#16: AI & Quantum: Sandbox AQ's technological revolution - Stefan Leichenauer
Ian Krietzberg:
We've talked a lot on this show about AI for good. Now, it's definitely a buzzword-y phrase, but the base idea is unpacking the machine learning algorithms or the neural networks that have been trained on specific data and tuned and leveraged to do specific things to aid researchers, to aid scientists, to drive scientific progress. We've also started to talk about quantum. what it is, what it can do today, what it can't do today, and what it might be able to accomplish tomorrow, or the day after tomorrow, or the day after that. It's kind of a long loop. We don't know when quantum computers or quantum technology will be very reliable enough to be usable. Now, today we're talking about the integration and intersection of these two technologies. My guest is Dr. Stefan Leichenauer. He is the VP of Engineering at Sandbox AQ. Now, Sandbox's whole MO is that if they can combine quantum technology, which doesn't always refer to quantum computers, with artificial intelligence, meaning machine learning algorithms, neural networks, et cetera, We can drive or they can drive advancements in scientific regions and everything from drug discovery to materials generation. I am your host, Ian Kreitzberg, and this is the Deep View Conversations. Stefan, thanks so much for joining us today.
Stefan Leichenauer:
Very happy to be here. Thank you for having me.
Ian Krietzberg:
There's a lot to get into and I definitely want to dive into the LQMs that Sandbox is developing and what that looks like and differences between other systems that people might be more familiar with. But before we do that, you know, you guys are kind of, your whole MO is dealing with the combination of these two technologies, each of which are kind of very hot right now, which is quantum. and artificial intelligence. And I want to start with the quantum bit, just so we have a bit of a grounding before we get into the other bit. And with that, when you're talking about quantum, what are you specifically talking about? How are you leveraging the idea of that technology?
Stefan Leichenauer:
Yeah, it's a great question and one we get all the time. When most people think about quantum and the idea of quantum technologies, they really focus on quantum computers, right? And for good reason, quantum computers are very exciting. They represent a next level of computation that goes beyond things that we can do with ordinary computers. But that's not all there is to quantum technologies. And in particular, quantum computers, while very exciting, are not quite ready for primetime applications, real-world applications. They're still in development, right? And so from the Sandbox AQ point of view, we're more interested in things that we can do today to solve problems today. And so the kinds of quantum technologies that are more important for us today are things like quantum sensing, for example, which is another aspect of quantum technologies. Really, the whole challenge with making a quantum computer in the first place is that the little pieces that you use to create the quantum computer are overly sensitive to things that are happening in the environment. It ruins the computation. But that property at a heuristic, slightly hand-waving level is exactly what makes them really good detectors of small, tiny things happening in the environment. So you turn the bug into a feature a little bit. And so quantum sensors and quantum sensing is another aspect of quantum technology that is here today from a real-world practical use case point of view. And of course, as the technology develops overall, as quantum computers become more capable, we'll be incorporating those into our workflows and the LQMs that we'll get into naturally would adapt to having quantum computers available, that kind of thing. But for us, quantum technology, it's just a quantum piece, is broad. It's more broad than just quantum computing. It includes other stuff. And yeah, the focus is on things we can do today from a practical real-world use case point of view.
Ian Krietzberg:
Now, I got to ask, right, so what makes a sensor a quantum sensor? Is it the hardware it's made up of?
Stefan Leichenauer:
Yeah, yeah. So from one point of view, right, and if all you're doing is using the sensor, right, then at least with today's technology, you don't really need to know how the sensor works underneath. Like, let's say you're trying to detect tiny shifts in the magnetic field, right? It's a thing you can measure. Or tiny changes in temperature, right? These are things you can measure. And if I just give you a little device, a small box, and you plug it in and it will read out tiny changes in the temperature or tiny shifts in the magnetic field. And classical sensors and quantum sensors are the same from that point of view, like once you get the output. There it is. But when you look in the box and you look at how it actually works, right? And the details of what makes it function, why is it so sensitive? Why is it so capable of sensing such tiny shifts in these quantities compared to what you might've thought was possible? It's because the inner workings are leveraging facets of quantum mechanics and the details of how it works. The electrons are doing particular things that require quantum mechanics to understand and to control and to have had the idea to build in the first place. So it's really about the inner workings of these sensors that make them quantum. It's kind of the new, more advanced control of quantum systems that we have these days that enables us to make such sensors. But at the end of the day, a sensor is a sensor, and to use it doesn't require any special quantum knowledge or quantum computation in particular.
Ian Krietzberg:
It's that rooting in physics, right? And I guess because of that, is there ever any question about the validation or legitimacy of the data gathered by those sensors? Or is it always, you know, this is accurate?
