we've cracked it, but we've started to make some real progress. in understanding intelligence and producing artificial intelligence that feels remarkable. Started to make progress.
Ian Krietzberg:
Everything you said there is stuff that I fully intend on exploring to a much deeper level, you know, reinforcement learning and artificial neural networks and artificial intelligence. itself and curiosity and research, these are all very tied together and very interesting and not talked about enough. But before we get into all of it, we're talking about intelligence, we're talking about the nature of intelligence, whether or not we can replicate it or create something comparable artificially. I want to start there, I guess, which is, I mean, to you, right, you studied the brain and people. What is intelligence? What's the thing that humans have that marks them as intelligent?
Aaron Andalman:
First of all, I don't think only humans are intelligent.
Ian Krietzberg:
Or other animal species. Yeah, yeah.
Aaron Andalman:
There's many different kinds of intelligence. And I think even in humans, we know that there are different learning systems in the brain that give rise to different types of our intelligence. So we have episodic memory, which is sort of powered, we think, by the hippocampus, where we can sort of experience something, store that away, and recall that later. And it has an ability to sort of capture salient events. So we have these long lives, but there's certain moments that get captured. And there's a whole system for doing that, and sort of storing what our brain believes is relevant. We also have sort of semantic learning, where we're just like, oh, 2 plus 2 equals 4. And that powers a lot of different kinds of intelligence, our ability to do math and our ability to reason. And then we have this maybe less appreciated form of intelligence. It has different names, but I'm going to call it probabilistic learning for now, which is rooted in these ancient systems, the basal ganglia, which is sort of the cortex and sort of the outer part of the brain. The basal ganglia is this deeper, older system that is used for motor skill. It's used for many things. But one thing would be learning a new motor skill, which almost happens subconsciously. A lot of the learning that happens in this brain structure, you're not necessarily conscious that you're learning. They've done these amazing tasks. There's one famous assay called the weather prediction task, where The essence of it is that, you know, you're asked some question and the answer to that question is unclear, but there's patterns that if you keep, they keep asking you these questions and there's actually patterns in them. So like, if you see a red square, you're supposed to say it's going to rain more often than not. And so people play this game, they see these cars, they have to guess. And if you ask them at the end, did you learn anything? They're like, no, that was a total disaster. I have no idea what was happening. I didn't pick up on any patterns. But if you look at their answers, they actually start getting it right more often than not. So they're actually learning subconsciously. They're finding patterns in data, but they're not consciously aware of that. And that system is sort of like, that actually, you know, we use this term like go with your gut. I feel like that's the system that when you say tap into that, it's like I tap into that subconscious understanding where things maybe aren't black and white, but it's probabilistic. There's a whole part of your brain that's sort of figuring out these sort of probabilistic. When is something more likely? If Aaron's tapping his nails on the desk, it probably indicates he's in a bad mood. Maybe it's not a good time to ask him a question. We pick up on these sort of cues, and we learn those things. So going back to your question, what is intelligence? I think there's many kinds of intelligence. And different animals have other unique kinds of intelligence. So the way I see it, I'm curious about all these different kinds. How does each of these work and what role do they play in the ability of humans collectively to do remarkable things? And I think what we'll learn over time is, you know, right now we're calling these neural nets, you know, close to artificial general intelligence. I think they're still a ways off. And I think what we're going to figure out is, oh, there's different kinds of intelligence and to really create something that feels you know, artificial AGI, we're going to need to incorporate these different components and figure out how they work together, which is something we really don't understand yet.
Ian Krietzberg:
We'll just kind of jump right into that on this AGI point, right, that people are chasing, developers are chasing, as you said, that they say that with the artificial neural nuts we have now, some people say we're there. But when we're talking about these different types of intelligence and the things that give rise to it, right? The brain is a neural network. What about the brain's neural network is different than the artificial neural networks that make up, you know, what we perceive of as artificial intelligence today? How are they similar, I guess, and different?
