#17: AI, compliance and utopia: Can tech actually make the world better? - Eric Sydell
Ian Krietzberg:
Welcome back to the pod. I am your host, Ian Kreitzberg, and I gotta tell you, I think a lot, like a tremendous amount, about the philosophy, the sociology, and the psychology that surrounds artificial intelligence, both as a field and as a technology. Fortunately for me, and fortunately for you, I hope, my guest, Dr. Eric Seidel, thinks a lot about it too. Now, Eric is the founder and CEO of Vero AI, but he comes from a background in psychology. And so he's bringing this social scientist perspective to the use and integration, implementation of artificial intelligence. And I have to say that this conversation really speaks to the nature of what we're trying to accomplish with the show, which is kind of rooted in this fact that a lot of what we explore here is necessarily confounding. We raise questions that don't have clear answers, or maybe don't have answers at all. We raise issues that are philosophically dense and intense, and we deal in areas that don't have a lot of clarity. But in doing so, well A, that's kind of the only thing we can do at this stage, But in engaging with these things that are difficult to engage in, we... We move closer to a point where we can better inform the trajectory that we are on as a society that is dealing with this technology, as an industry that is disseminating or adopting this technology, and as regulatory bodies that are figuring out the best ways to approach this technology. So this one is super wide ranging and rooted in discussions that are kind of philosophical in their nature, always excites me. And so with that said, this is the Deepview Conversations. Eric, thanks so much for joining me today. Thanks for having me. Great to be here. So I want to start, I know that there's a lot to get into and we're going to get into all of it. Um, before we do, I want to start with Vero itself. Um, and so, you know, very broadly, what's the big pitch here? What, what problem are you trying to solve and why is AI, uh, the tool to help you solve it?
Eric Sydell:
Vero AI is a platform for compliance. And in the compliance world, there are tons of different frameworks, compliance frameworks, things like ISO 27001, and HITRUST, and CMMC, and even Sarbanes-Oxley in finance. There's a lot of security, finance, cybersecurity-related requirements that companies have to comply with. It's hard to do that. These frameworks require a ton of time and detailed evaluation of documentation. It's difficult for companies to do this. It's difficult for audit companies and compliance companies to deliver the services quickly and affordably. AI is perfectly positioned to help with this challenge. We recognized this early on. We created Vero AI as a platform to help companies evaluate all this information and figure out whether and how they comply very quickly, very accurately with the most cutting edge AI techniques and other statistical techniques that we could compile and use. There are plenty of companies in the compliance automation space, but they tend to be very broad platforms. So they're focused a lot on project management types of things and communications, document libraries, and all sorts of things like this. Not necessarily what we're focused on most, which is using AI to help automate the human intellectual labor, if you will, that goes into these compliance determinations. And so we're envisioning what compliance looks like in an AI world where we have access to these cutting-edge large language models and other types of capabilities, and it's dramatic what it can do for that process. I can certainly blather on for days about the benefits of using an approach like ours in compliance and how it goes beyond what people think of as compliance, and also makes it very, very affordable and super cost effective. But I'll save some talking points for later. Sure.
Ian Krietzberg:
I wonder, with stuff like this, anytime I hear about applications of, you know, especially large language models, but whatever we might refer to as AI very broadly, in higher risk domains, right, that I have a lot of questions always. And here we're talking about compliance with I mean, if you're in finance and you're not compliant with FINRA, that is going to be a problem for you legally. And the list goes on for all these other industries. And we know that large language models have reliability problems. And even older statistical methods have certain problems if data is incomplete. And I wonder where that kind of reliability issue factors in here, how you ensure that whatever whatever work the system is automating, how you ensure that it's doing so in a trustworthy or explainable way.
