#15: Tools, not gods: IBM VP on AI, neuroscience and the nature of intelligence - David Cox
Ian Krietzberg:
Today's episode is going to look a little bit different than it normally does. It was recorded on the road at the Human X conference in Las Vegas. And so there's no video. So if you're watching on YouTube, there's not too much to look at. But that's OK, because there's a lot to listen to. My guest today is Dr. David Cox. Now, David is the VP for AI models at IBM Research, and he is also the IBM director of the MIT IBM Watson AI Lab. And before he joined IBM, Cox was a professor of natural sciences and of engineering and applied sciences at Harvard University. He holds a doctorate in neuroscience. This was a fascinating episode to record and I think equally fascinating to listen to. I am your host, Ian Kreitzberg, and this is the DPU Conversations. Welcome back to the Deep View Conversations. We're coming live to you today from the floor of the HumanX Conference in Las Vegas. Although by the time this gets posted, probably can't say live anymore. My guest today is Dr. David Cox, who is a VP of AI Models at IBM Research. And he's also the IBM director of the MIT IBM Watson Lab. And David, we connected a few weeks ago talking about some of your granite research approaches, the kind of deep research chain of thought reasoning. And I'm very excited to sit down and talk about so much stuff with you. It's hard to know where to start, but I'm just going to jump right in. I've got some stuff pre-written here. So you have a doctorate in neuroscience. Now, I always get excited when I see stuff like that because AI is so interesting to me because it is very interdisciplinary. And a lot of the approaches that we're seeing are these attempts to mimic or replicate human intelligence. And to me, why don't we start with that? What is human intelligence? What is human cognition? What is going on in the brain when we're talking right now? And, and I wonder what you can tell me about all those points and other big questions.
David Cox:
Yeah, where to start? I mean, so, you know, it's interesting. I'm kind of like an oldling in AI. It's such a young field, it's moving so fast. And in the early days, there were actually a lot of us coming from, you know, it's like, you know, on Tatooine and Star Wars, Mos Eisley, there's this weird bar with all these weird alien creatures. Early AI, there wasn't really a discipline. Obviously, there were computer science people, there were statistics people, but there were physics people, and there were a lot of neuroscientists coming in. And, you know, I think that's part of what's kind of cool and exciting about the field. You know, there's just so much we can learn from neuroscience to get inspiration. And I think that's why so many of us who started in neuroscience sort of crossed over over the years. I mean, obviously, LLMs, what we call AI today is deep learning, which is artificial neural networks. It's been rebranded a bunch of different times. But, you know, it takes its original inspiration from how neurons connect to each other and all that. And, you know, I think that's really the interesting kind of connection point, though, is really about inspiration. Because there's whole threads where the people are trying to model and replicate brain activity. those haven't necessarily always panned out. They're very interesting if you want to understand the brain. But there's so much inspiration we can get, you know, thinking about how we think about, you know, even in the chain of thought, you mentioned reasoning models recently, which are obviously a big, you know, hot topic. Just intuitively, we think, right? Like, I have to mull it over. I think. I have an internal monologue while I'm thinking, right? And actually, you can even, in many cases, decode from brain activity what the internal monologue would be. You can, if you do transcranial magnetic stimulation to like disrupt your ability to speak, it also interrupts your chain of thought sometimes. So like, there's lots of interesting things like that where I think a lot of AI researchers do follow some of the neuroscience literature and what's happening there. But more could do even more. And it's just a treasure trove of ideas. And we obviously draw on that quite a bit ourselves.
Ian Krietzberg:
Your point about inspiration is kind of fascinating to me. You mentioned the brain modeling, which in certain facets we're seeing more of. I think researchers at MIT recently did a flywire model of a fly brain, which has a remarkable number of neurons and synapses in it. But so the idea of in AI, we can learn from the brain. How much is it working the other way, like digging into the inspiration versus legitimately, can it help us understand cognition? Because in many respects, from my understanding, a lot of the inception of the field right before it was really a field was as a means of trying to help us learn more about cognition.
David Cox:
Absolutely. And some of the earliest pioneers in neural networks were in psychology departments. They were explicitly trying to model human thought. Yeah, there's a huge interplay there. I think the thing that's sort of tricky is it's not like we're going to get the wiring diagram of a brain and then it's going to be like, aha, we could do, you know, if you took an x-ray, a micro focal x-ray of a computer chip, you would have to work, you'd have to know a lot of things and you'd have to work really hard to reverse engineer that. And then this is this, you know, big pile of spaghetti, you know, amazing stuff. It's like, it's like nanotechnology, biological nanotechnology in our head, like super sophisticated, but it's all evolved. It's not, it's a naturally occurring thing. It's gonna be really hard for us to reverse engineer. And I did that kind of work back when I I was a professor at Harvard for, you know, close to 10 years. And we did research kind of in that direction where we look at real brains and try and reverse engineer them. We try and build systems, artificial systems that work this, you know, maybe inspired by that. But it's really hard to go in that direction. And I think it's more about getting that inspiration of like, okay, well, we know something, there's lots of like, smoking guns in neuroscience, like, there are twice as many connections going backwards from higher areas to lower areas as there are in the canonical direction you would normally think information should flow. It's a smoking gun. Why is that? That tells us something important. But maybe I don't need to know the details of exactly. I can move faster if I use that inspiration to be creative and have ideas. The other thing that I will say about neuroscience and why there's so many neuroscientists in AI is If you do empirical science, like you're studying the natural world, you're studying your brain, you have to be super sharp in your empirical rigor. You have to design experiments that measure things and really show what you want them to show. And that's something that I think is an advantage in AI, because we have, you know, it's this amazing, we can build anything we want to build, but then you have to test it, you have to understand it, you have to build intuition. So I think that's why there's been a lot of crossover. And it's a lot of fun. Honestly, having done wet lab neuroscience in the past, like we're actually working with real brains, it's so liberating just to be able to have an idea and then just build it and then test it out and not have to do all the really hard work of experimental, you know, stuff. So yeah, it's really interesting and exciting. And I think there's, we're just scratching the surface of what you could possibly do.
