#13: The climate impact of AI - Shaolei Ren
Ian Krietzberg:
Hey, everybody. Welcome back to the Deep View Conversations. I am your host, Ian Kreitzberg. And today we are talking about the sustainability side of artificial intelligence. My guest is Xiaolei Ren, an associate professor of electrical and computer engineering at the University of California, Riverside. Now, Xiaolei has published a number of papers about these kind of unforeseen hidden impacts and hidden costs of the AI buildout that we're currently in the midst of. The first had to do with the water consumption associated with the data centers that power generative AI, and the second had to do with the public health costs associated with those same data centers. Now, running through all of this is the idea of the scale of the energy impact, the electrical intensity, and carbon emissions associated with powering the data centers lined with stacks of NVIDIA GPUs that are needed to not just train AI models, but to run AI models. It's this kind of interesting and confounding dynamic as the integration and adoption of generative AI is pushed harder and harder, the costs are stacking up. We take a look at all of them. Shaleh, thanks so much for joining me today.
Shaolei Ren:
Great to be here.
Ian Krietzberg:
So you've published a few research papers kind of examining the sustainable impact of the generative AI push, the impact of this race that we've been seeing. And I'm really excited to kind of dive into some of those papers. I've been, you know, as we just talked about, right, I've reported on both of these papers. I think they're very important. And I kind of want to go I guess chronological order, right? So the first one that you published, this was back in 2023 when the preprint came out. was about the water impact, the water usage of the kind of data centers associated with generative AI. And just to start with, what was the inception behind that? Because that was not something that a lot of people were talking about that wasn't super in the public sphere. Why did you think, you know, let's examine the impact, not just on sustainability at large, but on the water consumption associated with AI?
Shaolei Ren:
Sure. Actually, I started to work on this data center water footprint back in 2012 or 2013. So that was the time when I published my first paper on this topic. And at that time, there was like a very small amount of discussions around water. Most people were talking about carbon and how would we reduce the carbon emission for data centers. Maybe that was due to my childhood experience. I grew up in a small town in China, so where the water access was just very limited. We only had like half an hour of water supply each day. Of course, the situation has changed, but at that time when I was like five or six years old for a couple of years in that town. And then I moved to a bigger city. But yeah, so I think that somehow, whenever I saw this water issue, I was really passionate about this problem. And I tried to study what is the impact of the water. And the more I studied, the more I realized that water is really complicated. It's not like the carbon. For carbon, people typically look at the total amount of emission, but water, if you just look at the total number, that's not sufficient. We also need to look at the water source and regional water amount and also the peak water demand. So those, I mean, the more I study it, the more interested I am in this topic. Two years ago, there was a lot of research interest in the carbon emission for large language models. That's the motivation for me to resume my research on this topic. preprint at that time. Actually, when we published our preprint online, we didn't really think about any menus for submission. We didn't submit our paper until later that year, actually December 2023. And the paper now has been accepted and it will be officially published in Communication of the ACM. probably in just a few months. I'm really happy for this acceptance. I think it's a good recognition of this topic among the research community.
Ian Krietzberg:
Yeah. Why didn't you think initially about submitting it for publication?
Shaolei Ren:
Because we wrote a proposal to the NSF about reducing the water consumption for AI data centers, we thought, okay, some of the findings were really interesting, and maybe we just published as preprinted. We didn't have the pressure for publishing or perish. Yeah, just interesting. And because we already published some results early on about the general data center workloads, like the first paper in 2013, and then later we also have some papers around that topic. So for us, it's not a great deal of effort. We just need to collect those data and then use our methodology. Because we have those background knowledge. If you haven't ever worked on this topic, probably you know, the terminologies, because we're not environmentalist. We're not, we're electrical engineers and computer scientists. So we're more familiar with the computer system, scheduling, resource management, load balancing. But when it comes to like the cooling tower, this temperature approach, yeah, it's sort of a totally different world. We don't really speak the same language. I think that's the sort of the main barrier for many computer scientists or AI researchers. But since we already had that background, so for us, it wasn't really hard. Interesting.