Eric Sydell:
And that is exactly the question that everyone should ask, I think, when they are investigating a tool like ours. That's appropriate and the right question to ask. And so You know, what we've built is a way to process information unstructured information of all types and to help make sense of it and package it in a way that a human can take it to the next step. So what we have not built is a platform that can automatically say. whether you're ISO 27001 compliant or not. We aren't doing that. No one can do that. Anyone who says they're doing that is not being honest. What we can do is process and organize vast quantities of information down to a simple output that a human can take to the next step. That's our purpose and that's our goal right now. I just want to be clear about that. The next point is that when we're doing this, There's nothing black box about our system. So we're not saying for this particular control standard, you're compliant or not compliant. We're saying we think that this is mostly compliant and here's why. So we're using our system to create a list of evidence that the human can evaluate and verify. Or it might say that we think this is not compliant and here's why, but it's going to specify the very specific pieces of the documentation that we reviewed as support and evidence for that determination. So at the end of the day, it's still the human that's using this information to determine compliance. So there can be inaccuracies. There can be things we miss. I can't warrant that that doesn't happen. But I can say that it doesn't happen very often and that the output is sufficiently high enough fidelity that it's very useful for the human and that it's rare that there's actually anything that would be considered a hallucination or that type of thing. And part of the reason is the way we've constrained these systems to work. So it's not just large language models. I mean, that's certainly a component of it. And of course, we're using, you know, RAG implementations of our system, which, you know, for listeners is a more private and secure, you know, way to deploy a large language model. So you're not giving your proprietary data, you know, to the LLM companies themselves. It exists in a secure kind of sandbox. So they're not training on it, you're not sharing confidential stuff with the whole world. But the way RAG systems work is the first step is more of a search. It's not even really AI, it's more of an old school search and there's different ways to match information. And we won't go into that because it would immediately put all of your listeners to sleep, things like cosine similarity and stuff. So that's the first step, and then it goes into the sort of generative aspects to some extent with the output that we print for users to see. But there's different levels of machine learning, and there's also logic built in that we program ourselves. So there's a lot more to it than just the LLM. And at the end of the day, everything is very transparent and visible for the end users. So I think that's how we've tried to deal with this problem. And LLMs get better all the time. Hallucinations are declining all the time. They're being worked on constantly by the big tech companies. Will they ever be fully gone? I don't know. I think I mean, I read your stuff and I know what you think about this and I agree, you know, that it's probably the case that we'll never fully get rid of hallucinations with current LLM architectures. LLMs are just one piece, you know, they're just one piece. And when we think about AI, gosh, there's machine learning and AI, there's so many high-powered capabilities and techniques that have been built. And what we're doing is building on top of them to try to show end-users, business end-users, real reliable results. And I think there's tons that we will continue to iterate on and tons of applications that haven't even been built yet that are out there that startups are working on, that are very exciting and will lead to a lot of additional automation.
Ian Krietzberg:
Yeah, it's the systems that are interesting to me, you know, versus like looking at a model as a component of a wider system and injecting bits of determinism into something that, you know, the promise was that it's non-deterministic, which is also the challenge here, because how are you supposed to deploy something in a high stakes business environment that isn't deterministic and that, you know, as I have written about, right, makes mistakes in these kind of unpredictable ways. Like we, we see a lot of conversation about the kind of the the jagged edge of of LLM adoption or LLM capability, where it might be very, very capable at one thing, and then fail in something that is so trivial in comparison, in the next second in the same model from the same system from the same company. And It's that unpredictability and how it's going to fail, right, that makes it really challenging. But like you were saying, this is why the system approach in the enterprise is kind of the approach in my mind, or for any high stakes place, because if you incorporate older schools, statistical methods, search methods, bits of determinism, older types of machine learning, then you have something where you're kind of, you're using everything for what it was supposed to be used for, right? At the end of the day, large language models are language models. And so they're good for kind of translating the information from one system into something that's readable by a human in the output side.
Eric Sydell:
Yeah, for sure. And, you know, these, the big LLM companies, I guess, naturally focus on these, you know, really easy to understand consumer applications of their technology. So you know, it's pretty fun to talk to chat GPT and ask it questions. And it's pretty fun to create generated images, you know, Mona Lisa, but if she was, you know, her father was Danny DeVito, what would that look like? You know, just like, just, you know, what's fun, right? But then we get into like, okay, well, how do you apply this stuff at scale to business processes? And I think that's a different conversation and you have to get outside of the hype. to really think in detail about how you can use these tools combined with other tools, like you said, to generate business results. And that's the space we're in. And I think it's really exciting and it's fun, but it's less sexy, I guess, than the mainstream hyped applications tend to be. But there's an article from a few months back that Sequoia Capital wrote, some investors at Sequoia Capital wrote talking about service as a software instead of software as a service, and this idea of cognitive architectures, which is building code on top of large language models to solve specific problems. Ethan Mullock on LinkedIn recently had a comment about this too, where he said that this basic LLM technology and these other AI tools that we've built, We could stop iterating and stop developing them right now, and it would still take the business world a long time to catch up with that capability and to start using it in all the ways we can already solve a ton of problems with these tools. that aren't yet being solved. So there's a lot for a company like us to do with the tools as they currently exist and a lot of value to add. I mean, you know, a pre-audit. Let's look at a pre-audit in the compliance space. Like a company could easily pay $30,000 to $150,000 to do a pre-audit on a certain framework for their business. It's a lot of money, right? And it takes a long time, it takes expertise, it takes consultants. We can do that in six minutes of processing time. Upload the documents, click a button, the system thinks about it, and six minutes later you get a report that's basically the same as what you would have paid $150,000 for one of the big consulting shops to do. I mean, it's right there. That's something we've architected and built. And I mean, it's eminently deployable. And what about this? What about, hey, instead of just looking that whole process at one compliance framework, what about we do them all at the same time? It still takes six minutes. And what about now you can do it continuously and not once a year? because it only takes six minutes. You can continuously evaluate and monitor everything. Yeah, that's fantastic, right? And yes, it's not perfect, but it gets you so far down that path that it's massively, massively valuable, and the economies of scale are insane. So this is just one area. We're just doing compliance. Now, I would love for us to go beyond compliance and do everything, because as a proper startup guy, I'm like, you know, ADHD is heck. So there's a million applications of this. But, you know, we're focusing on compliance right now. But I mean, there's so much that this type of stuff can do. So Sequoia, going back to that example, I mean, they talk about this market for this type of thing as being in the multiple trillions of dollars. trillions of dollars. That's a big TAM right there. And the reason they think it is so big is because this represents the automation of human intellectual labor in a grand scale. So they're seeing this potential and this possibility in the future. And I mean, that's, you know, there's an element of hype there, of course. But, you know, we see it as valuable and very exciting.