Stefan Leichenauer:
Yeah, that's the advantage of having a grounded physics understanding of what's happening, is you know exactly what's going on, you know exactly what the, you know exactly how to interpret the results. One of the nice things about quantum technologies in general, actually, this is, it's sort of a, it's sort of a nice bonus that you get by working at the atomic level. Atoms are all the same as each other, right? There's no, if you have a particular atom of a certain kind, like a rubidium atom, all rubidium atoms are the same. And so it kind of eliminates a lot of uncertainty if you have a certain kind of sensor or any other device made out of a certain kind of atomic ingredient and you're really working and you care about what's happening at that atomic level, there's no variation from device to device. Maybe there are other ways in which manufacturing differences in the device can matter, but by being rooted relatively close to fundamental physics, it actually makes things less uncertain in some ways.
Ian Krietzberg:
You mentioned a couple minutes ago that as quantum computers become more usable, Sandbox will start incorporating them into their workflows. Now, I'm wondering, you know, we have some iterations of quantum computers today, and folks are obviously working really hard on them. What are you guys looking for that you would see from a quantum computer that you would go That's it. It's ready. We can start using it.
Stefan Leichenauer:
That's a good question. So the starting point for all of these things, when we're talking about any of these technologies, right, we've been talking technologies, you know, for the last couple of minutes. The starting point really isn't the technology, right? The starting point is, what do we want to do? Like, what problem are we trying to solve? And then the question is, what is the right piece of technology to enable us to solve that problem? Or can we solve that problem? Or do we need to develop a new kind of technology to solve that problem? And so really, when there's a problem that we need a quantum computer to be able to solve, that's when we'll use it. And that's when the quantum computer is ready to solve that problem we care about. Well, then we'll use it. So what sorts of problems do I expect a quantum computer to be able to solve, at least in the sandbox AQ context? We do a lot of work related to modeling of molecular modeling and atomic modeling and what's happening at the atomic scale for drug discovery and materials design and batteries and all of that. And we do all of that work today without using quantum computers. And the reason is because quantum computers today, they're not big enough or reliable enough to be able to model a complex enough system that it makes sense to use the quantum computer over a classical computer. One of the original motivations for quantum computers is that we know that when the atomic modeling gets complex enough, you have enough atoms and enough electrons doing complicated enough things, it becomes out of reach of non-quantum computer-based modeling. So classical computers, GPUs, CPUs, whatever you want to use, AI, it doesn't matter. It becomes too much, and you need something else, and that something else is a quantum computer. But where is that crossover point? Where is that point where, okay, here up to this point we can use classical computers and AI and other kinds of methods, but beyond that we need quantum computers. And the fact is that Quantum computers today are not, they're not close to where that line is in terms of being able to take us over into that new realm of complexity. We're, you know, we're talking, if I, just to think about rough numbers for those who are following quantum computing development, probably in the realm of hundreds of nice, clean, logical qubits, not the noisy qubits that we have now, but clean, logical qubits that can actually be trusted for computation. Something like that would be needed. And right now, the technology is getting to the point where we're starting to see maybe one logical qubit starting to appear in the latest experiments. So there's a ways to go.
Ian Krietzberg:
Yeah, definitely a ways to go. I like how you laid that out with the kind of the graphs of ability. And it makes me think that there's so much crossover between, I guess, what is promised and what's capable today by AI, right, which is classical computing. It's based in silicon compared to quantum and, you know, qubits and these kinds of fundamentally different approaches. So in that world, obviously we're not there yet. There's a mix of opinions on how soon we might be there, and it's really hard to predict the future. I'm not going to ask you to do it. Of course. How would that, I guess, how would you still use AI as a form of classical computing in a world where we have quantum that has surpassed what that computer is capable of doing in the scheme of, you know, modeling molecular architecture like you were talking about?
Stefan Leichenauer:
Again, it's good to be rooted in what is the problem we're trying to solve and what aspect of that problem are we actually using the quantum computer for? So in this context of molecular modeling, the quantum computer is being used to help us understand what's happening with the electrons. So in some sense, we're putting a simulated version of the molecule that we want to understand into the quantum computer, the quantum computer is acting as if it were that molecule. And so we can easily, because it's almost like doing chemistry without the chemistry lab, right? You just have the quantum computer do the chemistry for you, in a sense. But there's a lot more to a computational workflow than just that. one piece of physical or chemical simulation, right? How did I decide which molecule I wanted to simulate? How do I decide the next one? What do I do with the output of that experiment? I run my quantum computer. I get some kind of output. The output is or maybe a binding energy. So now I know here's a particular, here's how well a pair of molecules are bound together, complex collection of molecules. Okay, now that's a number. What do I do with that? So really, the part of the total workflow, if my interest is to, say, figure out what the next drug is, what is the next life-saving drug, There's a lot more to it than just how well just a couple of molecules bind together. That's only one piece. That's an important piece. but it's only one piece. And so that the picture to have in mind is a very large, you know, complicated computational workflow, right? With a lot of things happening, a lot of decisions are being made. AI is great for making decisions, right? Humans are also great at making decisions. AI can automate some of them, but a lot of that is going on. And at one point in that large workflow, we're going to want to know how well pair of molecules bind and then we're going to want to do that many many times and each time we want to know an answer to that particular question we ask the quantum computer in this world where we have the quantum computer available but if we're not doing that we won't use the quantum computer Right? So the quantum computer, when it exists, will exist as a special purpose accelerator chip for certain kinds of things, for certain very special parts of the total algorithm. And the rest of it will be work running on classical computers, just like it does today.