Aaron Andalman:
That's a great question. Today's artificial neural networks are very different in a lot of ways, but at some core level, they're a very simplified version of a real neural network. You know, the brain is a network of neurons, and these neurons are connected in certain patterns, and they influence each other's activity. So, you know, a photon hits your eye, there's a retina at the back, there's a neuron at the back of your eye that happens to be sensitive to light, so it spikes. Actually, the neurons in your back of your eye don't spike, they're sort of a different kind of neuron. But, you know, they activate, and then that leads to it releasing neurotransmitter on some downstream neuron, and then that activates. So the artificial neural networks work like that in the sense that you give them some input, that would be the photon hitting the eye, and then the neurons activate according to that input, and then they connect to neurons downstream from that and downstream from that. And when we learn the connections, when we learn that the theory, you know, The theory of how learning works in the brain is the connections between neurons are plastic. They change. So as you learn something, one neuron might start to influence a different neuron more or less. So the parallels are, hey, it's like a connected network of tiny units, and the units influence each other's activities. the connection between those units is adjusted, and that's what learning is. So those are the parallels, right? Okay, it's like they're both networks that adjust their connectivity in order to learn things. But the biology side is much more complicated, right? Because these connections are chemical. And so when one neuron connects to another neuron, it doesn't just say, you're activated by 0.5, right? Instead, the way they talk is this neuron releases a neurotransmitter onto the next neuron. And that can have many different types. You can have glutamate release. You can have dopamine release. You can have acetylcholine release. So there's these different kinds of connections. And that sort of diversity of connection types isn't really in the artificial neural networks that we have today. So that's one big difference. I think understanding how that richness of connection types is important for intelligence might one day allow us to make more intelligent or more efficient artificial neural networks. Another huge difference is the way the connections learn or are updated. So in artificial neural networks, Jeff Hinton and others developed this algorithm called backpropagation, which is the way, which is a major breakthrough because it allowed us to understand how can we adjust the weights in these artificial neural networks in order to get them to do what we want. And that whole system is definitively not how the brain does it. So like, yes, they're artificial neural networks, but the way we update the weights is completely different. In our artificial systems, we use something called back propagation. In biology, it's more complicated and we're discovering there's different learning rules. So different neurons use different kinds of rules to update their weights. If I was still a researcher, one of my interests would be, what are all the different ways that the brain does that? I think we've identified some, but there are many more. And the question is, well, what is that diversity of learning rules? do for us from an intelligence perspective? And is there parts of that that we need to imitate in order to make, you know, more intelligent artificial systems?
Ian Krietzberg:
Yeah, you were mentioning how, you know, as we might understand more about the diversity of those connections, that might enable us to build more advanced systems. And I wonder at the stage that we're at now, Which, as we said, people are saying AGI is here or will be here soon, right? AGI remains hypothetical. How much we're limited by the fact that, you know, as artificial neural networks go, they're trying to mimic cognition. And we don't know a lot about the brain itself. How much of a limiting factor is our just lack of knowledge about how the brain does what the brain does?
Aaron Andalman:
Yeah, we don't know how limiting it is, right? There's some people who believe we've got everything we need. If we just scale these neural networks and apply reinforcement learning, which is sort of, in my opinion, the piece that people think is going to take these large language models all the way to AGI, some people think that's all that we need. We will get there. And that might be true. I think it will take more than a year. We could get into what I think the challenges will be of applying RL to really take these foundation models to what we're going to call AGI. And AGI is a tough term, and we can get into what the definition of that is. And then, like we said, we don't know. It's possible those two tools will get us there. But it's possible that we need an additional breakthrough. We need to understand some new learning rule or some way that'll just take that reinforcement learning and make it make it more effective or more efficient? And it's also how do these different forms of intelligence need to combine to create the diversity of curiosity? The fact that we've made this much progress in terms of how much knowledge we have as a species, isn't just a function of our intelligence. It's a function of how we work together and our ability to sort of like share knowledge and our ability to have diverse interests that don't completely overlap such that we can work together to discover new things. And so I don't think we have concrete solutions to how to make AIs that do all of that.
