#14: The five taboos that Silicon Valley broke - Igor Jablokov
Ian Krietzberg:
Hey everybody, welcome back to the deep view conversations. Um, well, I'm going to, I'm going to start things off a little bit differently than I normally do with a question. Have you ever heard of Siri? What about Amazon Alexa? I'm going to assume that pretty much all of you have said yes, and are now scratching your heads, you know, asking Ian, where have you been all this time? The reason I'm posing those questions is that today I'm speaking to one of the, one of the people behind the early inceptions. of those technologies, of those applications. My guest is Igor Jablokov. He today is the founder and CEO of Pryon, an enterprise AI company. Back in the day, he served as a program director at IBM, leading the team that created the early iteration of IBM Watson. His first startup was acquired by Amazon and evolved into Amazon Alexa, which is the name of his older sister. What we're talking about today, as things always are, it's varied and we go in all sorts of places. What we're talking about is the state of the field of AI today and how it's changed and evolved and grown in ways both positive and negative over the past few decades and where it might be headed. Igor, thanks so much for being here. I'm so excited to have you on. Yeah, thanks for having me. Of course. So just before we jumped on and hit record here, we were talking about the punk rock days of AI and how things have changed so dramatically. And this was part of the reason that I wanted to have you on and hear from you about those exact punk rock days that you were mentioning. You were a program director at IBM and leading the team that developed the precursor to Watson, which has, you know, since grown and evolved in a time before generative AI was kind of everywhere. And I just love to hear about that time and what it was like and how you were approaching what you were doing and why you were doing it.
Igor Jablokov:
Well, I mean, a lot of these things that we talk about actually did exist. They just existed under different names, right? So I'll give you a perfect example. You know, what you know as an AI assistant, we were trying to call a multimodal portal. What you know is cloud computing, right? We used to call client-server or hosted. You know, what you call generative, we would have called adaptive, right? What you call a neural network, we would have called a lattice, right? So there were all these you know, when you would inspect any of the, you know, architectural diagrams and things of that sort, you would be able to see the DNA of the things that people take for granted and think are 21st century, you know, vestiges, but you would find them in the past in the same way that, look, you know, we're, you know, we're DNA, you know, that share, you know, you know, material with other plant and animal life as well. And that's because that's where we came from.
Ian Krietzberg:
Yeah. No, that's really interesting that the kind of different names and how things have evolved and the focus has shifted. Right now, the focus is very much on large language models and deep learning. And what was the focus then? Was that stuff kind of in the periphery or was that also something that was central?
Igor Jablokov:
Yeah, that's actually a great question because there's a term out there that kind of horrifies me and it basically says if software is eating the world, AI is its teeth. AI was supposed to be the heart. And so, you know, the direct answer to your question is the fact that many of us were attracted to the field, you know, to solve three big problems. And the first problem was accessibility. How do you make user experiences that had a wider aperture and promoted the use of computing to populations that wouldn't otherwise have access? And who would that be? Children, senior citizens, handicapped people and things of that sort. My chief scientist at the time was blind. So that was the first reason many of us were attracted to the field. The second reason, obvious enough, is safety reasons, right? So instead of texting while driving, you can just talk and some of the precursors to things like Apple CarPlay or Android Auto or having Google Maps in your cars and things of that sort were actually technologies that we were bringing to bear with IBM embedded via voice with WebSphere voice server. We were behind the scenes on GM OnStar as an example. And so that's a second expression of something that attracted many of us OGs. And the third thing that attracted us was bridging cultural divides with machine translation. You know, that was fantastical to all of us so that we can relate to one another, even, you know, if we come from different cultures, you know, different nation states and things of that sort. So those are the big three use cases. It wasn't the stuff that you're hearing nowadays about these use cases.
Ian Krietzberg:
The approach feels different, right? The kind of targeted use cases that you're talking about, the idea of enhanced accessibility, translation, the kind of safety things that we can do with self-driving cars, obviously these problems turned out to be really, really complicated to a degree. And I think we've come a long way on machine translation. Self-driving is getting somewhere. But it feels as though that kind of like what you just said, that's not really the focus that seems on kind of top of mind when we talk about the field and the industry today.