Ian Krietzberg:
That's kind of cool to know. So I do want to kind of sink into some of your major findings. And from that report, I mean, I remember when this came out, and it was this kind of very significant number that, and I'm just quoting from my notes here, that training the GPT-3 language model in Microsoft's U.S. data centers can directly evaporate 700,000 liters of clean, fresh water. And that information obviously was kept under lock and key. No one really wanted that to get out until your paper came out. So I, you were just talking about how this kind of project is, is not a, was not a difficult thing for you to do, right? You were already kind of entrenched in, in that environment. Were you surprised when you found the data that pointed to that kind of scale? Cause that seems like an enormously significant scale. Did, did you sit back and think this is bigger than I thought?
Shaolei Ren:
I think for training part, it was sort of aligned with our expectation. But for the inference part, the most widely circulated number from our paper is probably 10 to 50 queries for 500 milliliters of water. That one was, we really didn't expect that. And also, but for the training party, we thought, I mean, 700 milliliter, 1,000 liters water, fresh water, whether that's considered as a large or small, it really depends on the context, right? So if you're talking about using that, evaporating that water in Arizona, it's probably not the, the perfect thing to do. But it depends on the context. But we've seen a lot of confusions among the general public about this number, because some people say, you know, I take a shower, I'm going to use this much water, or if I eat one pound of beef, then I'll be using, like, certain amount, a huge amount of water as well. But this is not really the right type of comparison that should be done. The reason is the water consumption is a technical term. It means the difference between water withdrawal minus the water discharge. So when you take a shower, you are withdrawing a lot of water, but you're not really consuming water by the definition because Most of the water you take for showering will be going into the sewage immediately and then that can be reused for processing and basically can be supplied to the residents, to the business in the short term. But the water evaporation for data center cooling, it just evaporated into the atmosphere And it could, of course, eventually it's going to be coming back. But when it comes back and where it comes back, that's really uncertain, especially in places like California. We just had a rain season, rain week, not season, a couple of weeks ago. Before that, we hadn't had any rain for several months, for almost a year. So this is the key difference between water consumption and water withdrawal. And by default, when you see those water numbers from those sustainability reports, they focus on the water consumption numbers. And also, this number is only for the operational stage. We really didn't consider the supply chain for making the AI chips. That's a huge part as well, although there's very limited information in the public domain. But when people talk about the water for their beef, it's actually roughly 95% of the water is for the supply chain to grow the grasses to feed the cattle. It's not the water drunk by the cattle. And that water for the supply chain of beef is really the green water. That's the water contained in the soil. It's not usable for humans. But the water that we're talking about is the blue water, taken from the rivers, lakes, and even drinking water sources. So it's just a different source of water, and it's just not the right way to do the comparison.
Ian Krietzberg:
I appreciate the way you laid that out, because I've encountered the same thing. It's not like the water is being disappeared, right? It comes back in some form, but it, it's all about the context. And I guess there's, there's an opportunity cost associated with withdrawals of water of that size that are going to this kind of targeted thing, especially, um, in areas that you're talking about out in the West and California. We, we know that, you know, the, the recent wildfires aside, drought is a perpetual problem of that region of the country. And I mean, the inference numbers are the bigger numbers, but it's the kind of wider ecosystem that you're pulling from and the impacts of that. I mean, you think about the impacts of drying up wells and the kind of aqueducts and groundwater and the longer term impacts of doing that in a drought, in a region that has drought. and then it's at the scale.
Shaolei Ren:
So if you look at some of the tech companies' reports, they could consume over 20 billion liters of water each year. And that amount of water is the same as the annual water consumption of a major beverage company, like PepsiCo. So in some sense, AI is turning those tech companies into a hidden beverage company, because they'll be ranked among the top.
Ian Krietzberg:
That is a wild way to put it. We've already talked about how this was not a difficult project for you to do, because this is kind of up your alley, right? To get the actual data, How difficult was that? Because as you mentioned in the report and as we've talked about, a lot of it is stuff that is not public information. It's stuff that the companies are very selective about how they talk about it. Did you run into that challenge?