Ian Krietzberg:
Yeah, kind of like a sharpshooter.
Stefan Leichenauer:
Yeah, exactly, exactly. It's very good at what it does, but don't ask it to do other things.
Ian Krietzberg:
Right, right. So you mentioned the systems, you're talking about computational workflows. Let's get into the systems now. The big thing that Um, sandbox has developed is developing is the, the large quantitative models, the LQMs you're, you're kind of answered to large language models, uh, LLMs. Um, so let's just start very broadly, right? What makes an LQM an LQM and how is it different from the LLMs that we've all become so familiar with?
Stefan Leichenauer:
Yes, yes. So, as usual, I love to start with a problem statement. What kinds of problems are we trying to solve? And then what tools should we pull out of our toolbox in order to solve those problems? The kinds of problems we're interested in solving at Sandbox AQ are, a lot of them have to do with problems that are rooted in the physical world. So molecular modeling is one, sensing of various kinds is another, biomedical sensing, geophysical sensing, kinds of problems, right? These are problems which have to do not with language. Language is not really part of the ingredients in describing what the problem is, but these are problems that have to do with physical processes and how real objects in the world behave. Well, we've learned a long time ago that the language, so to speak, that those problems are written in is not human English language, it's mathematical language. And so if the only tool you had was a large language model, large language models are surprisingly effective at a lot of things. But they do also have a lot of shortcomings, and many of those shortcomings have to do with the fact that they are using human language kind of as an intermediary step in doing everything else that they do, right? And so the idea of LQM, a large quantitative model, at least at a high level, and then we can go down one level from there, at least at a high level, it's really addressing this shortcoming of language models, that they're trying to use language as a one-size-fits-all, and it's surprisingly useful, surprisingly good at a lot of things. I think everyone would agree with that. But it's not perfect. There are a lot of problems with it. It's not so reliable when you're really interested in going into new directions of say physics modeling or chemistry, can you really trust a large language model? How would it know? How would it know that it's getting the answer right? It's read a lot of information, maybe even a lot of scientific literature, but reading all the scientific literature in the world is not enough to tell you really what's the next great battery chemistry and confirm that it's the right chemistry, right? Maybe you can start to make suggestions, but do you know you're right? There are more things. There are clearly more things needed. And that's where we bring in the idea of an LQM. So the LQM is sort of an answer to that large quantitative model. And a large quantitative model, an LQM, the idea of it, there's not just one thing, like a thing that's called an LQM that is the end-all be-all of this kind of model. There's one LQM for everything. That's not the situation. It's really more, you know, it's more varied than that. It's more nuanced than that. And it depends, you know, domain by domain, what sorts of tools do you bring out? What sorts of quantitative tools do you bring out to solve the problems you're interested in may vary. And the way you put those tools together might be different depending on what you're trying to do. And, you know, we have some examples today of LQMs deployed in some of these domains, and it's an area that's still being worked on. I think the LQMs of a year from now, two years from now, will look very different, right? We're not, it's still in development kind of thing. One of the main, one of the main, components of an LQM that sets it apart from an LLM or really from a lot of other AI models is this, you know, components which can address this issue of how do you know if you're right? This kind of verifiability piece, right? And what we, you know, what we need to remember is that there's more to computation than just AI. There's more to advanced computation than just AI. We also have the ability to solve equations in more traditional ways, right? A lot of times we know, we know what the fundamental equations are that tell us how a certain system is going to behave. And we can use those to confirm that something that we think is true is true. We can use those to, we can try and solve those equations in a new area. If you have no data at all about a particular new domain, a particular new kind of molecule you're trying to understand or a new area of chemistry where you've never done any experiments, you have no data. How do you know what's going to happen? Well, there is a handle that we have, and that is, well, we have fundamental equations that we can try and solve. And it's not easy to solve those equations. It can be very challenging as, you know, as we know, in some cases you might need a quantum computer to solve those equations, but you have a way to make progress. And part of the idea of an LQM is taking advantage of that, those sorts of, you know, more boring sounding computational modules and pairing them with AI and using the strengths of AI where the strengths of AI are needed and the strengths of this more traditional, boring sounding numerical analysis where it's strongest and has greater reliability and things like that.
Ian Krietzberg:
There's a lot there that I want to explore. The last point that you were talking about, the more boring stuff, the equations that you can use to verify So the, the grounding of the models is in what we were talking about at the top data gathered from these quantum sensors. So data rooted in physics that you can trust physically is, is accurate. And then output of the models is verified. And I don't know that it seems like the system kind of comes together and you're bringing in mathematical verification, almost like a, uh, like a mathematical confidence score, right? Where it'll, it'll be able to verify it. Does this happen automatically? Is this something that you have to go in and be like, it's trying to output on this point, so let me test on this equation.