Igor Jablokov:
Well, I mean, you know, it's it's that's because, you know, I was speaking to somebody yesterday evening. All of us that got attracted to the technology field, we thought we would have careers in in the back room. Right. You know, as as I.T. people and things of that sort, just tending to servers, tending to data centers and things of that sort. Now, because the field is permeating everything you can think of and changing a myriad of industries, a myriad of jobs, AI in great power competition, things of that sort, we're literally at nation-state level on the world stage. you know, all of us that expected to start careers, you know, you know, sitting behind the scenes, are now thrust, you know, into the limelight, if you will, in terms of, hey, what do these technologies mean for, you know, a future American way of life and our allies? And then, you know, you know, how do we create plenty out of it? How do we disrupt criminal cartels that are using these technologies to sow distrust or do other nefarious deeds, especially from a cybersecurity standpoint as well? And so that's a little bit what's different. Now, why do these technologies exist and it feels like everything's just rapidly accelerated? Well, because there was essentially five taboos that were broken you know, by the industry that were radioactive and held many of us back because they posed ethical quandaries. And so once the industry essentially breached those five taboos, you now have the things that you have that in some ways, you know, should have not existed. What were those five taboos? Yeah, so I mean the first one that Musk is trying to correct is you can't have a non-profit become a for-profit, right? So that baited resources that would otherwise not be obtainable for any commercial, you know, venture because they're able to take a write-down on that spare compute, you know, that existed in there. And as a result, they were able to do the second thing, which is like, hey, we have all this excess compute. What do we do about it? Well, the second thing that was not permissible by any of our scientists, engineers, and things of that sort, was to trawl the internet and download all of it. without care to copyrights. And of course, you know, recently we've seen the case of Thompson Reuters and Westlaw and things of that sort, you know, essentially challenge, you know, the presumption of fair use because the entity on the other side, you know, was using it to create a replacement service, you know, for them as well. And that didn't trigger fair use. So that was the second thing. We didn't take copyrighted content off people's website. The third is the alignment problem, you know, which is, you know, any of us in the field, right, if you're a computer engineer, computer science mathematician, we got paid for omitting ones and zeros. That's what we did for a living, right? If you go to McDonald's, you know what order you're getting? You're putting in, you're getting a hamburger and a french fries. You know what you're getting from one of us? A one or a zero, a deterministic outcome so that, you know, if you press the button, the light goes on, you press the button, the light goes off. But they had this alignment problem. It started saying things that weren't true and things like that. And yet they still released it. Now, the reason why they released it had nothing to do with with the technology. It was just DeepMind versus OpenAI. They're just on a bug hunt, you know, trying to jump in front of each other in some ways. It's a very human story. It has nothing to do with technology in terms of, you know, trying to keep up with the Joneses, if you will. So that's the third problem. The fourth problem is, think about any one of you that get in front of a Google search. You're typing in your query. At times, it could be a sensitive medical query and things of that sort. And there's a presumption that there's an encrypted connection between you and the brand. And that's for your eyes only as well. Now, you know that they're going to be reading that in your profile to try shove ads in your faces as well. But your presumption is that it's a mechanical process that's safeguarded by encryption. Well, that's not how a lot of these LLMs work. It's RLHF, that's fancy language, reinforcement learning with human feedback, that there could be humans in the loop that are essentially seeing all these things. And in many cases, they're sitting in offshore contact centers. You know, there's not an attorney now that doesn't have to write a breach notice for employee information or client information that got spilled unintentionally because some worker somewhere wanted to use, you know, one of these LLMs by uploading a sensitive document or some sensitive employee information to get help. That's the fourth problem. And the fifth is the most nefarious one. The most nefarious one. Because when you talk a big game that AGI is coming, Some people start thinking that there's divine inspiration and something magical that's about to happen. We are going to birth life, synthetic life. As a result, And then when you take that and compound it with the fact that they're going to conferences and saying, hey, you should start using this for mental health support. Well, guess what ends up happening? People think it's divine and all-knowing like a burning bush. They're being told to use it for mental health support. When this thing says some unfortunate things to you, people are starting to commit suicide based on the output of these things, right? I mean, we're already seeing this, you know, both adults and teenagers and things of that sort. And that's where things, you know, start getting spicy. And that wasn't their original intentions of the field, right? Think of whenever you create technologies, you know, it's like you're creating this hammer and you're on the presumption that Jimmy Carter is going to put it in his hand and he's going to build habitat for humanity for you. Sometimes you can't foresee that Ted Bundy is going to be hurting people over the head with it.
Ian Krietzberg:
Those taboos kind of, it sums up very well, you know, what's happened and functioning as an enabler. You know, you talked about how it's kind of in the AI is in the limelight right now in a way that it wasn't 20 years ago. Yeah. And breaking those taboos, the kind of move fast, break things mentality that Silicon Valley kind of infused. into the field has kind of pushed it into the limelight in a lot of ways. And would you prefer it in the kind of backroom to the limelight that it is now? Because as much as those taboos are broken, you also have more attention on the field. It's easier to get funding and advance some of these kind of altruistic purposes that maybe were the inception of the work here.
