#10: The state of AI with IBM's Chief Scientist - Ruchir Puri
Ian Krietzberg:
Hey everybody, welcome back to the Deepview Conversations. We've got a really, really cool episode for you today. My guest is Dr. Ruchira Puri. He is the chief scientist of IBM Research, and we talk about everything from artificial general intelligence, the risks posed by more powerful AI, to the era of agentic AI that is perhaps on its way to current large language models, their promise and their limitations. It's really, I always think and find it so special and so interesting to get the perspectives of the people that are inside the industry, that have been working on building these systems, that have been dealing with and trying to improve what we perceive as artificial intelligence for so long. And I think you'll get a lot out of it. I certainly did. Ruchir, thanks so much for joining me today. Thank you, Ian, for having me. There's a lot, as you were just mentioning, there's a lot going on. There's a lot that I want to talk about. I do want to start with you, though, right? So you are the IBM's chief scientist of or the chief scientist of IBM Research. And I guess the first question I have, right? Why do you do what you do? Why did you decide that the discipline of artificial intelligence is the one that you wanted to pursue to this level?
Ruchir Puri:
I really think if you go back, actually, almost to the history of computing, sort of back in the early days, it's been the desire of, I would say, humankind to try to understand intelligence try to replicate it in all kinds of different ways. Go back to as early as mechanical calculators, people added numbers, try to discover machines which can help you emulate some of the function we do. And that is done in very different ways. Neuroscientists try to understand the brain, engineers try to replicate things and build things. And to me, I was, my passion has been around really automation. I love to build things, whether they are software programs or hardware, which can help us being more efficient in what we do and continue to push the boundaries of Hopefully, more and more time we can gather for us as a society together for our personal well-being, if I may say it that way. And that's what drives me, actually. And personally, that's what drives me. And from IBM research point of view, our goal since our very inception has been to really spearhead the future of computing. which is underpinned by really understanding of intelligence and emulation of it in many different ways, which is the foundation of computer science itself.
Ian Krietzberg:
Yeah. Yes, it is that that that's really interesting that there's a lot to get into. But I guess the first thing that I'll pull on, let's talk about automation. I think the way I appreciate the way you're describing that because a lot of terminology gets thrown around in kind of today's discourse when we think about current generative artificial intelligence and the kind of large language models behind them. But it is rooted in automation. And I guess when you think about the kind of field now of the different generative models that we have, the level that large language models have seemingly achieved, what do you think about where the technology is today? The large language model side of things, what we perceive as AI or generative AI, are you very impressed by it? Is it surprising after all your years in the field that we've made language models that can do what they do?
Ruchir Puri:
So I would say, have I been surprised? Did I predict how fast we'll come where we have come? No. I think we have really come very far. And I'll just point out a couple of fundamental things that have happened. Throughout the evolution of human history, if I may say, in every revolution, in recent revolutions, whether it was industrial revolution or information revolution, I would say reading, writing, abstractions were always priced. Like, you know, when the Industrial Revolution came in, it was about really people who could summarize things, capture information. Knowledge workers were prized more and more, always, actually. I would say this is the first time in this evolution that we have discovered that kind of information capture and its generation can be done relatively well by machines. I'm not at creativity part yet, actually. So I'm saying really capturing information, the information that humanity has created, understanding it, and summarizing it, and generating it, was thought to be very hard task and that has been addressed and that's a massive achievement. But I think where I still believe there is a significant gap remaining and that is a journey is this part of new knowledge creation actually. Which is again that's This is where I see the gaps exist. Could these models reason? Yes, they can reason on the existing knowledge. But to me, the progress in our journey as a society is made through creativity and creation of new knowledge. Now I'm going to step back and say, I think we started the discussion on automation. I usually try to sidestep the question on artificial general intelligence because to me, and I'll say one thing and then I think I'll really make the point I wanted to make. Whenever we say artificial general intelligence, there has to be something real, right? That's why we are saying artificial intelligence because there must be something real, that real is with us, each one of us. Interestingly, that real sits in a 1200 centimeter cube, consumes 20 watts and runs on sandwiches. Versus the single GPU, which as impressive as it may be, I'll call it braindead, it does matrix manipulations, consumes 1200 watts, one GPU. Doesn't walk, doesn't talk, doesn't have emotions. So I think that's why I tried to sidestep this question on artificial general intelligence, because general intelligence is very, very powerful. Energy efficiency is a fundamental part of computing. Don't ignore it. Everything is possible in this world. Everything is possible given infinite budget. You can just consume the energy of the universe and something is possible. So it's important for us to keep that in mind and that's why I say to me what is most exciting is as an engineer and a scientist, what could you do with this technology? And that takes me to automation. That you could do things that were very manual, very labor intensive and make it a lot more efficient and make it a much more enjoyable exercise. automate things so that you deliver a better service. automate things so that the processes are more efficient. When we show up at a department of motor vehicles or somewhere else, like our experience is a lot better, thereby we are better as a society and our journey as humanity has been always about automating, making things more productive, making things more enjoyable. And that's why I bring this down to kind of sidestep artificial general intelligence. I've coined this word called artificial useful intelligence. I'm more interested in useful than I'm interested in just general because general has a very broad notion in my mind which includes energy efficiency by the way. That's not independent of the general intelligence.