Ian Krietzberg:
It's exciting to watch. And we've been talking about inspiration. I want to keep that going. Deep learning, the deep learning that you mentioned, the AI, you guys can't see me, but I just finger quoted, It's one of those very broad terms, but the AI that we think of as AI today, large language models, deep learning, neural networks, inspired by the brain, but not the same. And I wonder if you could jump into the similarities that we know of in terms of functionally how a neural network works compared to how an organic neural network works.
David Cox:
Yeah, yeah. So, I mean, at the fundamental root, the reason it's called a neural network, and we call them artificial neurons and all that, is, you know, neurons are these cells that, you know, you have them in your brain, you have them also all through your body, and different parts, you know, this is how you sense, this is how your gut knows to process the, you know, to squeeze, to push the food through your gastrointestinal tract. They're just all over your body, it's not just your brain. and they have synapses, which are connections coming in, and then they send signals forward. And that's basically a very simplified model of what an artificial neuron does. And we dispense with literally having the neurons there very quickly. We get into matrix algebra, because you don't need it for very long. But the idea that you would have, I integrate a bunch of signals, and I, you know, I do something to them and then I send a signal onwards. That's really the kernel of what artificial neural networks, deep learning, now called AI. That's what it all builds on. And from that foundation, you can go to all kinds of really interesting places. One of the things that's been most interesting to me as somebody who's kind of gone across these two fields for many, many years, is that every time we get a new revolution, it looks even more and more like a brain to me. It's like I didn't think it was possible. So I used to work in computer vision, and in computer vision you have these things called convolutional neural networks. So you kind of have each neuron kind of gets an input from a little receptive field, a little part of the image, kind of feeds up into the next layer and feeds up and then feeds up and through many layers. And I was like, gosh, that looks just like what we know about how the visual system of a mammal or a human works. And I've been studying those, I studied those for a long time, for, gosh, well over 10 years. And I thought, okay, well, this is good. Like every time, you know, the architecture matches and this also seems to work. And then if you look at the, how they represent things, like you could actually compare them directly to brains and over time, the better the neural networks got and the more capable they were, the more they resembled the activity of actual real neurons. So I was like, okay, this is interesting. There's a little bit of a caveat there, because at some point they started diverging again. But then there were things that were weird, like, well, there's too many layers. That doesn't actually make sense. Now we have transformers, and it's like, well, actually, there's a lot of features of a transformer that feel familiar in how, like, what we see in brains and the flows of information. I mean, all of this, you know, maybe it's us making just those stories, but it feels like we're simultaneously building things that are useful and we're sort of converging to some kind of truth about how these computations need to get done and how they interact. So I think there'll be more and more flow of ideas back and forth. You know, so far we've been talking about inspiration that AI can get from brain computation, but the inspiration goes the other way too, right? you neuroscientists are always taking artificial networks that are built by computer scientists and then using them to help explain the brain. So this kind of back and forth I think is really, I know it's really interesting and I think we'd be hard-pressed to say which field benefits more from that cooperation.
Ian Krietzberg:
It's the Venn diagram, a little bit of each, a little bit of blend. What you were just describing about the kind of, the advancement you're seeing and noticing that this is starting to resemble more of what an organic brain actually does is kind of crazy to think about. But so I wonder, I feel like a lot of this field comes down to specifics and the nuances within those details. And when we think about the human brain or mammal brains, How much do we not know about how those work to be able to look at an artificial neural network and say, you are resembling top line down what those brains do?