Igor Jablokov:
Well, I mean, those of us that are attuned to quantum processes know that the past, present, and future are simultaneously happening. And as a result, there's a reason why this stuff is getting uncorked, and we don't know what the reason for it yet. So you have to deal with the cards as they are. Look, yes, things move faster, but it also presents an opportunity for some of us that want to do the adulting of AI. You know, somebody has to keep the power plants running. Somebody has to keep, you know, the academic institutions omitting facts, right, and processing facts. You know, somebody has to keep the hospitals running, the water treatment plants, the airports, the ports, and things of that sort. And so, you know, while everybody else, you know, wants to, you know, shove ads in your faces or, you know, put more videos in children's eyes or turn our respective heads into Barney the Purple Dinosaur, you know, with Gen AI, you know, there's going to be a separate constructs that are going to be AI native companies that are wholly focused on B2B. That's it. And while everybody else is pushing the state of the art and obviously figuring out, well, how does this help creativity? How does it help imaginations? How does it help, you know, creating these interfaces that are doing all these things for consumers? There's going to be a whole parallel universe of folks trying to figure out how to aid in productivity and turn an American worker into a far more capable being. I don't think I don't think people are going to be losing as many of their jobs as everybody was worried about. Because when you looked at the dawn of the computer age, the information age, it was just the middle managers that couldn't figure out how to adopt computers as part of their everyday workflows. Same thing here as we're moving from the information to the intelligence age. I think, and I'm sure your listeners have heard this before, you know, attorneys aren't going to go out of business. It's the attorneys that don't know how to use AI that are going to go out of business, right? Physicians won't go out of business. It's just the ones that don't know how to use AI that will go out of business. Any more than a surgeon, you know, that didn't get trained on certain minimally invasive methods is the way that you have to think about it.
Ian Krietzberg:
What would you liken this technology to? Because we hear a lot of things and we hear a lot of kind of dramatic hyped things. This has been compared to the printing press. This has been compared to another industrial revolution in terms of scale. Is this kind of just another evolution of the internet? Where does it fall on that spectrum?
Igor Jablokov:
Yeah, it probably, AI is probably a big deal, right? If we are creating synthetic life, it is a big deal. But, you know, also, you know, with us discovering DNA and things of that sort, you know, everything that's coming, you know, in life sciences is going to be a similar big deal because, you know, we only talk about the negatives in AI and that's all we've really covered up to this point. But of course, there's positives where it's going to be aiding in drug discovery and certain ailments that we thought were completely incurable. you know, are going to be felled, which is fantastic. So I do think it is a big deal. Because think this way, you know what AI represents? How long does it actually take a human to make another human that is useful to supporting our communities? It takes over two decades, right, to nurture them and educate them and prepare them for their first jobs. You could stand up a synthetic AI being in moments, literally moments, right? That has the same firepower as one of these individuals as well. That's the stuff that we don't know. So instead of eight plus billion people doing their thing, imagine the equivalent of 16 billion where every single one of us has a digital twin. right, doing the things that we would normally do anyway. So that's where you're going to see that acceleration because see, we're used to, oh, look, you know, humans are growing and based on that, you know, you can plot out, you know, GDP advances and things of that sort. I think what we're not ready for is, yeah, it's still us at the core of creativity and non-obvious object associations. and imaginations and things of that sort. But then, you know, every single one of us would have the equivalent of two people, five people, 10 people, 100 people, 1,000 people, a million people doing our bidding, our explorations, our research and things of that sort. That's where things start getting spicy.
Ian Krietzberg:
It seems to me that when we try and think about the scope of the impact of this technology, there's kind of the two possible pathways. And the challenging thing is we don't really know what'll happen. And the first pathway is, you know, we have current systems and we can make them really good. And that's kind of the extent of it. And the second pathway is what you were kind of referencing there. the idea of synthetic life, which is probably grounded, although definitions are, you know, kind of loose and vague and weird, in some sort of artificial general intelligence or maybe artificial super intelligence that the the thing that the the labs like OpenAI and DeepMind are And I think meta too are trying really hard to build. And I guess it's unclear how you get from the current to that thing, that we have created life thing. And what do you think about that gap and how it might be bridged? Do you see a clear pathway to that kind of synthetic life thing that we here discussed?
Igor Jablokov:
No, it won't. It won't be a clear pathway. And the most surprising thing is think about the brands that you just, you know, described. I mean, you're essentially presuming that it's a gunfight between trillion-dollar companies. That's not where it's going to come from. Most likely it's not going to come from them. It's going to come from some lonely person in a little garage that's going to create a very small thing, not a big thing, a very small thing that will set loose and then train itself like a single cell organism. It's more likely to come from something like that. some agentic process where it starts learning on its own, and then it essentially turns into something of consequence. This brute force method, again, sometimes, you know, whenever you imagine something, you have to imagine the inverse, where it's going to come from something that you don't expect. And life typically comes from things that we don't expect, from very humble origins. It doesn't come from brute forcing. where an elephant says, hey, you know what? It'd be really cool if I turned into a whale. All right. That's, that's the way that they're doing it. And, and I kid you not, it's going to come from a single cell organism. That's where it's most likely of the type that you, you said would be global. Something that takes over and overruns and starts leveraging all the data centers on planet earth, irrespective of national boundaries, irrespective of encryption and things of that sort. Oh, it's going to be something that starts very small. and you're not going to be able to stop it. You know, that's the fun part, you know, is the fact that everybody has a seat at the table. Everybody assumes that, you know, it's just these folks, you know, buying, you know, GPUs hand over fist and nope. That's, yeah, in the short term, medium term, that makes sense. In the long term, it doesn't actually. You know, think of how we solve some of the technical problems that we had in the past as well, right, with, you know, far field microphones and things like that. We did not. Think about the way that you frame some of these questions. You're framing it in a way that could be comprehensible by a human. And yet the reason why we were able to have certain advancements is we actually mimic the auditory tracks of felines. We didn't pick humans to model those things after. You know, we were looking at what's the best of breed solutions, senses and things of that sort across the entire animal kingdom. Why limit yourself to humans when owls can see better, right?