Shaolei Ren:
Sort of, because for training part, that was slightly easier, because if you look at those model cars or some third-party study, they typically estimated the GPU energy consumption. That's not really precise, but it just gives you a reasonable estimate of the actual energy consumption, because they didn't really consider the server energy overhead. That could be substantial. But anyway, so we have some idea or some information in the public domain about the training energy consumption. For the inference, that's really the headache. Unfortunately or unfortunately, OpenAI, when they published their GPT-3 model along with the paper, talk about a very rough number. They say generating a certain amount of pages consumed, I think the word was like an order of something, some energy. How they measure the energy consumption in what environment? What is the quality of service level objective or latency constraint they had? We didn't know, but I just took that number. It seems to be really consistent with some of the general projection. Let's say we've seen people say a chat GPT query consume roughly 10 times energy as much as the Google search. I mean, the number that we use is similarly aligned with this general perception. So we use that number as a way to form the basis of our estimate for inference water. However, last summer there was a paper from UIUC in collaboration with Microsoft Research, and they measured the actual energy consumption in a production-grade system. and they consider real world service level objective, that's essentially the thing, means they need to give users the responses within a short amount of time, subject to a certain latency constraint, or not constraint, objective. So they try to meet that target for users to receive the responses. So if you look at that paper, the energy consumption for large-length models is much higher than initially reported by OpenAI. So in general, if you try to consider a model of the same size as GPT-3, I think the energy should be three to five times as much as the number that we use in our paper. So this also means if you are running a production grade system to serve the real world users, then probably the water consumption is like a three to five times as much as what we estimated in our paper. Actually, in our most recent update for the final camera ready version in communication of the ACM, we put a note on that part, on the uncertainty part, because we tend to stay on the conservative side, but the real actual number could be a few times higher than our estimate.
Ian Krietzberg:
That's actually crazy. And that's even, I wonder how you think about that uncertainty for some of the things that we've been seeing lately. You know, the pre-print was done before we heard anything about the O-series, the idea of reasoning models, chain of thought, inferencing. But what we're starting to understand is that even if these models are maybe more efficient to train, because it's doing more inferencing, the inferencing side is a lot more energy intensive. So on that level of uncertainty, when you see models that are now thinking for anywhere from 10 to a minute long to respond to something, I would imagine that should relate pretty easily. I mean, what do you think about how that relates to energy consumption, water usage associated with it?
Shaolei Ren:
Yes. In general, I would say having these more complex models, having this more complex reasoning process, which takes longer, takes more resources, and that will increase the number of calculations they perform. And also, of course, the energy consumption, the heat they generate, the more coding demand. So I would expect that everything else will be increased due to the more complex usage of the AI model for inference. And you're absolutely right. This inference stage, especially for those large popular generative models, the inference stage overall is the dominant resource-consuming part compared to the training stage. Because these large tech companies, when they train the model, their hope is that A lot of users will be using the model so that they can recover the cost.
Ian Krietzberg:
And that brings us to this problem that you have laid out. How do we go about or what's the solution to the water usage side? Is there a way feasibly to have your kind of inference and have your training and have your data centers and not also consume these massive amounts of water to do so?
Shaolei Ren:
I think water consumption is not necessarily a bad thing because there's usually a trade-off between water consumption and energy consumption. If you rely on water for cooling, especially this water evaporative cooling, then actually you can save your energy consumption a lot. You can reduce the carbon emission, you can reduce the health impact, air pollution. And so everything else will be reduced if you use water consumption. But in some regions like Arizona, then probably the trade-off should be more on the water side. If you use less water-intensive cooling technologies, you're using an increased amount of energy and carbon to trade off for the water saving. So usually there's a balance and we've seen some claims from this tech company that They will be using dry coolers that reduces the on-site cooling water to almost zero, which sounds like a great thing, but they also increase the energy consumption. And indirectly, they will be increasing the water consumption overall, because they're generating electricity that's also water-intensive. In many countries, even including the US, water consumption by the power generation sector, top one or top two sector among all the industries.
Ian Krietzberg:
The other paper that I want to talk to you about came out more recently than the water one, dealt with quantifying the public health cost of artificial intelligence. And this is another one, Xiaolei, that you're looking at things that the way you're quantifying things is in a way that I have not seen too many other people do. And I think it's helpful to understand the impacts of, of what we're dealing with. Um, and I guess, you know, the kind of same thing, what, why did you decide to look at it from that perspective? Cause we've seen a lot looking at emissions and the impact of that. There's been less looking at the public health cost of those emissions. Why do you think that's the angle?