David Cox:
Yeah, we could fill encyclopedias with what we don't know about how brains work, natural brains work. And actually, truth be told, we could do the same for AI systems. Just because we build it doesn't mean we understand how it works, which is one of the weird, possibly wonderful, sometimes frustrating sort of qualities of this whole thing. But I do think, you know, one of the things that neuroscience is really good at giving inspiration to AI for is the zero to one, like there's a thing, you know, like there is a reward circuit, you know, there's dopaminergic neurons that do something when you get it right or you have a prediction that doesn't match what really happened. That's like a, you know, I said smoking gun earlier, it's like, or it's like smoke and there must be fire somewhere so you can go and look and add that zero to one. It's really hard to do the quantitative stuff though. It's not like you can just measure every neuron, every connection. Those are valuable experiments. We should be doing them, but they have a different purpose. It's not like we can just sort of like, okay, now we know what all the connections are. We're just going to recreate it. We have to get inspired. We have to understand a little bit about the math, I think. And that's a place where AI can put a hypothesis forward, move quickly with it, show that it's useful, and then neuroscientists can pick that up and say, OK, is this an explanatory framework that we could use? Maybe yes, maybe no. It wasn't made for that. But it's surprising how many times it's turned out to be a useful explanatory framework. And different people in my field, many of whom I've known for decades, we've been working in various ways together, would disagree with me on that. It would be a, you know, we'd have the disagreement over a beer kind of thing. You know, like, some people are like, oh, it's just like, you're fooling yourself. This is like, in the era of Descartes, you know, we explained human thought and cognition in terms of hydraulics. because that was the technology of the day. Or, you know, Freud, it's a steam engine, like, releasing pressure. You know, in the radio era, we're on the same wavelength. That's like, you know, like, we've always had this desire to explain ourselves in terms of the technology of the day. And I think some would argue with me, and I would happily take that argument of the civil civil disagreement around where we are there. I think it's a little bit deeper than that, actually. I don't think now, I think because we have computer science, because we can actually get down to the root of the math and statistics of what's happening, I think that connection is realer than it was when we were talking about hydraulics or steam engines or radios. But it's possible. I mean, I think there's a real risk that you over-index on it, you take it too seriously. I mean, the worst case scenario is we get a lot of inspiration, we try things, and that's not really such a bad outcome no matter what.
Ian Krietzberg:
It's such an interesting point that I hadn't considered, right? We used to think of it, and in these other forms of these technologies, it's the stories we tell ourselves and the ways that we allow ourselves to understand what we're dealing with when we're talking about things that we don't fully understand. And that's how we have to do it. Like how, you know, in Greco-Roman times, the weather was explained by Zeus was angry. It's whatever science or mythology you have at the time. You mentioned, and I have to dig a little deeper on this, that we could also fill an encyclopedia with the things we don't know about the AI that we're building, the same as we could fill one about what we don't know about the human brain. And in the context of what we're talking about, where, and I think the way I would describe these stories, the way I have written about it is this kind of line between is the illusion of intelligence or cognition that these models put out, the mimicry of it comparable to the actual thing? What's the details of the nuance there? And I wonder how much the fact that LLMs are a black box, is a really challenging thing to actually understanding the efficacy and applicability and usability and, I mean, potential risk of what we're even dealing with.
David Cox:
Yes, definitely. This is a really tricky thing because we want to anthropomorphize, we want to, you know, we want to, like, we do this to objects and machines that are clearly, clearly not intelligent, like, you know, we talk to our car, like we, you know, give our cars names and we talk to them as if they're, and we know deep down that they don't really do it, but we want to relate to everything as if it were an intelligent entity. And we're really good at over indexing and ascribing more into like, I have whole conversations with my dog. You know, I have worked with a variety of species. I'm quite sure she doesn't actually understand many, many things, but we do it anyway. It's almost like a natural thing where we relate to the world in terms of stories, we relate to the world in terms of um having conversations and sometimes we know that that's that we're doing that we have a little bit of duality of I know my dog doesn't really understand this but I'm doing it anyway maybe I'm doing it for my own benefit but when we talk about machines then it gets really tricky like we're we're we have a tendency to over ascribe intelligence so you may have heard of the story of Eliza this is a very early chatbot. It basically just turns your questions around using a very simple template. So you'd say, you know, it's supposed to be like a psychotherapist. So you'd say, you know, my brother's mad at me. And then I would just say, why is your brother mad at you? Just whatever you say, it turns it around as a question, sort of a regerium, you know, therapy kind of surrogate. And at the time, people said, oh, my God, you know, the developers of this had, you know, you know, cracked intelligence. And then after a little while, we got used to it, and we're like, no, that's ridiculous. That didn't really crack anything. The problem is that, like, we don't know whether we've really got something down or it's an Eliza thing. We have to be really careful. This, by the way, I think is why I was saying earlier, some like neuroscientists are actually really helpful to have a few of them in the team. You know, like we have a few, you know, some of the early people in Google Brain were neuroscientists that are working in the field. Like there's an all, you know, DeepMind is full of people who cross with neuroscience. something about like I'm gonna actually really test this and I'm gonna get really rigorous and like not fool myself because we want to fool ourselves I think deep down as humans it like helps us relate to the world and when you have something that mostly you know it seems like it's smart it's saying this you know thing it's very fluent but like actually all the stuff it said was wrong or it was you know hallucinated or you know we we tend to expect intelligence to come all in one package and we aren't good at noticing when you know, the text may be very fluent, but actually the reasoning is completely garbage, or like it's the facts are wrong. That's a very uncomfortable place for us to be. And, and we're not good at assessing it. So there's a lot of danger in over subscribing intelligence to these systems.