Ian Krietzberg:
Yeah, I mean, it's very interesting. I, you know, like to circle back to what you said about what Ethan said, and I saw that post as well. And he's absolutely right. And it's kind of the, I think in some ways the industry has challenged itself in allowing that to happen. You know, what you're talking about is kind of the transition from a toy to a tool. And the toy is fun to play with, right? Mona Lisa, she was, her dad was Danny DeVito. But a tool has much more specific requirements to it. A tool has to be used, if it's being used for business decisions, there has to be an acceptable error rate. And how do we quantify that? And so the conditions for deployment are different. And because of that, in many ways, because of the hype and because of the playability and the gamification of these tools, I think the industry has challenged itself in selling a very specific tool, which is hard to overcome, right? And that's why if advancement stops, and I think on the other hand, too, I think advancement itself is hindering adoption because it's moving so quickly. I can't even count the amount of new models that have come out this year alone. You know, we're recording this towards the end of March, mid-March, whatever. So many models, and it's all slight improvements, you know, kind of benchmark leapfrogging is how I like to think about it. But but if you're, if you're an enterprise looking to adopt and you've got your board yelling at you, why haven't you adopted AI yet? And you're sitting there like, I don't even know where to start. They make a new model every 10 minutes. And I think the combination of those things has just created a somewhat of a challenging environment to get people to figure out ways to actually use them, where if you think of other technologies that have come in the past, It's been a little bit more grounded in my understanding, and it's also been a little more clear. And obviously, every technology will have pros, cons, advantages, disadvantages, risks, ethical quandaries. And so in that respect, I don't know that AI is unique. But I think the scale of the hype and the speed of perceived improvement does make it different than some of the other things we've dealt with. which just makes things tough.
Eric Sydell:
Absolutely. And I think another part of that is legislation and regulation around these technologies is so nascent. And, you know, we have what's going on with, you know, our own government and administration. In the Biden era, there were executive orders on AI, the blueprint for how to use AI ethically. Trump has gotten rid of that, and more of a deregulatory type of environment. organizations, businesses are like, well, what should we do? You know, is it going to be legal? Is it not legal? You know, how should we do this in the right way? And so, you know, you have a lot of states in the United States, there's a lot of states and even cities that have enacted, New York City, for example, that have enacted their own AI legislation. So it's this patchwork of regulations. And then globally, you know, same thing, right? I mean, the EU is pretty advanced in its regulations. the EU AI Act, and you've got other things like GDPR that are relevant for this type of technology. And then globally, every country's own thing. So it's very confusing as an organization, like, well, do we invest in this now? Knowing that, like you said, the technology itself could be revolutionized next week or whatever, or made illegal or what. So I think it's an interesting challenge for business because you have to innovate and you have to stay in front and you want to make use of these advanced technologies, but you're scared to and you don't know how to. And also it's super confusing because what even is this stuff? You know, a lot of people don't understand what we mean by AI or generative AI. So I think there's just a lot to think of. And so like for us, what we're trying to do is you don't have to worry about a new type of LLM being released that's going to be better than one that's already there because you can just change which LLM our system uses right in the system. And if you want to use OpenAI, great. If you want to use LLAMA from MetaGrade, you know, whatever you want. Or if something else comes out that's not even an LLM, you know, that's different or better somehow, great, we'll integrate that. So that's our job. You know, as a business user, you just get to focus on what it does, not the underlying technology. And we're not building our own LLMs. We're not open AI here with a data center. You know, we're just building on top of whatever the best is. So we deal with that. We work with it. The other thing that's interesting, I think, when we talk about the legislation piece is If you want to know whether your business process is likely to be in line with current AI legislation or future AI legislation on a global level, guess what? We can automate that and we can tell you that. So you can upload documents and information and overviews and plans, whatever you want, into our, you know, RAG-based system, i.e. secure system, and then we can map it to not only current legislation globally, but even emerging legislation, even stuff that hasn't been approved and ratified yet, and we can immediately evaluate, is it likely to be in alignment with, you know, some regulation from, I don't know, Morocco, or, you know, anywhere globally, right? And so this is a way to help reduce risk, I think, and help companies, when we specifically talk about AI technology. But it's an example of what this type of approach can do.