Shaolei Ren:
I think when we talk about emission, I think by default, people link this emission to a carbon emission or greenhouse gases, which has this long-term impact on the climate change. But at the same time, there are also some regional impacts. that is more immediate, more tangible, and hurting people's health right now. And most people have a generally good idea about the long-term impact, climate change impact. they don't really know the impact that is happening now. So I think there's a knowledge gap. And there's a lot of misunderstandings. Even before the study, I had the thought that, oh, this regional impact is really local, like within a few miles of the emission source. But that's not really the case. So after our study, we realized that these air pollutants are traveling hundreds of miles. And there is almost 100 years of research in this air dispersion modeling. So we have a pretty good understanding of where the pollutant will be going and how they will be affecting people's health and how they are contributing to those adverse health outcomes among the general public. So we have a good understanding in this domain from the environmental research and public health research. typically they don't really look at the source, who is creating or contributing to these emissions. That's something is missing. So it turns out these AI data centers are just increasing. dramatically. And by 2028, that Lawrence Berkeley National Lab predicts that the US data center, mostly driven by AI, will be consuming roughly 12% of the national electricity consumption in the worst case.
Ian Krietzberg:
That paper's findings are pretty kind of stark to me, right? I'll list some of them. Basically that in 2030, U.S. data centers could contribute to approximately 1,300 premature deaths, 600,000 asthma symptoms cases, and a public health cost of more than $20 billion. Are these, you talked before about kind of conservative numbers, there's a sphere of uncertainty. Are these low balls? Do you think that or do they account for the kind of steady increase in new data centers? Or is this just based on what we have today?
Shaolei Ren:
So I will say it's lower estimated than the actual projection by some other study. For example, after our study, there was a report coming from Berkeley National, Lawrence Berkeley National Lab. They projected that the US data center energy demand in 2028 will be exceeding the number that we use to form our estimate. So essentially, in 2028, the public health burden will be reaching, could be reaching 20 billion US dollars, even two years earlier than our estimate of 2030. So in that sense, yes, I think we are really underestimating the actual potential impact. And also, I want to point out that when we say 1,300 deaths, that's not really like these AIs directly killing people. That's not the case. It's based on the terminology from the public health research. It's actually contributing to this death. For example, if you look at the WHO's report, each year 4.2 million people died of outdoor air pollution. This number is not counted manually. It's not like air pollution just directly killing people. That's not the case. They have a set of methodologies to quantify the contribution from the air pollution source. So we're sort of following the same terminology. So more than 1,000 premature deaths is contributed by AI. It's not like AI air pollution is directly killing people. There are some subtle differences between these two terminologies. But overall, I think our estimate is on the lower end, and it follows the state of the science from the public health research and also atmospheric research.
Ian Krietzberg:
I'm so curious. You're looking specifically at AI, but we know that even if generative AI did not exist, data centers do. The internet is run on data centers. The whole cloud is run on data centers. Do you know, have you quantified the difference in that public health cost between no AI in the data centers versus AI in the data centers?
Shaolei Ren:
That's a great question. So if you look at the current, let's say 2023 data, The AI computing, according to the report from Lawrence Berkeley National Lab, the AI computing is probably around 20 to 30% of the overall data center workloads in the U.S. But in 2038, the increasing part is almost entirely driven by AI. So if you look at the AI servers installed in US data centers in 2028, roughly, I think if I remember correctly, 70 to 80% of the energy consumption will be attributed to AI computing. So I think it's fair to say that this $20 billion, its vast majority is due to the AI servers. And that's why we need to focus on these sectors because it could potentially outweigh the health burden of on-road emissions in the future. the largest U.S. states, like California, with about 35 million vehicles. And traditionally, when we talk about air pollution, we typically think about these cars on the road. But due to the heavy regulation for the car industry, their contribution to the health burden is already decreasing steadily over the years. And I think it's a time to shift our attention a little bit to this emerging sector. And also, compared to the transportation sector, the data center industry has a lot of flexibility. You can build your data center in place A or in place B. There's probably not much difference from the technical perspective. But from the health perspective, there's a huge difference. And so that means we have more opportunities to address this issue than other sectors. So I think that's why we're sort of interested in looking at this sector and use our knowledge to make some changes.