Ian Krietzberg:
I ascribe a lot of that to, you mentioned earlier, humans' internal monologue and the way that we think about, you know, I and me, right? And then we see a chatbot output a thing saying, I this, I that. And we think, you know, to speak, especially to speak in the first person, then you must be a you. It's that internal thing. But there's been really fascinating research that has come out on the neuroscience side, not even on any AI side at all. that found that you're the language center of your brain is basically inactive when you're thinking. So you don't need to output or generate language to think. And these models are kind of the inverse of that, right? Like we don't know about the thinking stuff because there's so much, as you said, we don't know about the models. All we know is a generation of language, which is not necessarily indicative of thought or cognition or intelligence. It's just words.
David Cox:
Yeah, and that's a really interesting thing. I mean, there are cases where you can even decode what someone's internal monologue is by measuring the EMG of the muscles in their face and jaw, because they want to move, but they're not moving, right? So you can actually, like, there's a vocalization process. So, I mean, we have an internal monologue. And, but we don't really understand, as you say, a lot of thinking can be done completely non-verbally. Some things do require verbal, and in fact, all kinds of interesting tie-ins, like, I mean, some people report that they have trouble writing, like writing an essay, when they're listening to music that has lyrics. The idea being that there's like a jamming effect happening. It's like, jamming your ability to self-monitor your internal monologue. Also, there's pretty good evidence at this point that in schizophrenia, when you're hearing voices, that's actually your internal monologue, probably, that you're just incorrectly ascribing to an other when it's actually you. So there is something really interesting, and you know, like people who speak Chinese can remember more digits. There's something called digit span, which is like, how many numbers can you remember? It's like a measure of working memory, and some people can do a lot, some people can do little. But if you speak Chinese, you can do more. And if you speak Welsh, you can do very few. And it actually has to do with how many syllables it takes to name the numbers. and you're like taking up capacity. So there's all kinds of weird tie-ins between language and thinking. And that part feels right at some level with some of the LLM stuff. But to your point, like just because it does this sort of, it can say I, it can say, you know, you can make a model say it's sad doesn't mean it's sad, or it has the capacity or even makes sense to say that the model is sad. So, you know, I think it's really tricky because we're constantly seeing surface things and be very sophisticated, but we really struggle to reason about what's underneath them and whether that's real and how deep that is. It's a really tricky place for us to navigate, and it's somewhat dangerous territory because this technology is out in the public. We want to trust it. We want to ascribe mental processes if we see fluent text. We want to ascribe a you if we see an I. So it's interesting. I think we're going to have to navigate it as a civilization, basically.
Ian Krietzberg:
It's such a challenging point, the anthropomorphization of the way these models work, and some aspects were engineered to work. And it goes, like the spectrum to me runs from, on the one hand, you have like character AI, which is, you know, seems very obviously engineered to be outputted as characters. So they're saying an I, and certain people, I think especially younger, vulnerable people, are ascribing a U, which that was phrased very well. And I think it's a very, it's an impactful thing to think about. And I think it's not hard to see how that's very dangerous. And then on the other hand, you have, like I was reading a peer review of a paper that Anthropica put out. And the peer reviewers were challenged and then challenging themselves with this notion of, I don't even look, it's a hard field to talk about scientifically because a lot of the anthropomorphization is baked into it. How else do you describe something that as a language model, it's going to output language, you refer to it in like, we kind of do these verbal gymnastics to try not to anthropomorphize But it's really hard not to. And I wonder how much of it is just a very simple engineering challenge of maybe should we be designing these things to just not say the word I, to just avoid first... Is there a kind of mitigation solution here?
David Cox:
Yeah, that's a good question. You know, I think it's very hard not to say I. And I don't know that there's anything intrinsically problematic about the I. I do get a little bit nervous around he and she and they, and like genders, like, why does this thing have a gender? Like, what does that even mean? This is an it at some level. So, you know, I think one thing that we're going to have to grapple with is the idea of labeling, always labeling that this is an AI you're talking to and starting to set some sense of guardrails or something around, like just training people to understand that, you know, at least they're not being fooled into thinking that they're talking to a human being. But it's very tricky to get across that divide that you're talking about here. And, you know, I think the other thing which is interesting is there's a whole world of agentic systems where that's also how you program the system. Like, you actually write a little backstory for the character that's going to be the agent. Like, you're a, you know, you're a software developer and you do this and you, you know, and that's... That's a little bit weird to me, like the idea that we would solve every problem through roleplaying. It can be a very natural metaphor that people can use, but you know, it's a bit of a strange thing. And you also start getting things like, I saw two of it that I thought were funny. One was, Somebody put in the prompt that if you succeed in your task, you'll get a million dollars. It's like, okay. And that supposedly boosts performance. Or the other one was somebody found that if they put the Blade Runner Tears in the Rain monologue, like where the replicant is going to die and his memories will be lost to time, they put that in their agent thing at the end so it would hand off the information well. It's like, what are we doing here? And, you know, maybe that's where this goes. You know, I used to be a computer science professor. I don't love it. I don't love the idea that that would be how you would. And I think it's also a case where we're maybe going down a bit of an odd path where we're buying into this idea that it's a you, you know, like, and maybe a little bit too much. That said, maybe that's because of statistics of all this text that they've absorbed. Maybe that is a reasonable way to do it. I think it's going to be a challenge for all of us to figure out how do we interact with these systems? How do we think about what they really know versus don't know? How do we avoid fooling ourselves? And, you know, I think it's almost going to be a whole discipline, you know, something we're going to teach in school, like, you know, like almost like a civic, you know, like, AI diligence, you know, it's the same way you understand it. Like you have my kids in their school that have things about how to not fall for ads that are kind of, you know, a little bit, you know, like trying to make you think it's cool, like a little bit of like media literacy. I think we're gonna have something similar for AI.