Stefan Leichenauer:
Yeah, so I think there are, this is where now we start to get into different, there are different things, depending on exactly what you're doing, the answer might be slightly different. But let me give an example. So let's say you're trying to figure out what the, some new material or some new battery chemistry or some new, you're trying to figure out what, the next life-saving drug is going to be. These are chemistry problems. These are problems at the level of, you know, does this protein, will this new molecule that I think is going to be a drug, is this going to bind to the protein I have in mind? And then if it binds, is it going to have the right biological effects afterward, right? Which of course is really the hard part of the problem. But okay, you have to start somewhere. And the problem, the fundamental issue, is that there are lots of candidate drug molecules, right? You've got a target in mind, let's say you know you're pretty confident, if I could just attach something to this protein, it would cure this disease. Let's say you know that with confidence. There are still many, many different things you can try at the level of, what is the molecule I use to attach to the protein? The number of combinations of atoms that you can put together is mind-boggling. You'll never be able to test them all. So how do you proceed? So you could take all of the data that you have so far, all the experiments that you have so far, and you could try and train an AI model to tell you what the right answer is. but probably everything that's similar to what's already been tried or what's already been done in the past. has probably been tried already. And this wouldn't be a question anymore if something similar to what you'd already done was the answer, right? Sometimes you might get lucky, but many of the problems we're dealing with today involve going into new areas that we haven't explored before. And if you just take a pure AI approach where you look at all that data and you say, all right, the AI is gonna extrapolate from the known data and tell me what to do next, Oftentimes, it gets the answer wrong. The situation is just too complex for the AI to extrapolate properly from the tiny amount of data that we have. And so the LQM answer to this problem is to say, all right, well, maybe the AI can suggest to you try going this way, but then instead of stopping there and trusting the AI, what we do is we have, we do some of this boring numerical equation solving, for example, or something similar to that, and create new data points, new data points that are trusted because we've solved the equations, trusted because we do something that's physics-based, and this gives us a new understanding of something we've never seen before. And then that data can be fed back into the AI, and now the AI has extended its understanding, and now it has a better idea. It says, oh, this area that I thought was a good idea, now I understand it better because you did something physics-based, and I now have a better idea. Now I say do this instead, or I say keep going in that direction because we were onto something. And it lets it update the information. And then this back and forth, this kind of trade off between what the AI is doing and what the equation solving is doing kind of leads you through in as intelligent of a way as you can through the space of possibilities. And then the question of, does this happen automatically? is a good one. I think, you know, at first, the handoff between these two kinds of systems is and was manual, right? There's a lot of, there's a lot of, well, expertise required to look at the output at each stage, right? And that's kind of the first step in the journey toward building these kinds of systems. But as the systems get more advanced, more and more of it can become automated. This is another very interesting aspect of AI is that you can have AI recognize its own, you can have AI go through this process automatically and say, oh, I recognize that this is, you know, the results of this calculation tell me this thing. And so now I'm gonna modify my earlier computation and keep going. Now we're sort of approaching the cutting edge of what AI is able to do in some of the more advanced areas, which traditionally would require a lot of expertise by a human in order to judge what the good next step is. the AI is now getting to the point where it can do that kind of thing automatically, which is very exciting. Because the more of this that can be done automatically, the more of this workflow that can be done automatically, the better. The more the whole thing can scale and be more effective.
Ian Krietzberg:
Now, these are large systems, right? They're not called small quantitative models. They're large, but they're also specialist, right? You mentioned that you're going to each LQM is going to look different in each domain that you're applying it to. And that's another area that seems to me very different from the large language model approach, which is a generalist approach kind of by its nature. They're trying to have one system trained on all the data that can do everything. And like you mentioned, it has limitations. Here you still have very large systems. But they're specifically designed to do certain things. And, you know, I wonder, A, why that specialist approach is so important, even as we're talking about more advanced systems. And B, you know, as you get down to the training data, Do you have systems that are trained first in more of a generalist way, like a kind of a general training set that all the LQMs might get, and then they're fine-tuned on specific sets? Or is it straight up, you know, if we're dealing with molecular generation, you're only going to be trained on molecular data? Like, what does that look like?