Ian Krietzberg:
You mentioned efficiency. And that's a really interesting point when we're talking about intelligence, because increasingly, you know, the idea of the way fundamentally these systems today are trying to mimic intelligence is through brute forcing it. And that's the kind of fundamental component of scaling these systems up. If we can just throw more at it, more compute, more chips, more data, we'll get there. But it's like it in its nature, it's unlike human intelligence, because human intelligence is not scaled to that size. Like we are, it's a vast network of neurons. But we weren't trained on, you know, we haven't consumed and memorized and read every piece of writing and every piece of content that has ever been created in human history. These things have, and these things are still brittle. These things are still unreliable and unpredictable ways. And it's interesting kind of looking at it from that perspective of, to me, something that I've been saying for a little while now is that, you know, my big marker of, of AGI, which, you know, we can get into it. I don't like the term at all. I don't even like the term AI, um, is something that would be rooted in a very small amount of training data, which would be a little bit more akin to the organic cognition that we're trying to replicate. And like you said, in terms of finding new breakthroughs, I don't see a path to something like that right now.
Aaron Andalman:
Yeah. I mean, I do think we're finding ways of getting more out of less data. In fact, I think kind of foundation models provide all this priors that lets you like fine tune with less data. So. I mean, multiple things there, but from an efficiency perspective, I think we'll certainly look back in 10 years and be like, wow, the systems we were building were. were wildly inefficient. And we've discovered new ways to learn faster and find statistical patterns with less data. So I think that will be true. But on the idea of, I think there was another component to your question, which is related to that diversity of intelligence. We have a kind of intelligence that doesn't involve reading every book on earth. These foundation models, in a way, I think one reason there's all this buzz about this is going to be artificial intelligence or even super intelligence is because they work in a very different way. And they have read, they do have this remarkable wealth of knowledge. I mean, if you play with them for any amount of time, you realize that there's actually these wild gaps. Yes, in certain ways, they seem remarkable and super intelligent. In other ways, you're like, oh my gosh, it has no clue what it doesn't know or does know. So I think it's not really about, is it super intelligent or generally intelligent? It's about understanding, well, what is it really good at? And how can we utilize that? I don't think it's about to be a super intelligence that dominates humans and then we have an existential question of what is our purpose? That seems to me some distance off, but it is going to surpass us. Computers surpassed us at long-term memory a long time ago. They can hold on to things forever. And they surpassed us at processing speed a long time ago. They can multiply way faster than we can. And over the next few years, these AIs are gonna surpass us at other tasks. I mean, it's already happening, you know? And that's amazing, okay? But it's not AGI, or it's not what I call AGI, or it sounds like it's not what you call AGI. You kind of were like, it seems like AGI is like ability to like go deep on one topic with not too much data and really like extrapolate out and discover something new. I mean, that's one definition, but, So my view is, hey, these things are going to get really good at certain tasks, especially where RL is a better fit. Where RL is not a good fit, I'm not sure how we're going to get really to that next level.
Ian Krietzberg:
The idea of what is it good at is an idea I like. And as we jump deeper into the RL question and the AGI question itself, it raises an interesting question to me that I have been asking since I first started doing this however many years ago, which is why pursue AGI at all? It's a buzzword. I don't even want to get into the motivations around the ways and reasons in which it's being pushed, but they definitely seem political in nature or related to raising funding and other natures. And AGI, the idea of this general intelligence, has been something this field has been pursuing for a very long time. This is not recent history. That's been the kind of end goal for so long. But when we're dealing with what we have today, and when you're talking about just applying it in situations where it's good, where it's useful, where it actually helps us, It's the perspective of looking at it as a tool versus kind of creating this new species, which is how it's talked about from some of these labs. And I wonder the value of the pursuit. If we just diverted all our efforts to improving models for cancer diagnosis instead of trying to build a general intelligence, so many resources are going to scaling LLMs up into this hypothetical thing, when if we looked at it from the targeted tool perspective, there's probably a lot of enhancement that we can do. And so, you know, for you looking at AGI, is it a worthwhile pursuit?