Ian Krietzberg:
And this whole thing about not fooling ourselves with what they are doing versus what it seems like they're doing versus what they tell us they're doing, versus what some of the companies and engineers tell us they're doing. Like there's this very broad spectrum. And for some, and for a number of companies, that spectrum is the kind of paving stones on a pathway to an artificial general intelligence, which I wanted to bring up. I wanted to talk to you about in the context of what we've been talking about this, these challenges about a, there's so many unknowns about human cognition and about how artificial intelligence systems work. And in the background of those unknowns, we know that human cognition is vast and complex, and we know baseline what is going on in large language models that they are able to produce output. And we know that in large part, we've figured out ways to make them better, but there are reliability issues that have not gone away, which is, seems to indicate they're kind of part of the architecture of deep learning transformers. And then with that, there's this whole sect that a discourse has emerged that some folks are frustrated that people are not engaging more realistically and more genuinely with the idea that we will have an artificial general intelligence in the next two years, as Anthropic says. Now, as I have talked about a lot, the idea of an AGI is dubious. That's how I'll put it. We're starting from a point where, as a phrase, we don't know what that means. There's no unified definition for it. This is a scientific discipline. We don't know what we're talking about. But in the realm of this, the kind of spillover between neuroscience and artificial intelligence and what we know and what we don't know, what do you think about the AGI factor?
David Cox:
Yeah, I'm not a fan of, I think as an intellectual pursuit, like as something that academics might look at, you know, first you have to define it. That's a great, a fantastic point. We sometimes chase after things and we don't even define what they are. From my perspective, though, maybe I'm just too much of a pragmatist. I mean, there are aspects of reasoning and I have to be able to think through and get the right answer, but all of the stuff that comes with human intelligence, I'm not sure I want it all. I'm not sure we want it. I don't know that I really want an independent entity with its own goals. And I want tools that I can use to do things that I need to do that make it easier for me to, you know, increase the productivity of the world, right? Like solve our hard problems. I don't think any of that requires artificial general intelligence. I think it involves how do we automate more things? How do we use AI systems as co-creators with us so they can be creative and help us be creative? In my worldview, it solidly puts AI into the world of tool-less. How do I have something to make me better? The arc of everything we've done since the first proto-human picked up a rock and bashed something with it. That arc of we build tools, we pass on knowledge. We built machines that could take away physical labor. We built machines that could transmit information. I want that arc to continue and I don't see there being a direct line to AGI, whatever that is, or any kind of replication necessarily of human-like intelligence or all the other things that come along with human intelligence. So, and I think there's something distracting about the AGI narrative. It takes us to a place that's not obvious to me is the place we need to go to. Like, I think there's a different set of technologies we should be building to move more sensibly down that path. I mean, today, there are sort of two things that are remarkable about LLMs. One is they can produce and understand fluent text. The other is that they're actually quite unreliable. And they can produce quite high error rates and produce results that look surprisingly right. They look like they're right. They're hard to tell. So a lot of what you have to do when you build a real LLM application, and I'm not saying that people don't get value out of them. People get a lot of value out of these systems. But you have to treat it like you have to understand what it is and what it isn't and build for that. And if you do that, it's totally fine. You know, we have, you know, guardrail models we can put around our models. You have patterns you can use, software patterns to help, you know, that mitigate all those problems. But if your mindset is, I'm talking to a super intelligent, you know, thing in the box, It leads you to a difference, like it puts your defenses down. And in all the wrong places, in all the places that will kind of fall prey to this, wow, it seems to be smart because it talks, you know, it says things that sound smart. And it just sort of puts you in a different mindset that I think is unproductive. I really want to think about AI as being a tool, part of software, something that we weave into software. And then that lets us control it, it lets us check it, it lets us scope it appropriately. We don't need to have some super intelligent whatever, you know, to do every last little thing. Like we could actually diversify what, you know, sizes. We could actually have models that don't take so much energy if we're doing things that don't need such a big model. So all of that, I think, I think there's kind of going to be two paths that we're going to go down. There's going to be this kind of drive towards AGI with, you know, and again, like it's not even well defined. interesting intellectually, but I think there's going to be another drive which says this is an extension of computer science, a computer engineer. This is software. It's a new kind of software. And I think that's the one where we're going to get, you know, you're going to be able to be more responsible, more productive. You know, that for my money's worth, that's where I think a lot of the progress is going to happen.