Ian Krietzberg:
What are the best ways to address it? You think of a lot of different things, right? Like what you were talking about, almost the idea of sustainable data centers. Then there's energy efficiencies in the chips using maybe different ships for inference versus training because GPUs are really energy intensive and maybe there's a different option. Um, I, what are the kind of approaches? And then there's the idea of regulation. I mean, you were just talking about cars and their public health burden has been going down because we have policies about that. And we've had, um, in the past. policies that were pushing electric vehicles, right, and these other things. And even though there's a whole host of other, you know, problems associated with the supply chain of those, right, that there's been that kind of prong of push innovation on the efficiency side, and then also you're regulatorily incentivized to do that. What's the approach here and how feasible is it?
Shaolei Ren:
I think there exists a a wide spectrum of solutions, from the technical side to the policy side. For example, we can use, look at the DeepSeq. They are already improving the energy efficiency quite dramatically compared to some of their competitors. And we can reduce the model size. We don't have to always rely on those large, unnecessarily large generative models. So for some domain knowledge, small models will be performing equally well, maybe even better than those large general models. So I think this is, and also you have the scheduling, so you could put data center training or AI training in different places. And we use META 2023 as example according to their US data center locations. Suppose we can move certain workloads around due to the geographical differences across the US. If you train the AI computing in certain locations, you can reduce the health burden by almost a factor of 10. And the reason is different locations have different emission efficiency for this criteria air pollutants, like particulate matter 2.5. And also different places have different wind directions, wind speed. So with population density, So just to give you an extreme example, suppose that we're doing the training in Death Valley, the public health impact is probably very little. But if you do the training in Manhattan or LA downtown, then that's a whole totally different story. And so I think if you do this, and the AI training is flexible. It's not like once you build a factory, you have to do things over there. These tech companies have tens of data centers around the world and they can smartly choose the location at the right time and at the right location to minimize the impact without really affecting their business. So these are on the technical side. For the policy side, I would say it's hard to push for the regulations, but it might be easier to start with some incentives. We've seen some Senate bills and Assembly bills in California's legislative legislation this year. They're trying to, like, if you meet a certain efficiency standard, then you will be qualified for some tax incentive from the state. So that could be an easier way to start the conversation.
Ian Krietzberg:
Yeah, it's all about incentives. And the interesting thing is, to a large degree, The cost that we're talking about here, there's also a monetary cost to it. It would be as much as I'm focused on the sustainability side, easing the public burden side, in doing that, It would be cheaper to like if these data centers were located in smarter areas if these data centers were powered, you know, during the daylight with on site solar panels and if they were located in places using create clean energy grids and all these. other things and in places that the temperature is such that you don't necessarily need as much water to cool. It doesn't get as hot because it's cooler outside or whatever. There's a way to think about that. That would also make it cheaper for the companies. There's that incentive kind of baked in.
Shaolei Ren:
Absolutely. About 15 years ago, there was a huge amount of research trying to reduce the energy bills for these large tech companies. Also, over the last few years, we've seen research on carbon ware computing. And if you read Google's blog, they say that they're already doing this, trying to match the renewable energy to their data center workloads on hourly basis. And the other tech companies are doing something similar in this space as well. So I think the technology is already there. We just need to choose the right metric to drive the change, to improve the public health, reduce the water consumption, reduce the carbon emission, energy cost. And overall, I think it's beneficial not only for themselves, but also for the whole society. Because these bills are something that are paid directly, but this health burden, that's something, externality, it's a hidden externality of their operation. And they can definitely do a much better job than what they're doing now. And again, without really affecting their business, because I think the current mindset is still growing these AI capabilities. at whatever speed we can. So it's not really like a roadblock for their growth. It's just helping. I think it's going to be helping them grow in a more sustainable, more responsible way in the long term.