Aaron Andalman:
You know, it's interesting. I don't really think I frame it that way of like you're either trying to do a niche thing with the current foundation models or you're trying to push the foundation models. I think I think there's room for both things to be happening. And you mentioned the pursuit of AGI as an old idea. I think that's grounded in curiosity about how humans work. I think that's how I got interested in neuroscience was sort of at a mentor at Xerox PARC, who was a cognitive scientist, just got me thinking about, Actually, he got me thinking about just visual parsing, like how we parse the world visually, which led me to reading some Steven Pinker books. And then I was like, wow, yeah, intelligence is this really fascinating topic. I went to get a PhD because of that. And I was interested in intelligence. I think I wrote my entrance essay or whatever on diversity and intelligence and wanting to understand that. So I think the interest in AGI is just interested in like, well, how do our minds work? How are we intelligent? So I don't think that will go away. And I think that pursuit is valuable. These days, there's, like you said, there's a lot of hype around we're already at AGI. And then there's that result in a lot of discussion of like, well, what does that mean? And I do think that if we did eventually create something that was super intelligent to us and along every dimension, that would be an existential like, what is our purpose, you know? I don't know if you ever saw that Broadway show Avenue Q where the characters are like, what is my purpose? I feel like if that happened, we'd be like, what is my purpose? But going back to like, why pursue this and what are we investing our time in, you know? I think foundation models are important. If we can build something that has this richer world knowledge on which we can build many different niche applications or sort of fine tune them for a particular use case, I'm an optimist. I think that's going to create a better world. If it happens too quickly, then like shifts, you know, I don't want to get into the politics and the shifts and what it will mean societally, but overall, I'm positive. Especially around like uses in education. And so your question, I don't remember what the exact question was, but I would say generally, I don't see it as like, should we invest in the individual applications or in AGI? And I don't even think creating the foundation models is purely in the pursuit of superintelligence. I think that's all spin around funding and things like that.
Ian Krietzberg:
Going back to the idea of scale, we're jumping all around today. The idea that we can use reinforcement learning to scale large language models into something better. And we've seen that, right? We've seen it play out. The recent kind of push in reasoning models, chain of thought reasoning, it's applying reinforcement learning to get these models to apply the kind of chain of thought. approach for more robust answers, an output which increases inference time compute. So we've seen, if you look at the O series in R1, and now everybody's doing it, the value that has at this early stage. But let's just take a step back first. Um, reinforcement learning, we, we touched on it a little bit, but if you just kind of want to dive into what that is and, and, and interestingly to me, um, because reinforcement learning happens in organic beings as well, right. How RL is different in these kinds of artificial environments compared to, uh, you know, like a Pavlovian thing. First of all, what is RL?
Aaron Andalman:
I think it's easiest to think about RL in terms of what it's good for. You want to use RL when you have a situation where you don't know the correct thing to do. So, like, if I knew, like, in this situation, turn left. In this situation, turn right. In this situation, drive straight. If I knew all the answers, then I could use supervised learning, because that's what it means. So, supervised in the sense that I'm telling you the right answer in all these situations. If I'm in a situation where I don't know the right answer, but I know the goal, but let's say the goal is to win the game, or the goal is to get the most dollars, or the goal is to get the most points, then RL is RL is designed for that situation. And the way it works is actually very intuitive. The idea is like, hey, this is your goal. Use trial and error to figure out how to maximize that goal. That is what reinforcement learning is. And so to make it concrete, you can think about situations where you would need that. So a super famous one is the game of Go, or any game. If you want to make a computer, play a game better than any human has ever played it. You can't use supervised learning because you would supervise it with human answers. And then it would just be copying what humans do. And it might be so good at that that no human could beat it because it would be like error-free, mistake-free, but it wouldn't really invent anything new. It would just be copying humans so well that, and maybe merging different humans' abilities, but it wouldn't be discovering new ways of playing a game of Go. With RL, you're just saying, your goal is to win. Try everything, you know? And that may sound simple, like, yeah, trial and error, but it's actually really hard in practice for lots of reasons. Like maybe the search space of things to do is infinite, you know? Because you could bet any amount from zero to a million or any penny amount in between, or maybe the, Maybe the number of moves is so large, like in Go. Or maybe the rewards are so sparse, like it's very hard to apply. Or maybe it's so expensive to do trial and error that you would lose all your money to use the system. So there's lots of reasons why it sounds simple, learn by trial and error. But there's lots of reasons why it's really hard and it's taking a very long time to apply in many different cases. And going back to your, so that's what RL is. And then, Really, in another