Ian Krietzberg:
Yeah, we can dive deeper into the regulation thing. It has been a persistent question, and like everything in this field, point of debate. I think ever since Sam Altman took the mic in front of Congress and said, If this technology goes wrong, it could go very wrong, right? My worst case scenario is lights out for all of us. These are quotes from Sam that are just imprinted into my skull for whatever reason. And I think in a lot of members of Congress as well and that that kind of touched off a movement. Now, the EU's AI Act has been in the works for years before that moment. But there's a lot of, like you mentioned, it's a patchwork assembly from local municipalities to state governments to federal governments, the bloc itself, but then also each different country has its own different approaches, which makes compliance really hard for everybody. But even the nature of the regulation itself, people are starting to talk about the idea that it's coming from a place that is misguided, because you have legislators that are attempting to get a handle on something that they don't really understand, that is moving at a speed that is hard to get a handle on, and the speed of that advancement is not slowing down. And there's very few, it seems, highly tech-enabled AI experts in these different legislative bodies. And so the rules, like you would hope, if we looked at the EU's AI Act, I think the intention is pretty clear. They want to optimize themselves for the use and application of it. which is why there's no, like, they have exemptions to their prohibitions for governmental use and for military use, which is, that could be a concerning point. But there's also a focus on, you know, civil rights and consumer protection, which was the dual focus as well of Biden's executive order, which as you mentioned, we no longer have. Um, and so I wonder what you think about all these different approaches and the places they're coming from. And, you know, if it's true that it's kind of to, to regulate, this is almost misguided from the start because, and it starts from a misguided impression of what AI is, which you mentioned earlier, right. AI is a result of a series of technologies that are not regulated. It goes down to the chips in the data centers and it goes into the networks and, you know, the internet providers and social media itself where they pull the data from and where it's integrated, right? And so if you're going to attempt to regulate the thing at the top, but you're not touching anything else in that stack, it's very challenging. And I guess that's the theme so far of the show, right? It's very challenging. But I wonder what you think about these approaches and what a good approach might look like and whether or not anyone's doing it. For sure, yeah.
Eric Sydell:
I agree with everything you said. It is super challenging because no one even understands what AI is fundamentally. I maintain that most people think it's a scary robot from the future sent back in time to kill us. And then when you talk about generative AI, people think of image creation and just fun stuff like that. But these technologies are so much deeper than that and so much more nuanced. And they're broad, there's a lot of different types of AI. So what are we supposed to do with that and how are you supposed to get a handle on it from a regulatory perspective? To me, and I always simplify everything so that I can understand it. And to me, when I think about AI and what do I want out of AI or any technology, to make the world better for humans, for human beings. And I'm a psychologist. I don't know if we talked about that, but my background and my entire company is actually psychology, not engineering, not law, not compliance. You know, I'm a social scientist. That's how I'm trained. And so I want to figure out ways to make the workplace better for humans. That's my training. That's my background. And when I think about AI or any technology, I want to apply it in a way that helps humans, not collectives, businesses. I mean, we are in business to serve businesses, but we have to do it in a way that's humanizing and not dehumanizing. So to me, the ultimate litmus test of whether we can and should be using a certain technology, whether it's AI or anything else, is Does it improve the individual life and work life? Does it improve a human's experience? And if it is only designed to wring the maximum amount of productivity from a workforce, you know, or to surveil us in some other way, is that the world we want to live in. I mean, we're all humans. I'm a human. You're a human. Everybody listening to this, well, I don't know, most people listening to this are probably humans. We want to improve the world for us. Right? And even though these companies and we, my company, we sell to collectives and to businesses, we still have to do it in a way that's good for the individual. And, you know, I don't know if you read a few years ago, there was a book called AI Superpowers by Kai-Fu Lee. And there's a passage in here, which can I read it? It's short. Go for it. Sure. I mean, it's very psychological, which is why I really loved it. But he said, The real underlying threat posed by artificial intelligence is tremendous social disorder and political collapse stemming from unemployment and gaping inequality. Tumult in job markets and turmoil across societies will occur against the backdrop of a far more personal and human crisis, a psychological loss of one's purpose. And that to me is very, very powerful. And so I'm like, what are we doing about that? This was written in 2017, I think. So it's old now. That's where things are headed. So what's our plan? What are we doing about that? So to me, when I think about harnessing these technologies, I wanna harness them so that humans have a better work life and a better experience. Um, and, and there's a lot of forces that don't care about that and that want to just, you know, maximize the productivity of humans on the way to replacing us with fully automated robots. And if that's the case and that's where we're headed, then I can't stop it. But I, I, you know, I I'm concerned about what that world looks like.
Ian Krietzberg:
Yeah. I mean, I, I think a lot of this comes down to balance and I think finding the balance is very, uh, I'm gonna say it again, it's very challenging. Because, I mean, fundamentally, right, what you're talking about, putting humans first, how do we better humans? How do we better ourselves? I think if you talk to people who have been in this field for a very long time, that was the inception of it. The idea behind self-driving cars even is because people get in accidents. Can we use technology to prevent that from happening? Can we save lives? I think that was the inception. I think what's going on now, that has changed. And the idea of AI as a productivity tool. We can leave AGI and all this other stuff out of it. Just AI today as a productivity tool. You know, for some applications, for some companies, the offering is not one necessarily that impacts jobs, because it's automating something that no one else was really doing before, so it just helps. For other applications, the only way it's worth it for a business to pay for a massive enterprise subscription to ChatGPT or whatever else it is, Is kind of predicated on the idea of I'm not going to hire anybody or I'm going to reduce my staff and how far can we take that? because the idea is really do more with less. And on top of that, there's this added risk of by doing more with less, you're automatically straining the people that are still there. And so there's been reports from nurses in hospitals where AI has been implemented, and it's being used as a method of we're gonna save on hiring, and now you have to see 20 times more patients because you can because of this tool. Which is this, I mean, we talk about balance, I don't know any way to ensure balance when it's driven by the baseline corporate need of the bottom line.