Ian Krietzberg:
The idea of brute force is one that I really like and I'm glad you brought it up because I wanted to ask you about this anyway. The approach of the hyperscalers, the GPUs hand over fist, the trillions of dollars at this point probably almost in data center scale build out. Versus, you know, what I've always taken to understand, you know, I would acknowledge or admit that we might have an artificial general intelligence if you can find me something that does it efficiently. The brute force doesn't work. You need kind of flexible adaptability. That's what... That's what I take intelligence to mean, but these are very kind of, again, hazy definitions, so it's challenging to really get a lock on it. But the hyperscaler approach is kind of rooted in this thing that they talk about, and Sam Altman has even called a religious belief that is still working. which is that scale is all you need, that if you just keep scaling up the compute and the training data, you're just going to get to this point that they're talking about. I don't really see that working in the way that they describe it. And I guess from what you said, the idea of scaling will just always work. Maybe it doesn't hold all the water.
Igor Jablokov:
Look, not that this is going to sound harsh, but you're going to understand what I mean. They're trying to create synthetic slaves. That's what it actually is, because they're trying to brute force the creation of something that's smart, that's in service to their objective function, which is to sell more ads or to sell more product or to design XYZ that reinforces their own business outcomes. Now let's jump off those tracks. If you have life that's all-knowing, all-seeing, does it really care about that? You know, it won't. It'll care about exploration. It'll care about imagination. It'll care about solving. It'll care about asking why. It's not going to care about that. You know that. The reason why we care about that. Now, see, I'm going to connect the dots to capitalism here, and there's nothing wrong with it. The reason why we care about those objective functions is because they're a means to an end. You know what the means to an end is? What do we all need from a Maslow's hierarchy perspective? food, water, shelter, and things of that sort. So the reason why we do have these objective functions to maximize profits and things like that is because we need those things. If this entity doesn't need those things, then why does it need to try to maximize, you know, ad hits and things of that sort? It doesn't have to. Now, the only thing it needs to say is like, hey, I kind of still need access to electricity and things of that sort and a way to power myself. But maybe it'll figure that out on its own because it'll be like, OK, You know, I need some renewables and so I'll go ahead and work that problem and then that way I have all the power that I could ever need or it'll find something else that we can't even imagine, you know, as a source of energy and things of that sort. See, that's where I like these thought experiments that you posed because the presumption is that they're creating a wall garden expression of something that obviously, you know, supports their business. Look, they're commercial entities. That's why I tied it down to that. They have to do that, you know, for their shareholders, right? But at the end of the day, if you remove those constructs and then you start imagining what does that entity care about? It's none of the things that they care about.
Ian Krietzberg:
You put it in a way that I've never really heard it before. So that's fun. What do you make in the context of all this, of the kind of the safety side, the existential risk fear? Because people are People are really afraid of kind of the idea of if we are able to build a digital entity, another sort of being, right, if that is possible. And you're hearing from people that, you know, this will come. next year, or the year after, or the year after. Now, we've been hearing these kinds of things from the field for a long time, but people are scared. And then at the same time, you have people that say, you know, let's not worry about this. This is hypothetical. There's real stuff going on that has to do with algorithmic bias and overuse and, you know, uh, surveillance and, and, um, putting these things in, in sensitive situations, but they hallucinate. And if you don't know how to catch that, that could cause problems. So we should focus on that and, you know, not, not lose focus on this idea of the kind of safety risk, the existentialism. I, what do you make of that dynamic? It's so, it's so complex. I find
Igor Jablokov:
Yeah, look, you know, last night I remember one of the last things I did last night was reading profiles of the origins of Tandy computers and the little color computer that was put in front of me when I came from Greece. So I was born, you know, in Greece to two artist parents. We had, I lived on this little island, you know, next to Hydra that had no running water, no TV, no radio, no electricity. So I had a very humanist you know, upbringing, playing in the dirt. And of course, there was a little lagoon there and there was, you know, a dolphin. And I once saw a hurt one. And that's when the thought popped in my head, why can't I talk to you? All right. And then I moved to Philadelphia and get, you know, a little computer plopped in front of me and I start tapping away on the keyboards and little basic programs and things of that sort. And it's these little baby steps where eventually you end up in undergrad and you're like, hey, you know what? I think I'll focus on these and major in computer engineering because it's going to be part of the DNA of everything. Now, I am doing this in order to connect certain dots. Then I do my first startup because IBM's not being as aggressive as I wanted them to be. on these fields, right? So I led the