example, to get to the component or question that's related to the animals, as a student at MIT, as a graduate student, I got my thesis. I did my research studying how songbirds, a small songbird called the zebra finch, learns its song. And it turns out they don't. They don't learn. They're not genetically pre-programmed to produce the song. They actually learn to sing the song of typically their dad, but any tutor. So only the males sing. They sing to attract a mate. And they hear a song when they're young, typically their dad's song. And then they learn to copy that as a kid. And they do that through trial and error. And over the last, and what we've learned is some of, I think the reason there's a lot of excitement about this neuroscience reinforcement learning nexus is what we've learned is some of the algorithms that these computational folks came up with in the 70s, we see signals related to that in the brain. So one key part of reinforcement learning is this idea of reward prediction error. I took an action, I thought I was going to get a reward, and I didn't. Damn it. That's like a really important signal. You're going to use that to update your behavior. And a famous neuroscientist, Wolfram Schultz, was recording in monkeys and found that exact signal. And so I was like, oh, maybe these algorithms that rely on reward prediction error to do RL, maybe that is actually how the brain works. So there is, I think conceptually, RL is really simple. It's like learning through trial and error. And we know humans do it. And now we have algorithms to do it. And those algorithms weren't really useful until we merged them with neural nets, which was this idea of deep reinforcement learning. And so it turns out that these reinforcement learning algorithms combined with neural nets It's a really powerful approach. And I think it is very closely related to how our brains learn things. But there are differences. I don't even know if I have all the answers for you on how exactly those are different or the same. That was a long-winded answer. I hope that was clear.
Ian Krietzberg:
I love the songbird. I love the bit about the songbirds. And it's really interesting, the signals that you're talking about that appear here, and then you study the biological life, and oh, that same signal is there. And that's, I mean, going back to this idea that, you know, at the root of this field is an attempt to better understand human cognition, animal cognition, organic biological intelligence. And I think an interesting similarity between these two things, right? We know large language models are referred to as these black boxes. We don't really know what's happening when they do what they do. We know general things about them, kind of similar to the brain. We don't know a lot about the brain. We know more. We're learning stuff. And so I, from that perspective, just of, of a pure, like cognitive science, neuroscience perspective, do you expect, I guess, that as we learn, it'll kind of be like the, the two components helping each other learn more about each of these two components, like learning more about how a deep learning system works might help us learn more about the brain and learning more about the brain might help us learn more about how a deep learning system works. If they. if they happen to kind of accidentally or on purpose mirror each other in action?
Aaron Andalman:
There is one. I am very excited about the mutual learnings that these two fields can offer each other. In fact, I think there's a new institute at Harvard that opened up since I left academia called the Kempner Institute that's just focused on this exact idea of, hey, there's AI can help us understand the brain, and the brain can help us make better AI. And I go into specific examples of both directions, how I think that's already happened, and how I think that will happen. From the songbird. Specifically, I think we've gained insight into how the bird does reinforcement learning that might inform future RL algorithms or even already have. So let's take you might know there's some famous neuroscience results. One is this idea of replay, where you look at neural activity in an awake animal, and then the animal sleeps, and it plays back that activity. That replay idea, was core to some of the first deep reinforcement learning algorithms that got published in like 2014, that actually were the first papers from DeepMind that then got them acquired by Google. So they had this idea in that first paper of replay. And the idea was like, you experience something, And you're like, oh, I didn't get as much reward as I thought I would. And now you need to learn from that. But you can't necessarily learn from that right away. Turns out you need to replay that experience over and over again in order to update all the neural weights effectively. And so the original deep reinforcement learning paper was like, ah, I need to replay these experiences. And that's exactly what we see in the brain. The brain replays neural activity at night. or even at rest. And the interesting thing about that replay is they can look at the speed difference. Like in a rat, it doesn't replay the activity at the same speed of real life. It replays it at about 7x the speed. So we're like, oh, like, fun quote is, we dream at like 7x the speed of real life. Or there's other. You know, there's also this idea of prioritized replay, which was another future paper out of DeepMind, where they said, what was the idea? That basically it was like, we don't want to replay all experiences equally. There's some experiences that are profound, and we should replay them more often and learn from them more. And there's others that are less important. And we see the same thing in animal replay, where it's like, that the events that the rat replays in its brain at night aren't just random events. Like, there'll be the moment that it, like, found something to eat, or that, you know, it replays the important events. So those are ways in