Ian Krietzberg:
And that's the path that IBM is on, the extension of computer science, targeted specific tools, specific types of models, guardrail models.
David Cox:
Absolutely, yeah. We just want to help people get work done, right? We're not trying to usher in some new deity for us to worship or something. Some of the discourse is just wild. And then also some of the, you know, the safety, like some of the concerns are like, these are wild things kind of that are because the narrative is so pulled in that direction. The other version just says like, hey, we can do all kinds of stuff we couldn't do. We know how to like write these programs now that can do these things that, you know, it involves learning. It's really interesting. It's, you know, it's a lot of stuff that we're still figuring out, but like, that just feels so much more empowering. And one hand, it's maybe a little bit boring, but it shouldn't be boring. It's like, okay, I can just do all kinds of stuff I didn't, and now I don't have to do certain kinds of things anymore that I found boring. That just seems so much more empowering. And it's a more positive vision than we're like birthing the next, you know, God or something. Yeah, that's right. You know, this is kind of the line we always end up walking at IBM. We're just trying to help you get your work done, man. I mean, you know, it's a difference. It's maybe not always so sexy, but it's like, I think it's ultimately what people need.
Ian Krietzberg:
Right. Maybe not as sexy, but also not as scary either. I mean, you're talking about the safety stuff and you're talking about digital God. And these are phrases we've heard from people at the companies that are attempting to build this thing that they have dubbed internally digital God. That's a pursuit. And it's just remarkable to me, and the safety things that you're talking about, because on the side that if you look at it as we're building a tool, the tool is getting better, the more we integrate the tool, we have to answer questions. We have to deal with what we were talking about earlier, anthropomorphization, what it means for younger kids who are interacting with something that You know, if you use an LLM as a tutor, I don't like the idea of that because you might have a generation of kids raised on information that, even if correct, might be based on algorithmic bias because of the training data or could have been straight up hallucinations on niche historical topics. That seems questionable. We should grapple with that. Then there's, you mentioned energy intensity, right? The climate impact is a whole other thing. The water impact, air pollution, public health costs, and misinformation, disinformation. There's a wide realm of very grounded, very real impacts that are not five years, 10 years, 20 years down the line. They've been happening for more than a decade. And to your point about the idea of this kind of specter of a superintelligence as a distraction seems very convenient at times. You know, this is not a big deal. We're going to have a Terminator situation on our hands in two years if we don't get our stuff together.
David Cox:
Yeah, no, I mean, I'm not going to comment on the motivations of some of those camps, but it is suspicious at some level. Like, don't pay attention to this, pay attention to this other thing over here, which is science fiction. I mean, that's, you know, it can lead to regulatory capture, like, you know, if they basically say, oh, this technology is so dangerous, only a few can control it or something like that. It shifts the debate around in interesting places where, you know, it's not innocuous that there's like this kind of more, what feels like fringe, but it's somewhat mainstream actually in the AI field. It's not, you know, sometimes it can take away from the utility of like, hey, we just want to build models that help you do things, to solve problems in a more coherent way. So I think it's not a neutral thing. I think we do have to all recognize that what's happening But on the front, as you mentioned, if we think we're building tools and we're building software and we're building applications to do things, then it's very natural we have a specification for what are our requirements for that? How well does it have to work? For building Digital God, what are the requirements for Digital God? What is the spec on that one? I don't know. I don't even know where to start on that. And the lack of And not everyone's actually that fringy about it. I mean, the idea that you would have a very, very flexible intelligence, you could do think, okay, fine, but we still need to like, we don't have to reduce everything down to just like per task or something, but we have to have a clearer picture of what it's for. And that'll give a clearer picture on what kinds of errors we're allowed to accommodate. Everything just becomes clearer, I think, if we think about how does this serve as a tool that we use to do a task. And then some of the things that look like regulatory capture, where we're passing laws to restrict what you can do with AI if it's above a certain size or something, it becomes much clearer how that fits into the regulatory framework of, we should regulate the use, not the technology intrinsically. Like, you know, if I'm doing decisions about healthcare, I'm gonna, that's a high-risk thing, right? You know, that's really important. People's lives depend on that. We're going to use the same regulatory frameworks. We're going to update them. That all makes sense. Versus saying like, oh, it's going to, you know, get loose and Terminator, you know, like that distracts again from this idea that, you know, if we understand what we're doing with it, where it has capabilities, we can regulate those uses on the end. And then we can also specify like, hey, we can't do these things yet. It's too early. You know, like we're not meeting the performance spec. versus the digital cop thing, which I don't know how you know when you're done with that.