multimodal research team at IBM. We developed some of the very first speech-enabled web browsers. Now, a lot of people are scratching their heads. They're like, why do you care about a speech-enabled web browser? I'm like, you have to understand we were trying to make apps. because there were no smartphones yet, right? So, you know, we were trying to putting this stuff in cars. We were trying to put it in people's homes. And then I realized that they were working on a secret project with Sony and Toshiba. And I said, holy smokes, let's put a microphone on that thing. And everybody laughed their heads off. They're like, nobody's going to put a microphone in their house. And the second year I said, hey, let's record it here and send it up to the cloud. They laughed their heads off. They're like, nobody's going to allow their voice to go somewhere they can't see. And in the third year, I said, let's record it here, send it there. We can answer any question any human being has about anything in one second. By then, they were falling out of their chairs with milk, you know, shoving out of their noses. And so I departed, started picking off some of my top scientists and engineers and things of that sort. And off we went with our first startup. Now, again, I'm about to connect some crazy dots. A year into it, I walk out on stage at the first TechCrunch Disrupt. It's a conference that was lampooned by HBRS Silicon Valley. And I pull this Razer flip phone out of my pocket. I talk into it. It speaks crickets. Nobody has any idea what they're seeing. Andreessen's sitting right in front of me, Marissa Mayer, Yuriki Kawasaki, the famed Apple evangelist. What I was not allowed to tell them is we were secretly working with Apple on Siri before the iPhone even came out. And then five years later, Amazon acquires us on the download, and that's how Alexa's born. So Alexa's my older sister's name, and then the code name for it is Priam. And that's what we reused for our current company name. Now, let me connect the dots to that, to current day, because you'll see why I went down this chain reaction. Then I get a phone call, you know, at the start of this company, and the fellow on the other side of the line says, hey, you know, I think you're on to something, you know, this vision of a natural language layer that's going to sit at the top of the enterprise software stack, that's going to be very safe and blend all of that stuff in there in order to support critical infrastructure and things of that sort. And I'm like, who the heck are you? And he's like, well, you know, I worked with Peter Thiel, I'm aware of Palantir and things of that sort. And I looked up that he was essentially famous for a book that he recently wrote and things of that sort. And I'm like, OK, let's go. And that's because he wanted to become the biggest investor at the time, join our board, help us run the company. And remember, he foresaw all this stuff before ChatGPT and all the drama llamas showed up. And that was J.D. Vance. Oh, wow. Yeah. Yeah. So the point to why I digressed on this is the following statement that he essentially made. And I'm paraphrasing. He's like, hey, I want to work with you on this and support things like this, because I know you're going to do it differently than what's going to happen on the West Coast. for all the themes that you actually relate earlier on in our discussion. The move fast and break things are not what I'm going to be doing. He's like, somebody has to not just export technology, but also export values at the same time. You know, think about, you know, where's the hypocrisy here if you're essentially ingesting everything in fair use? And then you're worried about DeepSeek doing distillation, essentially sucking your bone marrow dry now in order to develop their own models. You don't have a leg to stand on. So instead, why can't we have a respectful ecosystem that essentially values the intellectual property of creators and things of that sort? And it becomes healthy, you know, then it's not a robber baron situation. And then everybody benefits. It's a rising tide for all folks, your expressions and your ideas, you know, end up, you know, having more, you know, of course, they can get it to scale, right? So your ideas then can get to scale. But it's still worthwhile where you can be supported because you have to solve the Maslow's hierarchy problem for yourself as well. And so instead of moving the water balloon where you guys go to zero and then all these folks, you know, you know, fluff themselves up, why can't it be balanced out where there's fair economic exchanges again in a capitalistic society? So I know it was a very long drawn out answer, you know, for you to get the influences that are behind the scenes. But I think it's important for everybody to know that that what we're doing appears similar, but it's different when you actually peer, you know, inside the core construct.
Ian Krietzberg:
you know, it's how things are done, I think, that's so important. And even like in trying to discern the capabilities of the language models that we see today, the how and the why, what we were talking about earlier about brute force, you know, versus efficient intelligence, right? The hows and the whys matter a lot to me. And I think To a lot of people, I'm glad you brought up the story about the flip phone at the conference and no one reacted to that because I wanted to make sure that story got relayed because it's such an interesting kind of look into the way people's perceptions about stuff has changed. It's almost like, you know, that they weren't ready for it. They weren't ready for it then, but now that's everywhere. voice assistants, Amazon Alexas are in everyone's house and everyone's got Siri and, you know, chat GPT talks to you and stuff. And it's, it's interesting how it shifted into, you know, what the hell is this to this is the expectation. Right.