which neuroscience inspired reinforcement learning. Or even another great example, not to be long-winded, but One key breakthrough around 2012 from Jeff Hinton was this idea of dropout, which is a way of getting neural nets to generalize better. It's like, I could train this neural net, but it just memorizes everything. But I don't want it to over-memorize. I want it to extract the right information so that it applies to other examples well. That's the idea of generalizing. he invented this this way of doing that called dropout and it was really inspired by neuroscience. So what we know is like when two neurons in the brain talk to each other They don't reliably connect. So if this neuron spikes, it doesn't always release neurotransmitter on its downstream partner. It's probabilistic. Sometimes it'll do it, sometimes it won't. It's this weird noise. It's like, why would you want that? And so Hinton said, well, what if I make my connections in these artificial neural networks drop out sometimes? Like sometimes they'll be connected and sometimes they won't. And that was actually super effective at making the neural network work better. Now, I don't know if his original idea came from the neuroscience, but when he presented this idea, he linked these two concepts, the neuroscience binding with this idea of dropout. So those are very concrete examples of neuroscience inspiring artificial intelligence. And I'm equally excited about the reverse. or maybe more excited, I think, like you hinted at, something I think that's really profound. We don't understand the brain because it's 200 billion neurons and trillions of connections. And we find these little artifacts like, oh, this neuron fires when you see a dog. But we don't understand the meaning of that. And we're having a hard time getting to the meaning of that. And at the same time, as you pointed out, we also don't really understand these deep, these large language models. There's like, you know, they have 2 billion neurons. They don't really have 2 billion neurons, but they have, they have 2, they have, they have, maybe some of them have a trillion parameters, which is basically a connection. So they have a trillion connections. And we're like, we kind of understand them, but you can never fully understand them, which is why there's this problem of alignment. Like, how am I going to align this thing that I can never fully understand? And I think that gives us a clue. The same way we're never gonna understand an artificial neural network that has a trillion parameters. We're never gonna literally understand every tiny thing in the brain. We need this like conceptual frameworks. of like, well, what are the learning rules that this neural network uses in order to achieve intelligence? And what are the connection patterns? And so I think as we think about ways to understand these artificial neural networks, those ways of thinking will apply equally well to real neural networks. We're like, oh, I came up with this clever way of understanding how an artificial neural network works. or I understand the learning rule, I want to understand artificial, real neural networks in the same way. I think there'll be a... I think there'll be important parallels there that are only just starting to happen. I could go on, but I think hopefully that's a sufficient answer.
Ian Krietzberg:
Yeah, I love all this stuff. I love the neuroscience. It's always a confounding thing to me. We're dealing with stuff that we know enough about that we know how little we know. Correct. Which is just a funny place to be. Um, and then this idea that you're laying out of, it might not be possible for us to, to understand in depth, everything in the brain or everything in a neural network is interesting. And the, the, the concept of, you know, if we can understand the rules of the road, that's enough of an achievement. And I think about, you know, like beyond the curiosity of we want to chase understanding, the value of us understanding in more depth how the brain works medically, there's a lot of reasons for doing that, right? Like the more we can understand how the brain works, the more we can maybe understand, mitigate, treat neurocognitive disorders, which would be a good thing. But it's really, it's really interesting to try and to dive into this thing that is, it, you know, seems in many ways so far beyond us and to try and, like, brain mapping is this one thing, right? And I think researchers at MIT were able to map the brain of a fly, like a flywire brain. Connectome. Yeah, and it was really hard. And it took a really long time. And it's such a small, simple thing compared to our brain. And it's such a fascinating thing. We're chasing understanding. We talked earlier about curiosity and the kind of randomness of chasing these passions and human curiosity. You know, even down to you and chasing the songbirds and cognition in these animals and the researchers who chase the giant squid axons, right? And, and I wonder as we get to this point where, you know, curiosity is leading us to advancements in artificiality. If that's not a word, I just made it up. And I, you know, we kind of touched on implications for society, right? Like if we were to develop a super intelligence with that, what would that mean? And yeah, there's there's a lot of, There's a lot of what would that mean for superintelligence, but dealing with the more real thing of we have neural networks, we have LLMs, they're proliferating, we're chasing advancements in them. Those advancements are powered by curiosity. Could a more powerful system then start blunting our curiosity and blunting our passion to chase knowledge if, you know, kids start being raised with a system that just has answers to questions so they don't need to pursue stuff in the way that things were pursued? And I wonder what you think about that kind of just weird juxtaposition of creating or attempting to create a thing that can just do everything and what that might mean for innovation?