Stefan Leichenauer:
Yeah, these are great questions. When it comes to generalist versus specialist, it's true that the large language models, one of the things about them is that, and one of the advantages, one of the, I guess, part of the breakthroughs of the modern era of AI is the idea that these generalist models are a good thing to train prior to the recent advances. People had a bunch of specialist AI models doing various things, you know, translations and summarizations and all that, all different models. And now it's like, oh, wait, one big model can do it all. That's amazing. And it works for It works for language and it works for, let's say, generic kinds of tasks in language tasks. There are a couple of reasons for that. One is, one clear one is that we happen to have the internet as a training set, which has all kinds of language use associated with it and kind of covers a lot of bases all at once. Right. There's, and is complete in a certain sense, right, it kind of covers all topics. In a lot of. the kinds of other tasks that we're interested in, these sort of detailed chemistry tasks, for example, or these detailed sensing tasks. There's no such thing as a data set which kind of covers all the cases. When I was describing what makes these kinds of problems hard, it's because you're always on the edge of what's known, right? Like in every case, you're trying to push in a direction where you just don't have a lot of information about what's happened before. And so you immediately, you're always, and then once you figure that out, well now there's a new frontier. And so you have to keep on pushing the frontier and doing things that are unknown. So it's a different kind of problem. The other, I guess the other point is that even if you believe in If you believe in AGI, one day there will be an AGI that is powerful enough to actually do everything, right? One way or another. And we could debate about what such a system would even look like. I would argue that that system probably looks kind of like an LQM, but we're not there yet. And so if you wanted to, the general systems are advancing in capabilities, it's true. but they're not yet solving all problems, okay? And we can either sit back and wait for the generalist approach to kind of get around to being good enough to solve all of these very special problems we have, or we can say, certain special problems are very high-value critical problems, so let's put extra effort into solving those. And that's necessarily a more specialist kind of approach. And this specialist approach, it kind of implies or it dovetails nicely with a few choices that you make about how to build these systems, which comes back to this question of, what commonalities are there between these different specialist approaches? So, because you would like there to be commonalities, right? Even though you're trying to specialize, you wanna save as much, you wanna be as efficient as possible, right? So are there pieces which can be shared? And so the right kind of approach or a good kind of approach in this business, and it's kind of a good idea generally in software, is to be modular. So when we think of a large language model, an LLM, we think of one big model. It's got 100 billion or a trillion parameters or something, and it's this big black box. that's been trained on a lot of data and sort of does magic. And that's great. But for an LQM, that's not, that's not really, I mean, most of the time, that's not really what it ends up looking like. It really is, you know, I could make, I could describe it as a big box that does something cool. But then when I peer inside of that box, Oftentimes there are smaller boxes little modules that have been tied together some of those modules are AI neural network modules that are not a trillion parameters large, some of them are, you know, they can they maybe can afford to be smaller. because just well just because of the nature of the problem and maybe there are other modules which are these kind of numerical solver modules which are solving equations and have you know in each of those has inputs and outputs and then can be connected together and woven together in various ways and then those little modules maybe can be recycled from problem to problem. Maybe a given problem is, or a given LQM for a given kind of problem means taking this collection of modules, tying them together in a certain way, wiring them together, and then that total becomes what you might call one big model. And a lot of times it ends up looking like that. And some of these modules, by the way, some of them individually may be quite large. Some of them might be LLMs. And LQM is not a rejection of LLMs. LLMs are still very useful. They do do a lot of things. But the point is, they're not. the only, they're not the only piece. In particular, things like user interfaces are, it's great. Every user interface should have an LLM involved. And for even in these very technical kinds of problems that we're talking about, if you want to digest all of the literature, all of the scientific literature, on that problem to inform what the next step is. You should do that. You should not ignore all the scientific literature out there. And processing that scientific literature is a very clear LLM kind of problem. And so to the extent that you're interacting with any piece of literature, LLM should be part of the workflow as well. So it's sort of a mixture of different pieces some of which could be reused and some of which might be more specialized or tuned for a given problem.
Ian Krietzberg:
That modular approach, right? I mean, as you mentioned, we talk about large language models as black boxes. We don't really know what's happening inside of it. It sounds like from what you're saying that you have a little bit more insight into physically how your LQM derives an output because you know what the box is made of, you can peer into it. I guess for certain things, right, if you're incorporating some aspect of large language models or neural networks, you might have little black boxes within a box, but you still have more visibility than if you're just using a black box.
Stefan Leichenauer:
Yes, yes, that's right, that's right. And you need that. You need to be able to confirm, you know, if I just come back to this chemistry example, if I tell you, oh, here's a molecule that I think is gonna bind to this protein, there's a certain amount of explainability, which is, well, possible in that case and should be leveraged, right? You can check. You can check the answer. You can check that it's true. You can ask in a little more detailed way why the system thought this was a good idea, right? You can rerun the calculation if you think, oh, wait, that looks funny. Let me rerun it or let me check it. Let me cross-check it in some way. You can interrogate what each piece of the system decided to do in each moment. and having that kind of Transparency and explainability is, it really helps and is, I guess, in a lot of ways, necessary, right? Because you don't want the, when it comes to these kinds of things, you don't want to be bogged down by hallucinations, right? Finding some way to get around the problem of hallucinations is critical.
Ian Krietzberg:
Yeah, and I mean, I guess we sort of already talked about hallucinations or whatever you want to call them. I know that there's a lot of, there's a lot of debate over almost every phrase that's used in this field, hallucination one of them, but that you're kind of rooting things in the context of how do I know if something is right or wrong and the algorithmic verification. seems like it kind of addresses that problem, which is just kind of part of the architecture of the systems that LLMs are based on. Have you run into problems related to hallucination within the LQMs, or does the kind of mathematical physics-based approach mitigate those away completely? Is it sort of gone?