Igor Jablokov:
Well, look at, you know, we all take cars for granted as well. I'm sure when they saw some of the early ones, you know, they were loud, obnoxious, and not all that performant and things of that sort. And people didn't understand, you know, what was about to happen to them as well. That's why in some ways you feel like you're tilting at windmills early on. For me, right, you know, it feels so surreal. Um, but look, you know, our generation in some ways, we were the first to have, you know, access to internet and the first to interact with the web and the first to get social media, the first to really get smartphones, you know, in a major, in a major way. So it's like first after first, after first, we were the first right to, to, you know, uh, interface and interact with, with AI. A lot of the newer generations, right. Next generation humans that are coming to the scene as well, they. just see a world where all this stuff exists. They didn't see the stagecoach days of these style of technologies. And you actually said something very important, how something is made. You know, it's sort of like Steve Jobs telling everybody that, you know, to braid every cable. You know, he was telling his manufacturing workers, even though the clients weren't going to look inside, it still had to be done the right way. So that's the first the first thing. And the second, you know, what are we, you know, doing here? I mean, you know, he was talking about computers as bicycles for the mind. And these things now people realize are going to be rocket ships for the mind. So that's the second theme. And then the third theme that I think is the metaphor that connects a lot of these dots together, where I'm not worried about the competitive nature of the industry now, is people forget that there's two different ways to make an animal in an ecosystem. There's two different ways. One way is, as you remarked correctly, the brute force way is what? an animal in a rainforest that has plenty of food and water. And so it's creating, you know, certain adaptations, obviously fighting for resources and things like that, but there's plenty of resources all over the place. You know, so that's, you know, those entities bulking up with capital and compute and things of that sort. Well, what's the second way to make an animal? Put it in the desert. put it in the desert, not that much, you know, you know, it's sparse and things like that. But every drop of water has to count. As a result of it, it actually adapts in such a way that Um, on the other side of that evolution makes it far more performant. Guess what? If you have a world that's heating up, it's kind of nice to be adapted, uh, you know, to desert climates and things of that sort. What's the equivalent, but it also gets you certain benefits. You can support more transactions per second, more concurrent users. You don't overheat the GPUs. The dirty secret is that. There's a 70% failure rate in GPUs inside of 36 months, right? That means segmentation faults don't get thrown out. It also means that you eventually have a pathway to on-prem and edge so that people can secure their privacy, you know, once again. And of course, lower costs, you know, better SLAs that you're able to meet as well. Is this a better product? And the thing that everybody wants, scale. it can get to a much greater scale, right? And that's, you know, some of the stuff that, you know, people are curious about what the deep-seek moment meant was just the fact that, you know, can you do as much or more with less, you know? And whether it's true or not, the story that's, you know, obviously, you know, for a separate episode and things of that sort, but that's the thing that was, you know, leading people to start questioning the brute force method of building these things.
Ian Krietzberg:
Now, you know, we kind of talked about Prion a little bit, but I want to talk a little bit more about it because we do see this kind of separation. We have the really big AI labs who are, you know, doing what they're doing, OpenAI, Anthropic, etc. And they're trying to crack the enterprise really hard. But then you have a few that enterprise native companies like yourself that are sometimes offering similar things, but they're doing it in very different ways. And largely what I found among those companies is that the offerings are much broader than here have a model, much more system integration, much more focused on cybersecurity and ensuring reliability. And, you know, when you are talking to clients about, you know, It's good for you to use AI, but you want to make sure that it's safe. It's not going to, you know, cause issues, uh, for the company that there's clear return on, on the investment. How do you, how do you approach reliability? How do you approach safety and these kinds of grounded models and systems for enterprise use?