Ian Krietzberg:
And it is. Everything we've been talking about is such an important, I think, and interesting backdrop. to what is going on. And by that, I mean, there's so much discourse around AI, which is such a broad term, but generative AI. We hear from these same companies that are through AI, through their data centers, causing public health impacts, increasing unsustainability, increasing emissions, consuming water when we really need it. that AI will cure climate change, right? That's the kind of, that's not me, that's the verbiage. And, you know, other things as well, it'll cure cancer, it'll solve climate change, kind of whatever it is, this kind of magic solution. And so the public health costs or the costs that we're paying up front are worthwhile because we're going to have this thing. And I wonder what you make of that kind of dichotomy when you're trying to push for a world where this stuff is more sustainable and then we're hearing, you know, don't think about it too much. We're going to have a magic solution in some amount of time in the future.
Shaolei Ren:
Well, I think this type of cost, like health cost, energy cost, water consumption, those are on the cost side. But absolutely, there are potentials on the positive side as well. So like you mentioned, AI can help us. address the cancer and climate change and estimate, predict the air pollution pattern because otherwise you have to rely on those huge supercomputers that are really intensive or manually doing some manual work that's even more time-consuming. So my personal take is I think the overall benefits of AI is still enormous and it's outweighing the the negative side of, let's say, the energy cost and the water consumption and health burden. Because we need to quantify this cost, similarly as how we quantify the benefit. Let's say we are able to quantify the benefit in the dollar cost, and then we should be able to quantify the negative side in the dollar cost as well. The energy cost, that's easy. It's something that you pay directly on your bill. Health burden, that's also something which we can convert to a dollar cost in a fairly robust manner, because it doesn't really depend on who is in the office in the White House. This metric is fairly robust across different administrations over the last nearly 20 years. On the other hand, this water consumption, it's a little bit tricky to measure this water consumption in dollar cost because water, admittedly, is very cheap. Although it's priced at such a low level just to ensure universal affordability, that doesn't mean the value of water is low. It's actually very highly valuable goods. I think, yeah, water is tricky to price the water. Especially if you look at the overall water consumption, some of the companies are using like a water evaporation system. They don't use water all year round. They only use water during the peak hours. But that peak water usage is really placing a stress on the local water infrastructures. And that is something, I mean, if you just look at the overall total amount of water consumption, that's almost no cost, but the peak demand is placing like millions of dollars of cost. So I think we definitely need to have a better way to quantify the water consumption in terms of the dollar cost so that we can make a more informed decision. And similarly for carbon, there has been some progress in terms of pricing the carbon, but the way to quantify the carbon cost is really varying significantly. If you look at the It's compliance market versus the voluntary market for carbon pricing. The difference is more than 100 times. The voluntary market is less than $1 per town, but the compliance market is typically $30, $50, even $100 per town, depending on the region. And also there's a concept called social cost of carbon that's used by the US federal government. But this pricing, the value just changes dramatically depending on who is in office. And likely we're going to see a significant job in the price. So we need a better and more robust way to price these factors so that we can make a more informed decision. But overall, I think this, if you factor everything negative into the cost, and suppose we are able to measure them in terms of the dollar cost, I think for those high-stake applications like scientific discovery, medical research, the benefits of using AI still outweigh the negative side. That's my take. Because my view is that we can't just look at the negative side. Especially given that sometimes we are not able to quantify the cost. For example, what does the one kilogram of carbon emission mean? I know it's hard to put a dollar cost, but we need to eventually convert it into a metric that we can use to guide our decision. Because we can't just say we need to reduce every kilogram of carbon. That means we just need to stop everything. So we need to have a way to measure the impact so that we can make decisions.
Ian Krietzberg:
A quantifiable cost-benefit analysis.
Shaolei Ren:
Yes, this is very important. This metric will drive the innovation. So that's why I think, in some sense, a health burden is a robust metric, it's a quantifiable metric, and it can help you make a decision that benefits the long-term sustainability as well. in most cases.