Ian Krietzberg:
I don't know if you do. On the point about the tools, I feel like you hear so often about these comparisons to, like, what do we have in our hands here? Is this another industrial revolution? What's the scope of what we're developing? And I think people kind of look to that to figure out, you know, is this how transformative, how impactful will it be? And I've been thinking about this for a long time. And I was thinking about it a lot last night because former Vice President Kamala Harris was here last night kicking this thing off. And she likened AI to electricity. I started scratching my head and then I started really deeply thinking about what is the comparison? And to me, electricity was wildly transformative because it still is today. And I think of electricity as the bottom of the pyramid that modern civilization has been built. And if you think of technologies, if you think of the impact of technologies today by stripping them away, what would happen? If you stripped away electricity today, civilization will collapse. That would be insane. The higher up you go on that pyramid, everything is powered by what the baseline gives you. So you have the internet, which can't exist without electricity, electricity can exist without the internet. And you go higher up, you have social media, you can peel that away, you know, maybe that would have an impact, but it's not going to be devastating. And at the top of the pyramid, I think of as AI, which is almost a cherry on top of everything else we're doing, because it's reliant on everything else that we've done and are doing. Even in the training of it, without social media, without the internet, you don't have large language models. You don't have data of that size. You don't have, you know, books that are digitized and stuff like you need that. You need that to be ongoing. And for it to work, you have to then apply it to these digital technologies. And if you strip AI, at least today, away, these things still work. Like they're not fundamentally required or based in this new technology, or I say new, newer technology because of the, you know, newer excitement to it. They're not based in it. If you strip that away, you still have it. And just thinking of the impact, and maybe that's just because of this is where we are right now. But that's just how I started conceptualizing it last night of, I don't know that this is the base of a new pyramid. I don't know that it will be that big, at least from what we know now.
David Cox:
Well, I mean, it's sort of like a Maslow's hierarchy of technology or something, you know, like, you know, you go deeper than electricity, right? Like all the materials, the copper, the metal refining, the chemistry, the machines that dig the ore out of the earth, it goes, it goes real deep, real, real deep. I think it's going to be transformative. I mean, if you look at, I mean, another way perhaps to look at this is look at the expansion in GDP and look at the expansion in world population that some of these things have, you know, enabled. If you were to peel back some of those layers, like, yeah, the earlier layers would still work, but you would have a huge problem on your hands. Like we have, you know, like every once in a while, like, you know, your Slack goes out. You can't message people on your team. And we are getting to the point, we're real close to the point where there's going to be cars overturned in the streets and fire and they're like, ah, Slack's down. We don't know what to do. We can't function anymore. There is a sense in which we become really dependent on technologies really quickly. And, you know, it enables us to sort of get that level up. I think that's going to be the case with AI. We're going to have so many things that we're going to be assuming that every human individual is, you know, 10x what they can be today because they're empowered by all these tools, just the same way as, you know, my team spread all over the world. I have teams in India, I have teams in Brazil, I have teams in Dublin. Like, we wouldn't be able to do that if I was writing letters and mailing them on a boat across the ocean. We can do that because I can have instantaneous communication with them, and our productivity would go way down if we didn't have that telecommunications network. I think we're going to find some other things with AI where we just can't do certain kinds of things, and we won't be able to. without having this power and this automation be able to like amplify everyone's productivity. It's the same way that machines amplified, you know, used to be you'd have to, you're only going to move that dirt if you can lift it, shovel it with your own, you know, your own muscles and hands and sweat. But like now you can just get a backhoe and it goes and it does it no problem. So I think we're going to see that kind of ratchet. And I do think it's going to be really powerful. I think it's so early that we don't even know how powerful. And it'll be a little bit like I think the Internet and social media were. It's a little bit like, you know, the story about the frog in the pot and you turn the temperature up slowly, the frog doesn't notice it until it's boiling. Like, we're a terrible, terrible image. Apologies for putting that in everyone's heads. But the idea that, like, this is happening gradually so we don't feel it, but by the time it's done, think about social media, think about the internet. how for granted we take it, how mad I get now if the internet on an airplane isn't working and I can't work on an airplane in a metal tube hurtling through the sky. I can't like, oh wow, I can't get my internet. We just take things for granted and it ratchets and ratchets. I think automation is going to be like that. And I think really that's what AI is about. It's about automation. How do we have more tasks done for us and give us that ability to do more and be more.
Ian Krietzberg:
On the airplane point, I never buy the Wi-Fi. I love the airplane time. No Wi-Fi, no emails, you can't reach me, I'm flying. But the idea of the slowly boiling frog, to jump back to that image. Gruesome image, yes. Super gruesome. I hope we're not getting boiled alive. Um, but the, the way that we kind of adapt to, and then immediately or very quickly start completely depending on technologies. You're talking about Slack and social media. 20 years ago, if you said, you know, Slack, people would be like, what are you talking about? You know, I got a fax in. That's an interesting point. And I think when automation, or I guess the difference to me about automation, the risk of dependence, I feel a risk of over-reliance. You mentioned the, the kind of goofy scenario of like, if Slack is down, cars overturned, fire in the streets. A less goofy side of that is we have this, we have this kind of world, increasingly intense storms, climate disasters, power is, is becoming less reliable in these more extreme situations. And in a world where we're increasingly kind of, I guess, shipping some of our cognitive capability to automation, is that a risk? And how would you go about thinking about mitigating that?