Stefan Leichenauer:
Depending on what you're doing, if you're using some kind of generative AI somewhere in your workflow, you'll have to deal with hallucinations somehow. That's just the nature of how they work. But the idea in the quantitative problem context with quantitative AI, the kind of AI that we're doing, you have handles, you have various ways to check and to mitigate those hallucinations. Let me give you another example, again, coming from the chemistry space. So previously, I described looking at walking through the space of possible molecules by kind of alternating between solving equations and then using AI to guess what the next thing is. And that's great. That's one approach. You can imagine doing another approach, which we do, which is one of the cooler things, I think, that we've looked at recently. which is to use generative AI to just create a molecule. It's kind of going more into that black box sort of direction in the same way that generative AI can create images or can make sentences appear. Why not just have it suggest a molecule, right? And that this molecule just somehow came out of its inner workings. And that's, that's great if it works, but how do you guard what kinds of, what is what a hallucinations look like in this context, well, maybe it generates a molecule that out of nowhere, that doesn't actually do the physical thing you want it to do right, but you can. you can check for that and you can sort of nudge the model in the right, you can say, oh, hey, generative model, you kind of did the wrong thing. Let's nudge you back in the right direction and have kind of a feedback loop. But there are other sorts of hallucinations that come up when you're taking this approach. because you might end up, the generated molecule might be somewhere far out in chemical space, way beyond where you have a lot of data, and you may not know how to make the molecule. Like, how do I make it? Is it something I can actually make in principle, or is this a thing that exists on a computer, and I don't know, I mean, one day I'm gonna have to make it in my lab. How do I do that? Is it something that's synthesizable? And so you can try and quantify that as well. And how easy would it be to make this thing? Do I know how to make it? And again, provide feedback to the model. to the generative model based on how easy it is to make the molecule that it's suggesting. And once again, the pattern is one of AI makes suggestions, and the more grounded modules or more grounded techniques, physics-based techniques, other kinds of techniques, kind of evaluate and provide feedback. And that kind of feedback is how we address various forms of negative hallucination.
Ian Krietzberg:
Now, we've kind of danced around specifics and I want to just kind of get into those specifics of the work you're doing. We've talked a lot about chemistry, molecules, biology, and I know For much of the industry, the idea of drug creation, the kind of drug creation that you're talking about, finding that candidate that matches, that we can maybe cure this disease, that is a hugely, I don't know, guiding light. I guess you would call it for like that. That's where people want to get and that's starting to happen. And you guys are doing work on that front. And what can you tell me? I know there's a few other specifics that I want to get into as well, but let's start with drug discovery. Where are you at in that process? What has happened?
Stefan Leichenauer:
So we are developing these LQMs that can attack these sorts of problems. And the way that we work as a company is we don't try and, like, we're not just going away and spending, you know, three or five years figuring out the best way to solve a problem and then coming out and saying, oh, here's the solution, let's try using it. We're working with partners and customers the whole time and battle testing our solutions as we go and seeing them work in practice. And actually, if we're not making a difference, then we have to try something else. Even Even in the first year, maybe the LQMs that we're using are relatively primitive, but it tells us, hey, we're onto something and then we can advance. But it goes hand in hand. And so we've already had a lot of success in drug discovery, in particular, working with some of our partners and customers using some of these methods that I was talking about. So, like, as an example, we're working with the Stanley Prusiner Lab at UCSF, who are working on neurodegenerative diseases, things like Parkinson's and Alzheimer's, very difficult kinds of problems. And before we got involved, they spent a year physically screening 250,000 molecules looking for a match, looking for something that would work. And they had a very, very small hit rate out of this 250,000. They found like 25 or so possible candidates, which is a very small fraction of that 250,000. And it took a year. And then when we got involved, we said, all right, let's use one of these LQM-powered computational searches, right, rather than a physical search of something of the kind that I was describing. And in a month, in something like a month or something like a timescale like that, we were able to go through computationally a library of over five and a half million molecules, and we recommended at the end, hey, here's the top 7,000 molecules, right? rather than screen 250,000 over a year, take these 7,000 that we think are the best ones and only screen those. So that's like a big cost savings. And the hit rate, like the number of the, you know, the fraction of that 7,000 that ended up being worth taking to the next stage was 30 times higher than the previous screen that they had done. And so it's a savings of time and money and various efforts and is kind of more successful. And so these kinds of techniques, they work, right? They show a lot of promise and it only goes up from here.
Ian Krietzberg:
It's a really interesting thing, right? The give us a month, we're going to go crazy with our things. Now, now take a look at what you've got. It's kind of a more targeted approach when we're searching for needles in haystacks. Um, you know, the, the LQMs can find them faster. Now I've got two questions based on that. And the first has to do with the kind of pipeline of finding that needle and getting it to a point of even a clinical trial. Right. Obviously this is a massive acceleration, um, being able to go through five and a half million in a much shorter timeframe. And then. Here's the 7,000 best right now. The researchers have a more targeted kind of approach, but can this approach impact anything further down the pipeline than that just initial discovery phase? Cause then you have wet lab research, clinical trials, get a pass the clinical trials. Is there any. Is this the only place, I guess, at the inception, at the search, that an LQM can really accelerate?