Eric Sydell:
Yeah, I mean, I and I worry about this too. I mean, you know, we're obviously building technology that is automating to some extent of what a human does. And so natural thing that we think about a lot is what are we building towards? You know, where is this headed? And so right now in the compliance domain that we're in, We know that most companies really struggle to comply with all the frameworks out there. There aren't enough humans to do this work. And it's grueling work, too. If you're an auditor that's been tasked with an audit that takes 700 hours to do, which is what it often takes for something like a high trust audit to be done. That's a ton of reading of nuanced stuff. It's hard work. It takes a long time. So the goal is with what we're doing right now is that we can help them be more effective and regain some of their life and maybe do more audits and be more productive, but in a way that is less draining. And I think there's plenty of opportunity for that right now. The bigger picture, the longer term is, you know, as a society, as we build these types of increasingly automating types of processes, what is the plan? And I think if you think of, you know, an optimistic vision of our future, And there's a new book, by the way, Derek Thompson and Ezra Klein's Abundance. I'm not sure if you've seen that or if the listeners have taken a look at that yet, but it presents a very, it presents an optimistic and I think exciting, compelling vision of what we could build towards, which is sort of a future utopia, you know, based on high tech stuff and using it to make the world better, using it to make the climate better and to make our working lives better and everything, you know. But in so doing, there has to be social systems and systems that support humanity. Otherwise, we're all going to be, you know, twiddling our thumbs and bored out of our mind and probably murder each other. So, you know, there has to be things for us to do. There has to be ways for us to live and to generate income and all these things. This is the job of government and society to figure out what the world looks like in a in a future that is exciting and compelling and not negative. And I think that the book is exciting to me and the thinking is exciting to me because it paints that sort of optimistic picture and it says, hey, this is cool stuff, yeah, we have issues to figure out with it, but that doesn't mean we stop innovating and stop building necessarily, it just means that we have to figure out what it means for the future of humanity so that we don't wind up in a, you know, Kai-Fu Lee's dystopia where no one has any purpose. Let's solve the problem, let's figure it out, you know, let's create a world where we don't have to do all this drudgery, but where we're also taken care of. So that's the conversation I think that is that we need to have. And the problem with that is that it's down the road. So it's never, it's a distal thing, not a proximal thing, you know? So it's like hard to get our politicians and our leaders to actually focus on this somewhat fanciful sounding future, right? But we need to recognize that it's real and it's happening like crazy, crazy fast, so.
Ian Krietzberg:
Yeah, I mean, the politics are reactionary. And so we're kind of automatically limited by that. And I guess that's my problem with the idea of abundance. I think I have a bunch of problems with the idea of abundance, probably. But one of them is that notion, which is, you know, two sides of that, again, that broad term of AI. And one is what we have today, an overhyped but very useful tool that comes in a lot of different forms. And basically, you're just talking about algorithms that can aid our work in certain applications. which has huge implications for research, right? In the hands of scientists, a machine learning algorithm and 5,000 pictures of some whales out in the ocean can help us do so much work in conserving those whales and in understanding that ecosystem. And the same goes for coral reef restoration and wildfire prediction and cancer pre-diagnosis and all these other things. That's a very real thing. Then you have, you know, everything taken to the extreme, and that's the kind of other side, and it's hard to engage with because it is hypothetical. We don't know that we'll get to a place that we'll get there, where the algorithms become more than just algorithms, and the idea of these kind of instantaneous solutions to problems that have vexed us forever. And it is attractive, right? Like these companies do paint these pictures of a near term utopia on earth. Which, yeah, that would be really nice. But running these things down, right? I guess it's hard to look at that possibility as separate from where it's coming from. And where it's coming from is from for-profit corporations who aren't incentivized to really use these systems altruistically. And separating the image they're painting with the work they're doing is challenging for me. Feasibility of that kind of world of abundance aside, and I think issues with energy constraints, resources, climate change, these things will probably be big curtailors of that environment, but can you separate, you know, what it might be able to do, or what they what they show you, that might be possible from what they're doing. And even if you think of, you know, recently, OpenAI submitted policy proposals, right, we're just talking about regulation. And then it's the same thing, right? They're talking about that era of coming abundance. And they're saying, to get there, and because it's important that we get there ahead of China, you have to exempt us from current laws, like copyright protections, which, as it is, you know, people that work in the arts are, you know, there's not a lot of money there. And the idea here Taking that to an even further extreme exempt me from licensing regimes exempt me from copyright lawsuits I don't want to pay him anymore because utopia is within reach and we can't pull that down And so I think that the separation of where it's coming from is really really hard and I don't like it and it's it's harder the further you take it along because what do you do and Do governments step in? But if governments step in, then are governments in control of it? Is that any better?