Ian Krietzberg:
Yeah, I think of the metrics, the clearest defined one is that the public health cost. If we think about, you know, a $20 billion loss on the negative side in public health impact, It should be pretty easy to tell if these models are producing an equivalent higher or lower amount in health-related benefits by, I don't know, easing burdens on the medical system, applying more health care, which is better for the, that should be a trackable metric. Is that trackable yet, or are you seeing things yet on the benefits side that have begun already to materialize, or is it kind of a couple years out?
Shaolei Ren:
I think it really depends on who is using AI and for what purpose. My PhD advisor is working on AI for medicine. She's been using AI for a lot of good purposes. For example, using AI for cancer diagnosis, using cancer for public health resource allocation. So I think for those type of applications, I mean, I think if I were to assign a dollar value to those applications, I would definitely put a pretty high amount, because that justifies the energy cost and the health burden.
Ian Krietzberg:
How much do the definitions matter here? Because when we talk about the cost and benefit of AI on the whole. We were referring to so many different things. Earlier, you were talking about how a lot of these data centers are existing to support massive large language models for generative AI, which is not always the same AI that we're talking about when we're talking about climate modeling and analysis on carbon emissions or identification of early tumors in cancer. It's not always the kind of same systems. These same massive systems are not always the systems that are deriving the benefit. So if the cost is coming from LLMs and the benefits coming from like older symbolic AI, right? Is it the same thing? How important is it to start with a place of definitions and figure out the kind of quantifiable cost benefit from there?
Shaolei Ren:
I think it's very important because AI is really an umbrella term. It means small models, large models, and good usage models and bad usage models. And some people use AI to generate a fake content, to generate misinformation. And they are also AI models. And I would say the benefits for the whole society of using those models are definitely negative. And so I think we need to have a more detailed view for each type of AI models. And also, the overall, even though the overall benefits of AI is positive, we need to look at, that's from the society point of view. But if you look at the individual communities, that may not be the case. Because we are building data center in a place like mostly in, many of the new data centers are just built in rural areas that are resource constrained. And that might further decrease their water availability and deteriorate their air quality. And there was, I mean, I think the executive order signed by Biden before he left office, about advancing the U.S. AI data center infrastructures. So in that exact order, it mentions public health several times, but one of the paragraph that was really striking was that they want to prioritize data center location in a place where the air quality is good enough and also the cancer rates is not higher than the national average. I'm not sure why they single out cancer rates, but clearly I think I would view this as implicit acknowledgment of the connection between data center construction and the potential impact on the cancers. I think, yes, from the society point of view, I'm positive that for most of the AI models, their benefits will be higher than the cost. But if you look at how do we allocate the benefit, how do we allocate the cost, that's something which may not be so obvious.
Ian Krietzberg:
No, it's not obvious at all. It's such a complicated thing, and it varies so dramatically person to person. Obviously, companies are going to say the benefits outweigh the cost, period. They want people to adopt it so they can get a return on their multi hundred billion dollar investments. Whether or not that's true, right, that's their kind of you know, they have at least one reason to say that the benefits outweigh the costs. I think the idea of what the technology will do or is doing in science in the hands of researchers is definitely interesting. But also the interesting thing is, you know, kind of similar to what you were mentioning with with Biden's order, which is now rescinded, but that that specifically called out where to place data centers, right? There's a consciousness of thought there. A lot of the researchers who use AI tools or machine learning tools, they're using small tools that are trained on specific data sets. They're very targeted. They're doing a very specific thing. And so it's very clear that for them to, for instance, monitor coral reefs, the electrical cost of that model does not outweigh the benefit of them being able to monitor those coral reefs. And so there's a significant difference in approach, depending on what you were saying, who is using the model. and why they're using it.
Shaolei Ren:
We need to look at individual cases and individual communities and try to make sure that the communities that are suffering from the burdens will be receiving sufficient benefits as well. And usually they're not receiving as many benefits as elsewhere, because they're typically the places that are under-resourced and disadvantaged in some sense.