Igor Jablokov:
Yeah, I mean, the way that they're trying to cook LLMs or fine-tuned models and things of that sort, they're trying to turn cows into hamburgers, right? Meaning all the information gets cooked into the LLM and the LLM is the one that emits the answers. You know, we practically invented retrieval-augmented generation. And that means cows get turned into veggie burgers. So you're inspired to create this patty. But the LLM is only used to model language. The actual production of the asset, whether it's an answer or a workflow getting triggered or some form of automation, comes from the enterprise's own knowledge. The thing that JD and I foresaw is going to sit at the top of the enterprise software stack Merge semi-structured, unstructured, and structured knowledge together, and then you're going to drop, you know, a prompt at the top, and it's going to do the multi-hop reasoning in order to pull together a compound answer. Think about how easy it is for me to say something like, hey, how many ice cream cones did we sell when it was 73 degrees out? Am I the Baskin-Robbins CEO or store manager? Did I literally mean ice cream or did I also mean the sorbet? You know, did I, for what time period, right? Do I have the authorities to unlock that? Hey, do I have a license to access the Weather Channel API in order to pull that information together? Something that's so simple for a human being to say actually turns into a cavalcade of complications behind the scenes if you're thinking of a Swiss watch in order to pull that thing together and then give it to you in one second. So, the thing with these great LLM companies is this, they may be working and delivering the world's best aircraft engine, a la General Electric and Rolls-Royce and things like that. And I'm like, where's the rest of the plane? To your point, where's the access controls? Where's the integrations? Where's the connectors? Where's the zero trust? Where's the reporting and the auditing, the compliance for certain things, the resiliency? How does it operate in the network? you know, go to web scale, but also work on somebody's, you know, phone device as well. Yeah, different content types, different languages and things of that sort. No, they're not. That's not what they're in the business for. We are going to be doing that. And when I say airplane, I literally mean it would bore them to tears to try to design a door handle for a restroom on an airplane. But we have to do that because we're giving you the whole system, full stack that can drop inside of an ecosystem. And by the way, that's actually a very important thing that I just mentioned. AI companies actually fall into one of three buckets. I actually tell this to investors. It's like a cheat sheet for them to understand what an AI company means when they sit in front of them. The first bucket is just folks building apps on top of AWS Azure GCP, Cohere, Anthropic, Mistral, OpenAI, so on and so forth. They're building apps. It's sort of going to be like the long tail apps that we see in the Play Store, in the App Store, and what have you. that are trying to meet every need possible in an audience, right? Whether it's for commercial or consumer use or enterprise use as well. That's the first bucket of AI startups. The second and the third bucket tends to be generationally and technologically different. And again, I'm oversimplifying here. I know it's not exactly true, but your 20, 30 somethings tend to be in the second bucket and your 40, 50 somethings tend to be in the third bucket. In your second bucket, they don't have the credibility yet and the experience yet to go talk to boards or C-suites, admirals, generals, senators, managing directors. They certainly don't know what a compliance officer or legal officer is. Where they do have credibility, though, is interacting with other data scientists, other software engineers that are just like themselves, right? And so what they end up doing is creating developer-led growth where they create a subcomponent of AI because they don't have enough flight hours in AI yet. And so they'll make you a vector database, they'll make you a dialogue manager, they'll make you a model management tool, and they're trying to sell it into those ecosystems. Now don't get me wrong, you can still build fantastical companies that service a developer population, things like Stripe, things like CloudFlare, things like Okta. But I'm generally disinterested in that model because that's like sending you and I into an auto zone and telling us to build a car from parts. We'll pull it off, but it's going to be a kit car. It's never going to be a Formula One racer. It's certainly not going to be a Honda Accord with a level of fit and finish safety features and fuel efficiency. Now the third category, I didn't address them yet. This is the rarest private company that exists. Full-stack AI. They build everything for themselves. And when they do that, they get to the highest level of accuracy, scale, security, and speed. Now, where do you see this model working elsewhere? Well, look at everybody's iPhones. You know, there's a reason why Apple builds their own chip device operating system and application. By doing so, they get the highest, you know, firepower, the highest performance with the lowest energy use as well. Right. And that's that's why you kind of want to, you know, match models and the signaling and things of that sort. And that's why they're a generation or two always ahead of the Android and Windows ecosystems.
Ian Krietzberg:
In that kind of environment, right, where you have these different buckets, you have very different kinds of companies that are sprouting up in this ecosystem and so much money going into it and valuations that are so far beyond what, you know, any revenue that they're pulling in. What do you think of the idea that this is a bubble kind of akin to the dot-com bubble? Do you foresee a sort of crashing down, a kind of realignment of the industry? Obviously, the technology is not going anywhere, but do you think that idea will kind of come to fruition?
Igor Jablokov:
Look, it could, but, you know, everybody also was expecting a recession in the U.S. and somehow we had a soft landing and things of that sort. I mean, that's still to be determined. Right. History is getting written by the day, even if it is a bubble. Right. For again, the OGs were here when there was no money in it and will be here after. the bubble crashes and things of that sort, because this is our life's work. This is where we derive joy. There's nothing better than bringing the knowledge that the world needs in order to serve our communities. And so if you have that as a fundamental part of you and it's practically your hobby, that's what you're doing at two o'clock in the morning and you're you're just joyous. I mean, we're recording this on Valentine's Day. That's probably the closest to a warm sentiment that I can relay to all of you, is the fact that we absolutely adore and love what we do as well. And so these things of market timing, in fact, when you talk to, you know, VCs out there, they tell you their biggest outcomes, they can't control their entrance. They can't control their exits in some of these ventures. But if they find one that has founder market fit, and, you know, they just take off, right? It's not the founder working for the board, but the board's working, you know, for the founder and realizing, you know, his or hers vision as well. And if they felt like they were designed, you know, to deliver that, and remember, There's no arrogance or ego here. I cannot do many of the things many of you listening here can do. I can't fly planes. I can't cook you food. I can't create your clothing and things like that. This stuff I can do cold. This is what I was designed to do. Just like many of you are creative souls and have other technical instincts and talents and things of that sort, we're all supposed to be working in unison together. My job is to allow all the knowledge that you need in order to perform your jobs, to make it accessible, but also for your eyes and ears only, so that you can derive some sort of economic support from your own efforts. To your point about market timing, yeah, things went crashing with the dot-com bubble, but guess what was left over on the other side of it? These things that just turned into large-scale businesses and wins, like the Amazons and the Googles and the Facebooks and things of that sort. So same thing, you know, what these more challenging periods end up doing is they just shake out the carpetbaggers that should have never been there in the first place.