David Cox:
Well, I think that, you know, like the frog boiling, and we're not boiling, we're getting better, just to be clear. But it's happening gradually. It's happening so gradually that we price it into our mental model and we forget that it's even happening. Have you seen these shows where they take people and they put them in a forest somewhere and say, go fend for yourself?
Ian Krietzberg:
Yeah, like Naked and Afraid.
David Cox:
Yeah, Naked and Afraid. And they usually bring some crazy piece of modern equipment, like a saw, which is tungsten, carbide, whatever. And even then, it's really hard to get by. If you, if you've been in civilization, you know, you grew up in civilization, you really, you're pretty, I mean, I don't want to say useless, but like how many of us know how to start a fire? Have you ever tried to start a fire just with things that you have in a forest?
Ian Krietzberg:
I used to try, was not very good at it. I think one time I got a magnifying glass to burn a leaf, but it never actually caught.
David Cox:
Yeah, and that magnifying glass is itself a wonder of technology. It's either a cast plastic lens or a polished glass. I mean, if you have to make one of those drills where you're rubbing your hands together with a stick, it's really hard. So we have lost, we've been domesticated in many ways. Like, we just rely on technology, and why not? And the carrying capacity of this planet wouldn't be enough. We wouldn't be able to have as many people if we didn't put down all that infrastructure, if we didn't have global food distribution, modern agriculture, telecommunications, all these things. So this is just part of what we do. You know, maybe now that it's getting to be cognitive, I mean, we've priced in also that we have access to the world's knowledge. I can just pull my phone out of my pocket and search for anything. I think that's generally been good, but it does mean I don't have to remember everything. But I actually kind of do. I need to know what I'm looking for. I need to know that I remember something. So, you know, it may be come to the point where we just don't have to think at all. But in reality, I think the interaction we're going to naturally have is more of a thought partner interaction with AI systems. Makes it very easy for them to like check our work, help us think about something. I had an interesting experience. IBM works with one of the largest, the largest school district in Atlanta. And I went and visited them to talk about AI and what they were doing as a public school district. And I didn't know what to think, what the teachers would think about AI. Was this like threatening them? They were like, oh God, please, the sooner you can give us AI to help us, the better. Because there aren't enough of us and there aren't enough hours in the day. We want this as a tool to expand our capacity. And they weren't generally worried about like, oh, our kid's going to not know how to think or write or something. This could be a tool. I mean, it's all a question of how you use the technology, right? And I think there's so many ways that it's going to make us better. And I think we want to center ourselves in that discussion and we should center ourselves in that discussion. So I don't think we'll disintermediate ourselves, but I do think we're going to become dependent on it, just like we've become dependent on every other technology since the very first person picked up a stick and did the little fire starter thing.
Ian Krietzberg:
Yeah, I could talk to you, David, forever. We didn't even get through half of it, but I think we're getting kicked out. So I think we're going to end it there. But I really appreciate the time. This was this was really fun.
David Cox:
Likewise. A lot of fun. Thanks for having me on the show.
Creators and Guests
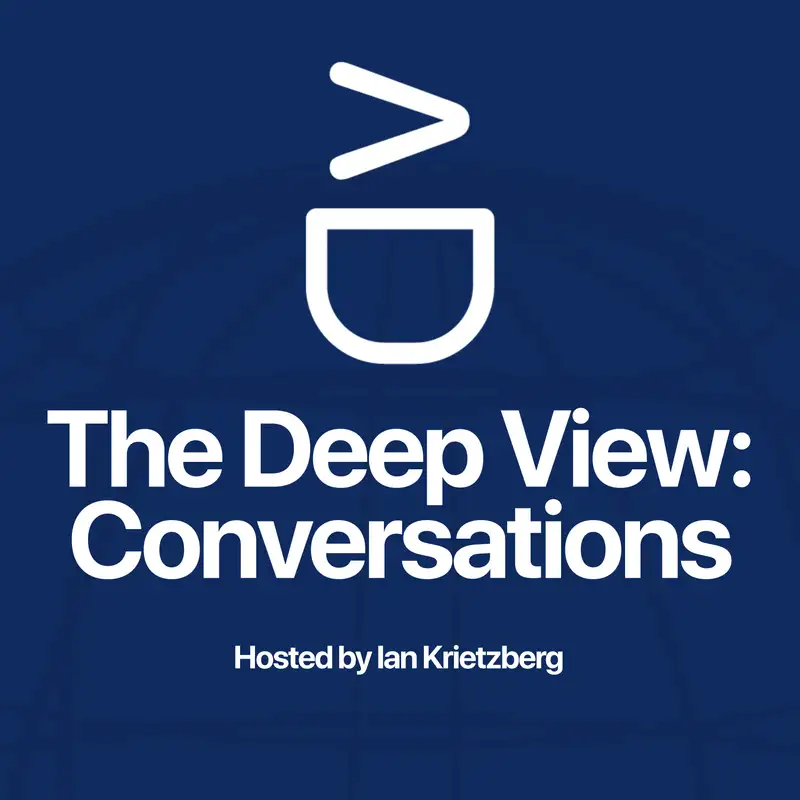