Eric Sydell:
It is hard. It's super hard. And I think that when I, you know, hear about the idea of abundance and this kind of future utopia, that's what I want to work towards. I'm not sure if it's doable or not, but that's what I want to work towards, because what else are we going to work towards? So I hope we can figure out ways to get there and that the public will. understand the importance of that and get behind politicians who support it and things like that. I don't know. It's tough. I guess what I'm trying to do and my lens at my company is to help us understand the data around us, which includes understanding whether and how systems like companies like OpenAI are performing and what they're doing. I want VeroAI to be an engine of observability. To be able to monitor outcomes is what's really, really important to me. I don't care how the algorithm is written. I don't care if it's efficient or not or if there's a better way to write a line of code. I don't care about that. I care about what comes out of it. I care about the thing that we can observe in the real world and monitoring and harnessing and understanding that. And that's sort of behind our you know, our approach. And in fact, when we started, we, I actually came from HR technology. And so we started with an approach to sort of harness and understand hiring decisions specifically, and understand whether they're biased or not biased against protected classes and understand whether they're predictive of job success or not, i.e. useful to a company or not. And it was interesting because we didn't find a lot of traction for that. And you would think, well, don't companies care about that? And we think, well, they should. And in some level they do, but maybe not enough to have a process or to pay for a process that monitors it. Instead, what's easier to do is to just take a vendor's word for it, that the product works and is good. And that's easier, it's cheaper. So we'll just accept that this thing works, but not really critically evaluate it. And that leads to, not just in hiring, but in every domain, a lot of garbage, a lot of business processes that people pay for that don't work, that lead to compliance problems, and that ultimately humans are very susceptible to hype. And so, I mean, as again, you know, we're scientists, I'm a scientist, I want to make objective and rational decisions about the information in the world around us. And we're using these tools to the best of our ability to help people do that. And that's what we can do. And that's what I'm trying to do. And so we don't have all the answers. But we're trying to fight the good fight in the sense that, you know, we want companies to be able to really understand and evaluate complexity and these complex systems and get beyond the hype. And so I don't know, you know. That's all an individual can do, I guess. And I don't know where all this stuff is going. And it's frightening because the hype of everything is, hype powers the economy. So how do you get beyond that? How do you get beyond that to stuff that really works or doesn't work and understand that? And we're trying to create one way to do that that I hope is compelling. I think about like, AI is like nuclear power. It's pretty powerful. It can solve a lot of problems. I mean, nuclear power is green and can solve a lot of the energy issues we have, but it's also dangerous and people are scared of it. You wouldn't build a nuclear power plant down the road and then just skimp on the monitoring of it and have your, you know, your dropout buddy Kevin go check on it every couple months to see if it seems like it's running okay. But that's what we do with most AI systems, you know, and with tons of the high-tech solutions that are out there is we're just like, oh. Well, the company said it works, so let's pay him a million dollars a year and we'll implement it. And then everybody gets to pat themselves on the back and make it look like you were making an advance and using something that's good. And nobody ever takes the time to actually really look at it and understand, does this actually do what it's supposed to do? And a lot of times, in a lot of cases, it doesn't. In a lot of cases, that thing is melting down. But you're not watching it, so you don't really know.
Ian Krietzberg:
It's very interesting where we're at. And I think in many ways it's, it's the kind of necessary or unsurprising or perhaps very surprising, um, evolution of our pathway from the internet, right? We've been interacting with algorithms and we've been, in some ways, our behavior has been controlled by them or influenced by algorithms for a long time. And our expectations from coming from the era of, like if the 2010s was the era of social media, why was that a hard word to say? And coming from that era of immediate accessibility and comfortability with digital technology in a way that never really coincided with I think there's probably actually a steady decrease in critical consumption of media or technology the more these things have integrated and proliferated in and among our lives. And coming into AI and increasing automation from that point where our, if our guards were up in 2010 by 2019, they weren't, you know, this is the algorithm, this is social media, get my notifications rolling. Uh, I got to check in on stuff. My iPhone, my Apple watch, my Mac, my iPad, my, you know, smart TV, it's all, it's all hooked up. And it's interesting because I feel like the expectation would be increasing automation would be just another elevation of we can keep stepping back a little bit. But I think the reality is that in order to actually use these things, to actually derive advancement or assistance, we have to go the other, as a society, as a digital society, we have to go the other way. We have to become more critical consumers of rote information, whether it be output in a language model or a post on Twitter. We have to be more critical about the sources of data, what something was trained on, where something's coming from, what something does versus what something says it does. And I think on a cybersecurity front, that's a big challenge. Right, because you're talking about what people have become easy to fool because they trust. And just on the usability front, Anna, can we actually leverage the good stuff without getting bogged down in all the bad stuff? Not an easy task because you're talking about a societal shift. And I mean, as we've mentioned, you have a background in psychology. And you've done so much work in technology. And I wonder how you look at the way humans interact with technology and how that all relates to what we should be doing, should be thinking about, consider approaching, whatever it is, when it comes to this wave.