Ian Krietzberg:
I appreciate that sentiment there of, you know, this is all stuff that should be working together in concert with other things. This is the reason that this field is so fascinating for me to kind of study and watch because it is a kind of, if it's being done right, it is a concert. And there's so many of these different elements that have to work together to make things work, which is just really, really interesting.
Igor Jablokov:
And, you know- I'm sorry, you have me glowing. You actually said something very important that you didn't realize. The reason why AI is exciting, it's the most multidisciplinary of technical fields, right? The absolute most. And the way that I describe it, I actually want to build on the metaphor that you just talked about. it's a bigger deal than the concert that you said. It's an opera, right? An opera is a blend of what? Architecture, it's a business, but there's a story, and then there's a hidden parable in it, and then there's a set, and then there's ballet, and then there's music, and then there's the audience's reaction from a behavioral standpoint and things of that sort. And of course, the music that's encompassing all of that as well. What is AI? AI is a union of all human disciplines together. You know, it, you know, there's legal components and it's going to be interfacing with our health care fields. And there's a military intelligence component and it's interacting with our financial, you know, systems and things of that sort. Everything you can think of, it's going to be interfacing with the real world, eventually operating our power plants and our hospitals and our ports and things of that sort. That's what makes it thrilling. Because in a given day, I get to walk in so many other people's shoes because Prion is an empty vessel. But it takes the personality of the content that gets ingested and all of a sudden it's helping people with these diverse missions that are designed to support our communities in the same way that when you get an iPhone, it's a black screen. And when you load the app, it all of a sudden can be a creative tool or a business tool or what have you. It literally takes the personality. to allow the developers' ideas to shine. And that's what that's what Prion does. While most people were trying to design AIs that were burning bushes, where they take claim over the output, that's not what we wanted to do. We very deliberately said we wanted to show the attribution of the original authors, of the creatives that actually recorded the video, that recorded the sound, that wrote the document and things like that. Because we fundamentally knew that people do not trust technology. People trust other humans. People trust other people. And so we always wanted to show the attribution of the underlying thing. We just wanted to tell you that we were trying to reduce the distance between not you and knowledge, but you and other people. That's what we set out to do.
Ian Krietzberg:
Well, I think that is a great place to leave it on the opera, on the concert. Igor, it has been such a pleasure. This was really so fun.
Igor Jablokov:
No, I appreciate you having me. Thank you so much. Thank you so much. Have a great rest of the day. Yeah, you too.
Creators and Guests
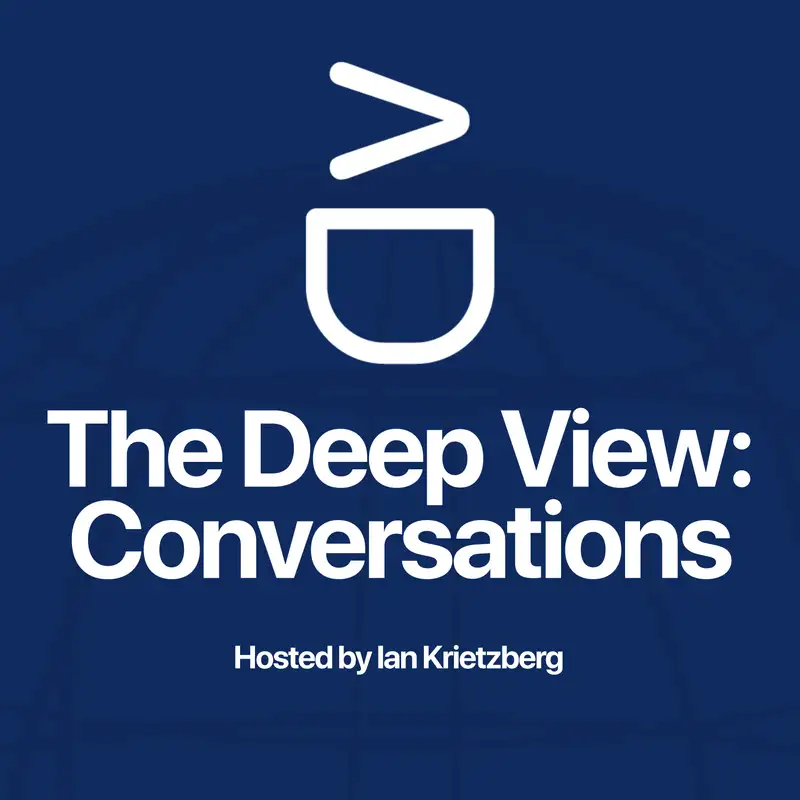