Stefan Leichenauer:
That's a great question. That is sort of the question, right, for what comes next. And the answer is yes, LQMs can make a difference, but of course, the problem is a much harder problem. And there's a good reason for that, right? The reason is that once we move from chemistry and physics up to biology, It's more complex and no matter what kind of computer you have, even a quantum computer, nobody expects to be able to really fully faithfully simulate the entire chain in a physics accurate way of what's going on. But that doesn't mean there aren't things you can do, right? It just means the set of tools changes a bit. And so what you want is a quantitative and reliable understanding of, say, cause and effect within what's happening inside of somebody's body, right? If I wanted to know, like, the next kind of question to ask, besides, does this molecule bind to the target? Is this molecule going to be toxic? to the person if they ingest it, right? Like what else does it do in the body? And this can involve, this involves having some kind of understanding of, well, biological processes. And if I tweak this part of somebody's inner biology, what are the cascading effects of that? And having a representation, a computer representation of all of those different possible cascading effects is the sort of the data structure and the tool that you might try and use to solve this problem. And so part of the next phase of the mission, or the current phase of the mission, is to build up that kind of knowledge base, that kind of level of understanding of what's happening. And you can build that sort of thing up piece by piece by looking at, say, all of the data that's out there and digesting it and saying, all right, well, all of these clinical trials were done, for example, which taught us this and this and this and this. Let's synthesize all of that together and create some map of how we think the inner biology works. And then we can ask, well, does this suggest anything that hasn't been tested yet? And some of those linkages, maybe you can confirm or check, or maybe you have suspicions, and there are certain computational things you can do. to confirm or disconfirm certain causes and effects that you think might be the case, and just build up over time, and through a lot of effort, a better understanding of what happens. And this sort of thing, it's hard to say if it will ever be perfect, at least within our lifetimes, but there's a lot of reason to believe that progress can be made, because if you can improve on just throwing darts at a dartboard, then you've made progress. And so that's definitely the next kind of phase of things to incorporate.
Ian Krietzberg:
Right, yeah, biology is super, super hard. Now, the other question I had, right, you were kind of mentioning costs and cost savings of, you know, if we can do in a month what would take you a year of research, you can be a lot more efficient with your funding, et cetera. Now, something we know about large language models is that they are incredibly costly to train, to perform inference on, as we're getting into the kind of reasoning models, right, that have become very popular, the cost of inference is rising, this is why NVIDIA has been so happy. I wonder for you, for your large quantitative models that are these kind of module of modules, what that cost looks like. Is that a thing from the LLMs that kind of carries over, which is that one month of computation looking for those needles and haystacks is a very, very costly month? Is it manageable in the grand scheme of the payoff?
Stefan Leichenauer:
Yeah, the question of costs is an interesting one. So the costs, the usage costs and the development costs, I mean, it's not nothing, but it's also small compared to the, say, development costs of an LLM, right? Because LLMs being truly gigantic, trained on the whole internet, it's really taken to another level. But that's, I mean, in some sense, that's maybe just the point in time we happen to be at. Eventually, as things get more advanced, our ambitions will rise and we'll want to be doing more and so on. But I think from the cost point of view, part of the real difference though is that LLMs, a lot of the use cases of LLMs that people have in mind are really about cost savings. Like, can you use an LLM to save costs somewhere by being more efficient? And then you have to balance that against, well, the price of actually executing queries using an LLM, because they're big and expensive. Price is going down all the time, but anyway, it's a trade-off that one has to consider. When it comes to these LQMs and the problems we're trying to solve with them, the calculus is totally different because we're really doing a lot of value creation with the problems we're solving, right? So if you had to spend, you know, X amount of dollars to save Y amount of dollars, well, then you're, you know, that's what's bigger X or Y. But if you're spending X amount of dollars to possibly create something that is going to be exponentially valuable, like a new battery or a new drug or a new life-saving medical diagnostic device, right? The value of the answer, the value of the output is, well, it's much higher and it's much different. It's a different kind of thing. The upside is tremendous. And so the way you think about the costs is very different.
Ian Krietzberg:
It's an interesting way to look at it. Now, I've got two more for you. I know we are running out of time. That happened very quickly. And the other one is the kind of specifics, right? We've talked so much about the drug development process, the challenges of biology, chemistry. You're also doing work on materials discovery, which I feel like, you know, comparing the two, materials discovery maybe doesn't get talked about as much. But it's really interesting, and it has big implications. And what can you tell me about the work that's going on on that front as well?