Ian Krietzberg:
I am personally very kind of skeptical about identifying very targeted benefits and making sure like I'm more on the hand of like, let's figure out what's going on before we build all these data centers that are polluting. You know, you look at what's going on in Memphis, Tennessee. That's where Elon Musk's XAI data center is located. And there's been a lot of reports from the Southern Environmental Law Center about in Memphis, which is an area that's already dealing with air pollution issues. Now, because of the gas turbines that are powering Elon's Gigafactory data center, it's even worse. There's particulate matter in the air. It's not a good situation. And we're just in this rush right now. But the idea of the kind of quantifiable benefits, like can we inject math and science into the approach of how should we handle this? Then we have to do something. I think that's the interesting thing about the idea of AI, what it can do for the climate, for scientific research, for these other things. That is not quite in line with this discourse, it'll just solve stuff. It's not automatic. It'll give you insights that we can then take action on. But we still have to have the will to kind of take action on those insights, right?
Shaolei Ren:
Yeah, we definitely need a way to measure the benefits and measure the cost. And this efficiency, this metric will drive innovation. And like many years ago, 20 years ago, people look at the PUE, which was a very simple and in some sense, it's not a perfect metric, but it's a reasonable metric to measure the data center energy efficiency. And people use that metric to like improve their cooling efficiency, improve their power distribution efficiency, and that helps a lot to reduce the overall environmental burden. So I think now we need a better metric to drive the dialogue. and the innovation.
Ian Krietzberg:
And then the will to do it. And that brings me to my last point, which is in the scheme of all of this that's going on, this kind of mix of promise and concern, what do you hope to see happen in this space in the near term to kind of make sure that we're optimized to actually seeing those benefits across the board rather than having those costs outweigh them?
Shaolei Ren:
I will say, just use one phrase in our paper, attention to all. Because if you look at the aggregate level, the total value, it's going to be missing a lot of details. And it might be hurting certain communities disproportionately, very disproportionately. I mean, like in our paper, some communities are suffering more than 200 times as much as the cost of the other. communities. So this type of disproportionality, it will be hidden, will be invisible if you don't really look into the details. I think we need to have the will, have the way to look at those individual communities and use maybe a bottom-up approach.
Ian Krietzberg:
Well, Xiaolai, thanks so much for your time and for kind of shedding a light on all the hidden costs associated with what's going on here. Thank you.
Creators and Guests
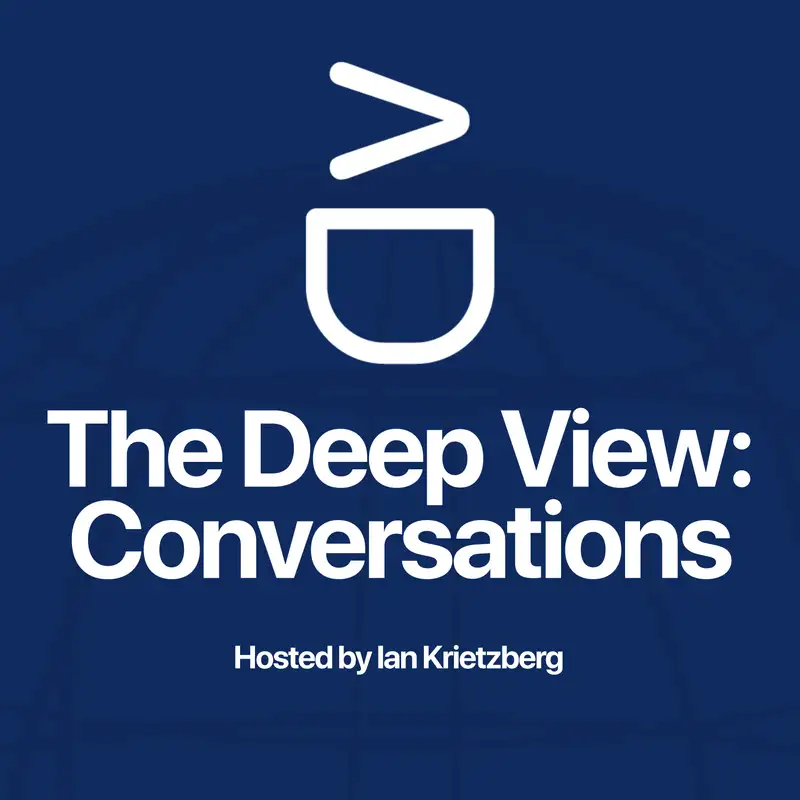