Aaron Andalman:
Well, first of all, I think a machine that will just do anything, everything is more than five years away and probably farther than that. That's my prediction. I'm with you there. Go out on a limb there. I think Jeff Hinton, I just watched his Nobel interview and he had it at like five to 15 or five to 20 and I was like, yeah, I'll go with that. I think maybe farther, but I don't think it's this year. Let's put it that way. And then also going back to your comment about the connectome. So Sebastian Siong was one of the important people on that fly connectome paper. He was actually on my thesis committee. I think that work is amazing. And I think it will fundamentally help us understand the brain better. Kind of like the genome, but for neuroscience. I think it's... I think some people question it, but I'm a big believer in that work. Just check that box. In terms of AI-based system being so good that they blunt, I guess your question was like human curiosity. I'm broadly optimistic. I think AI can be an amazing teaching tool. I'll give you a real example. So my daughter, She was working on this on this pretty hard math problem. It was what was it exactly? Okay. It was that there's a 9 by 9 grid. Okay. And you have to put the numbers 1 through 81 in this grid. And the question was. What is the minimum number of rows and columns whose product will be divisible by three? So you can put the numbers one through 81 in this grid, and what's the arrangement that'll minimize the number of rows and columns whose product is three? And that's a pretty hard problem. Turns out there's some tricks. It's not that hard. Basically, the question is like, how many rows and columns will have a number that's divisible by three in it? That's all you need to know. And so between 1 and 81, 27 numbers are divisible by 3. So the question is, like, if you have to put these 27 numbers in, how can you compact them so they touch as few products and columns and rows as possible? And I was trying to explain this to my daughter, and I was like, she wasn't quite getting it, you know, because it's hard to visualize. I was trying to explain it, and she was like, I don't know. I could just see that we were missing each other. And I thought to myself, it would be really cool if I can make a little tool to visualize this. Like, I have an image in my brain that's helping me solve the problem, but my daughter doesn't have that image in her brain. And, you know, it would typically take me Let's say I wanted to code that visualization. That might take me four hours, six hours. It could take me a long time. I don't code that much these days. I'd have to go figure out what Python package to use or whatever. But instead, I was like, hey, I don't remember which model I used. Probably chat GPT. I was like, hey, I just wrote a prompt. It was about a paragraph. This is what I want. I want a little interactive thing where I can get a grid and I can click the boxes. And I'm going to put the numbers 1 through 81, and you're going to show me the count. of rows and columns that are divisible by three. I punched it in, got some code, ran it. It almost worked, but like the colors, when I clicked the boxes, nothing changed. And then I was like, hey, it's not working. And it was like, are you on a Mac? And I was like, yes. And then boom, out comes the code. 10 minutes later, I have this tool just for this problem so that my daughter and I could share a mental model of how to solve this problem. And I thought that was And I was like, you know, there's so much potential there. It didn't blunt either of our curiosity. It allowed me to share my understanding with my daughter. at a speed that wasn't possible before these tools existed. So, you know, going back to your question, your question was, you know, are these going to blunt human curiosity? I think there's always a risk that some people will rely too heavily on those tools or disengage from learning. But it's not clear to me that the tool is what's going to do that. Like maybe that student's not interested in this topic, and so they're going to go lean on that tool. Or maybe The same thing can happen today. You can go on some website and buy a paper. I don't see this ultimately as living curiosity. I think there's a diverse range of people. Some people might get sucked into using them poorly, but I think other people are going to benefit hugely. I think the research shows that one-on-one can be extremely effective. And I think this is going to allow us to do more of that as a society.