Eric Sydell:
Yeah. And certainly, obviously, I don't have all the answers. But to me, AI, Generative AI, large language models, machine learning, deep learning, all of it is technology that allows us to understand the world around us in some measure. So fundamentally, AI allows us to understand the world. Only about 20% of the world is numeric. Only about 20% of the data in the world is numeric. 80% is unstructured, qualitative. It's text, it's images, it's screenshots, it's videos, all that stuff. And there's been no way to systematically study and understand that stuff at scale. I think that large language models and other deep learning things, machine learning techniques, give us ways to study that 80% of the world's information in a more scaled, objective way. And there are obviously issues and problems, hallucinations and this and that. with these types of technologies, but fundamentally that's what they do. And so when Elon Musk says he has a full self-driving car, it's fundamentally scanning the environment and taking in that data to try to determine how to drive that car. It's processing the environment. It's making sense of the environment. Now, that's purely good. The problem you get into is when they call it full self-driving, And it's not, right? So then you get into this level of marketing hype. But fundamentally, if you just look at the ability of the car to read that environment, it's pretty good. It's not full self-driving, but it's pretty good. So AI gives us this way. to study the world around us. It's investigative. I think of AI as an investigative statistical technology. And full stop, that is good because it allows us to understand more about the world around us. And it makes us, it helps us to be more objective and to make more rational decisions. And that is a beautiful thing. I mean, as a scientist, that's what I care about. The scientific method, I think, is the greatest invention in history, better than LLMs even. It's a way of thinking and we can all use it and we need to use it because there's so much noise out there. To me, that's ultimately what we're trying to scale and build is a way to make sense of the world around us. Quick story. On December 20th, 1996, it was my 23rd birthday, my parents gave me a book by Carl Sagan called The Demon Haunted World Signs as a Candle in the Dark. And in that book, Carl Sagan has some extremely, you know, impressive passages that seem like they could have been written like last week about the state of the world and disinformation and, you know, grabs for power and autocracy and things like this. But he's fundamentally talking about using the scientific method to understand the world around us. and to combat pseudoscience and misinformation and disinformation, which of course is so prevalent today. So to me, that book, that book formed my, that created my life, that created my trajectory is reading that book. Carl Sagan died the same day, December 20th, 1996. So that's always stuck in my head as that day. It was something that transformed, I guess, my trajectory and led me down this path. This is about, applying science and AI or any other tools to make better sense of the world around us. And I think the only way we can harness AI is with AI, you know, is with other AI. Think of AI as an investigational statistical approach, more than a hyped up fun consumer-oriented thing. Because fundamentally, that's what it allows us to do is study information and pull insights out of information that we could not previously access and do. A further development and application of what we've built at Vero AI is a fact checker. an automated fact checker. Like what if you're watching a presidential debate and across the bottom of the screen in real time is a percentage of accuracy. It's being checked instantaneously against multiple different fact-check databases, some funded by progressives and some funded by conservatives, right? So it's subjective. I mean, that's an example of what Ethan Mullock is talking about. Like, that's possible now. It's just that nobody's built it yet. We have the technology to do that now. Um, so, you know, that's, that's, that's cool. That's exciting. And that's what we're trying to build towards. Not that specific application, but things like that. Yeah.
Ian Krietzberg:
Yeah. I, I think that there's even some research in that. Um, uh, I believe Preslov who's at, uh, NBC UI works a lot on fact-checking misinformation, uh, using machine learning models to, to, to do that. And, and yeah, to your point. that's achievable now. And then it all just comes down to trust. Trust and responsibility. And what you're talking about is very interesting, even from the self-driving example to people can leverage this technology as a means of enhancing the scientific method in whatever practices day to day in their lives. Is the onus, I guess, on people to sink into these things, to understand these things and to dive into it? What would you say to or what would you think about take a very opposite tack and go, you know, I don't want anything to do with this. I just want to live my quiet life and read my books. We have too much digital technology. It's proliferating too quickly. People are getting left out. People are getting hurt. There's environmental impacts related to the data center that are not being addressed. You know, the data sets are trained on systems that were done without proper licensing. I don't want to touch it. And in fact, I don't need to touch it. Right. I feel like you hear that argument a lot, especially from at work, higher performers and their studies, the names of which are not at the top of my head. the higher performer you are, the less you stand to gain from something like this necessarily, right? And so it's an interesting, I do think we're seeing a divergence where, to your point, this could be leveraged, and then other people are kind of, you know, a different camp is taking a stand against it. And not that they don't want to be informed about it, but that, and I don't know that they're scared about it. either are scared of it. I think it's just a thing of, you know, potentially also in the camp of, I would like to throw my iPhone into the ocean and just sit beneath a tree because this is all just too much. And it's the kind of the closing in the walls of this digital environment that we're now subject to. And it's, it's, I don't know. Do you have any thoughts on that? I think I got a little rambly.