Stefan Leichenauer:
Yes, yes. So materials, it's itself like a big topic, right? And so within that, there are several things. But the basic The basic idea is similar at a high level to the one of drug discovery. It's about can we find something that's chemistry and physics based that's going to advance us in some direction. So can we find a new way of making batteries which has the right kinds of energy density and isn't going to catch on fire and isn't toxic, that kind of thing? Or can we find a new catalyst which can change the chemistries of some reaction that we care about, which will lead it to be more efficient and maybe greener, right? Green chemistry is a thing. Or can we find a new material for, for vehicles, which is going to be lighter, but still have the right kinds of strength to protect the people inside but be, you know, allow the vehicles to be more fuel efficient, that kind of thing. And so it's in all of those cases. It's a really, it's sort of a search problem. It's a search problem through this ginormous space of possibilities with constraints, right? With complex constraints, multiple constraints that need to be optimized. It can't be, it can't have all these negative properties, but it has to have these positive properties. And so all of the things I just mentioned are things that we're working on. And the approach is the same kind of approach. A lot of the same LQM modules that we were talking about in the drug discovery case, like I mentioned, hey, maybe you generate a possible answer, generate an example of a new catalyst that you think is going to make this chemical reaction more efficient. But then deal with the hallucinations by providing feedback that says oh actually the chemistry is slightly wrong actually I don't really know how to make it actually it's got these and have those kinds of feedback loops. So the the ideas are very similar and a lot of it. A lot of it can be reused because it's so similar a lot of the modules can be reused. As we were saying at the start of our conversation, at some level, each of these things is a special area and you need to do some kind of specialization. The amount of specialization is something you don't really know ahead of time until you kind of dig into it to try and shoot out ahead and really get something that can be transformative within those very high value problems.
Ian Krietzberg:
Now, in our last minute here, I'm going to throw something back at you that you mentioned earlier, which is that you're kind of working at the edge of what is known, which is a very cool sentence. But, you know, because of where you're sitting at this kind of frontier of scientific advancement in these specific areas and regions, what kind of outlook do you have for the next few years of what kind of transformations, you know, wild or gradual or exponential that we might actually see and experience and witness coming soon.
Stefan Leichenauer:
Yes, yes. So I think, you know, there are two perspectives on this sort of future outlook. One is, what kinds of problems do we think we'll be able to solve? I love taking this problem first approach. And I think the problems, some of the problems that we're trying to solve today, I think will be solved in the next few years. The things that we've already started on. So something we didn't talk a lot about was like medical devices, which comes back to this idea of quantum sensing. But in the next few years, There will be some from Sandbox AQ, maybe some from others. There will be new medical diagnostic devices out there in hospitals saving lives, and it's going to be amazing. There will be some drugs getting through clinical development or clinical trials that came from these AI-based searches, like those kinds of things, the fruits of today's labors will have yields in the next few years. From a technology point of view, I'm very excited and bullish about AI agents. I think AI agents represent a next level of automation. It's, I think, an opportunity as things like LLMs and the base models themselves become more and more commoditized. This next layer, this agentic layer, is really about It's not just, oh, here's a large language model, have fun with it. The agents are really about, now let's solve a problem with it. Let's now go one level up with our automation, take all of these tools, right, all of these different kinds of modules, and actually do things. And I think there's a lot of potential there. We'll see how it pans out, but there's a lot of potential there to supercharge actual real problem-solving workflows, not just black boxes, but things that are actually performing a task. And now that you're performing tasks, it's going to lead to greater acceleration on real results and real value and value creation.
Ian Krietzberg:
Yeah, no, it's a very exciting time. I feel like we just scratched the surface, but thanks so much for coming on.
Stefan Leichenauer:
Thank you. Thank you for having me. It was great.
Creators and Guests
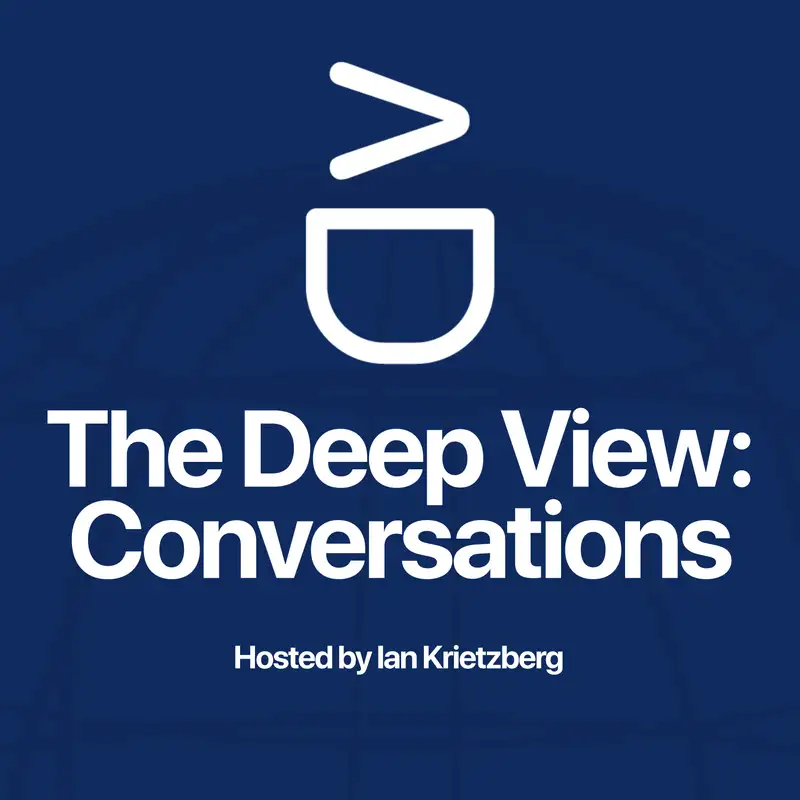