Ian Krietzberg:
Probably as good as any of a place to leave it off. You know, optimistic, uplifting tool for the society. Yeah. You know, dangerous superintelligence is not here yet and probably won't be for a while if it's coming. Yeah, I, that, that example, the, the, the kind of code you created with the grid, it's a really interesting, uh, use. And I think, and what you're talking about, I think it speaks to this environment that we're starting to find ourselves in, which is for the highly curious. Whether or not they use this tool, the tool is not an inhibitor. it's a means of potentially enabling. I think for less curious people, you might start to have a risk there of over-reliance, which could lead to apathy and atrophy. And I guess the challenge, I couldn't imagine being a teacher right now, but I guess the challenge is to make sure that we cultivate not just the critical thinking, but inquisitiveness and these kind of other things to make sure that there is no atrophy and to make sure there is no apathy. And then that, it comes down to a- Wally, you're bringing Wally to my mind.
Aaron Andalman:
I'm like, oh no, you know, like that's a question. Like in a world, I think we're far from that, but you know, you're saying the productivity tools alone might create an environment where we're overly dependent on them. That certainly hasn't been my experience. And I think there'll be teachers who benefit from it and teachers who struggle. And it will change the way we teach. And it will probably change the way we have to test. There was an example on Hard Fork, which is a podcast I liked last week about someone at Columbia who created a tool to help people ace their coding exams on these for internships. So there will be. You know, little things, but big picture. I think those are solvable problems, separate from the existential question of like, you know, in a world where the AI can do everything, like, what is our purpose? I think that's a harder question.
Ian Krietzberg:
We'll leave that for next time, hopefully. Next time. Hopefully it'll be a while before we even have to think about that. But yeah, how to be curious in the age of AI. Aaron, thank you so much for letting me pick your brain about the brain.
Aaron Andalman:
Thank you. I really enjoyed our conversation. And even though we went in many directions, I thought we hit on a lot of interesting topics and really brought back a bunch of memories about neuroscience. I don't think about neuroscience every day in my job. So kind of fun to go back into the annals of my memory and try to think of some of them.
Ian Krietzberg:
Yeah. Activate the long-term memory. Awesome. Yes.
Creators and Guests
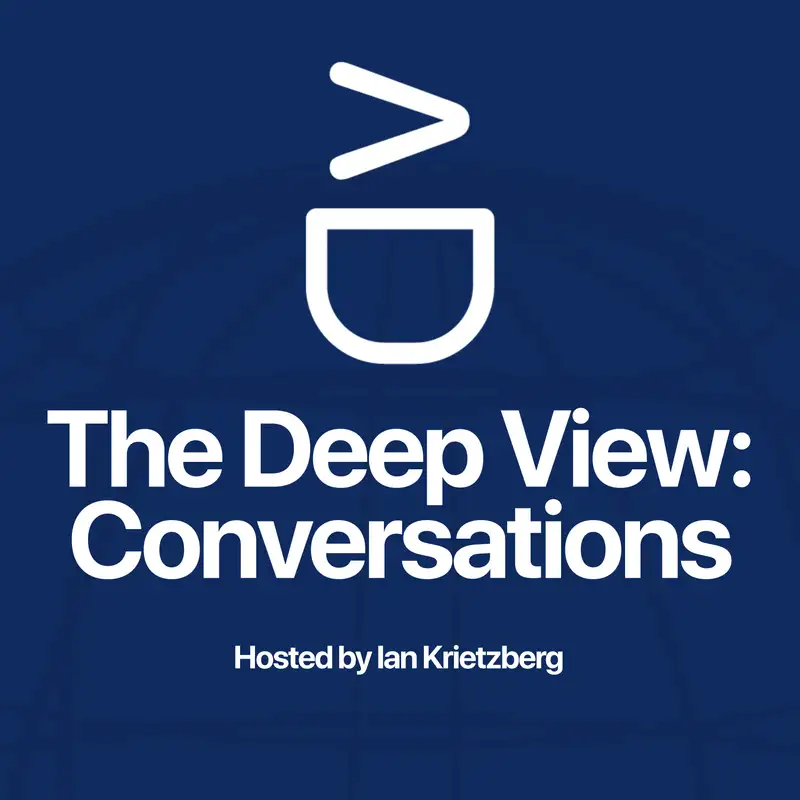