Eric Sydell:
Well, I mean, I think it's right. I, you know, I don't disagree that there's that impetus. And I feel it too, you know. I mean, I love to unplug and get away from this stuff. And, you know, I mean, we were talking earlier, you know, I like to build stuff in a workshop with my hands, you know, like, what? That's like the complete opposite of what we're doing in front of computers all day long is, you know Just physical labor and physical tasks and being in nature and these things are super compelling and we should all do more of that So, I don't know it's a balance and I don't know how you stop the the train, you know of technology and progress I mean our whole entire economy runs on innovation. We have to innovate or the economy stagnates. So, you know, it's difficult. I don't know that there's a clear solution, but I think everybody as individuals has to find balance, their own balance, and figure out how to unplug and what to do. to maintain the proper balance. I mean maybe if everything's automated one day we can just be having fun in nature all the time, right? The problem with that is of course that then society has to fund that experience in nature and that's the thing that is the broad societal conversation that I think needs to happen.
Ian Krietzberg:
then society would have to fund it. That is the fundamental tough thing. There's so many questions here that are just unanswered because they're unanswerable. But I mean, hell, if someone was able to figure out a way that I could just play guitar all day and hang out in the woods, I would not complain. So please, guys, bring that on. So I guess to finish up here, you've talked about working toward this, for want of a better word, utopia. We don't know how we're going to get there, but the idea of let's push in that direction. It's not a bad thing to push in that direction and try and get there. And in cutting through all the hype and the noise and these other things that are going on, in a more near-term world, I don't think we're going to see a utopia in the next couple of years, but in a few years, Where do you think we're going to? And you seem like a very optimistic person, so I'll just angle it this way too. What are you optimistic about in that near term, you know, say beginning of next decade?
Eric Sydell:
I don't know if I'm actually optimistic. I think I'm a builder and I'm a problem solver, and so I don't see an alternative other than trying to build towards an architect of future that we want that's good for people and humans. And so in my, in the time that I have, I want to spend it working towards things that I think are beneficial for the world. And we're building a company, we're using technology, we're innovating. And It's my responsibility to do that in a responsible way and not to do it in an overhyped way. And I think that I have to support causes that are important to me, which includes, you know, harnessing and using these technologies in a way that is beneficial for humans. That's what I can do. That's all I can do. And it's, I mean, I'm trying to do it as much as I can. Am I actually optimistic? I don't know. I'm scared. I'm scared. I'm scared of what the future looks like. I don't know that we're on a trajectory that will lead to abundance and utopia and things like this. It doesn't feel like that. It doesn't feel like we're on that trajectory right now. So we could be, we could be on it though. And if enough people talk about it, like us, then maybe it'll help us get there. So I just, that's what I can do. And I'm gonna do that to the best of my ability and I can sleep at night because I'm trying to do at least as much good as we can do. That doesn't mean that I'm going to be a Luddite and go bury my head in the sand and just read my book in the backyard, which is kind of what I wish I was doing, because I'm just not wired that way. So I'm going to innovate and I'm going to be as responsible as I can. And I'm going to speak to the causes that I think we need to speak to. And collectively, people like us can try to publicize and get out there.
Ian Krietzberg:
And hopefully, maybe. Well, world, if you're listening, we need to get on a better trajectory so that Eric can be optimistic again. Eric, it has been a pleasure. Thank you so much. Thank you for the time. Appreciate it. It's been fun.
Creators and Guests
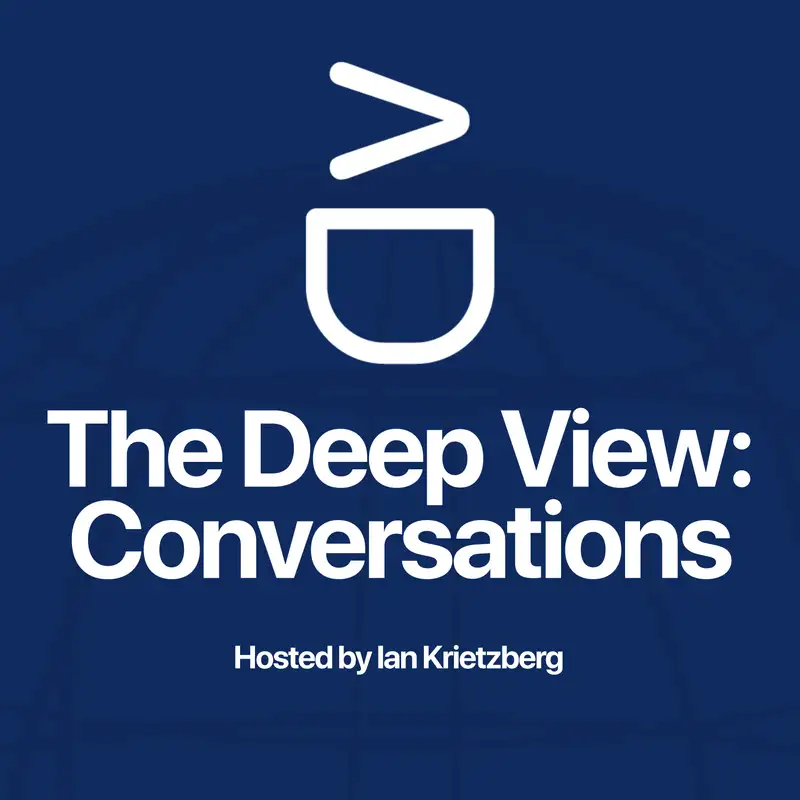