Ian Krietzberg:
This is really cool. We're just jumping right into the AGI. Cool. All right. I did have a lot of questions about that. I appreciate the sidestepping of it. I think AGI is a very dubious term. There's a lot of hype into it, and there's no universal definition of what an AGI actually is, which makes it really hard to understand what we're talking about. That kind of base idea, like, you know, if you're to use your phrase artificial useful intelligence, the idea of what some of these labs are pushing toward to have this kind of generally intelligent, whatever that means, open AI says it means they can use do economically viable work. Other people have different definitions. It's very messy. The idea of that, do you think we actually need something? Is there any usefulness attached to if this hypothetical AGI were to become real? Using systems that we have today, or slight advancements in efficiency of current systems, could this useful intelligence just be a much less dramatic, much more advanced tool, rather than the kind of creature that we hear about that people are you know, trying to create whatever that means.
Ruchir Puri:
Like, if I just go by OpenAI's definition, economic viable work, and you can attach dollar numbers to it, whatever you would like. I would argue we can do it today. It doesn't need to be, I don't need to wait for it for three years. I can do it today, actually. Economic viable work. We've been doing this, you know, at IBM, we focus on enterprises. and like there are lots of enterprises lots of capabilities we are working on with you know fortune 500 companies and beyond in doing economic viable useful work. There is no universally agreed definition of this general intelligence and to me I would like to push for a definition where We are not talking about nuclear power plants left and right. By that, I mean energy efficiency should be considered as part of this AGI definition. At what cost? At what cost are you achieving what you are achieving? And are there economically viable, more efficient ways of achieving the same thing? Again, I always focus on even it matters less to me on, there was a task given, potentially somebody could do it with rules based methods. There is nothing wrong with them by the way, really nothing wrong with rules. In some ways rules I would argue are the output of a intelligent model which is our brain. We absorb information, we sort of summarize it, analyze it and say by the way these are the rules of thumb. That is a output of a model which is a real intelligent model. So when we talk about this notion of AGI, to me, of course, it makes us, you know, the whole point of AGI discussion is, which I do agree with, is push the boundaries of our understanding of how do we capture the advanced capabilities of intelligence better. It's a very vague notion of how do we make progress. But have we arrived yet is a very interesting question. So I'll just take us back to our time when we were playing chess. Chess was thought to be the pinnacle of human intelligence, like the pinnacle of humans which can play chess and machines will never beat humans actually in chess because it was thought to be such a hard exercise. You figure out that machines as they become more and more powerful combined with very powerful algorithms can lead to machines that can defeat the world champion of potentially all times, actually Garry Kasparov in a live match. I said, well, that's not intelligence, actually. That's kind of brute force. Output is the same, by the way. And then you get to the point, well, you know, really intelligence is that game called Jeopardy. That is very hard, actually. That is intelligent. I said, OK, well, fast forward, I would say, 15 years later. You go on the live TV, and you have a game, and you know the outcome of that game, and say, Well, that's not exactly intelligence as well. And that event happened in 2012. So now we are talking 13 years back. Well, that's not exactly intelligence either, actually. And we push the ball forward, actually. And I think we will continue to. So that's a pattern. Anytime we arrive at it, we say, I don't think we are like that, actually. I don't think intelligence is that. It's something else. And to me, it is a guidepost for us to sort of move us in the right direction. I don't know if we'll ever know exactly we have arrived or not, actually. And that's why I like this notion of useful intelligence. Are we using it the right way? And that becomes, you know, this question of, of course, there's a question of responsibility and other things as part of it as well. But it's really about are we using it the right way versus have we arrived? Because every time we thought we arrived, we didn't arrive. Pre-2000 we didn't arrive, in 2012 we didn't arrive, I believe now also we will figure out, I don't think we arrived actually.
Ian Krietzberg:
I agree with you on that, I mean like the chess thing has shifted and now it's these reasoning benchmarks, but the benchmarks are hard to trust because we don't have transparency around the training data and there's no evidence that doing a benchmark equates to efficacy, but the idea of using it the right way is an interesting idea that I feel like there's more of a focus in the broader discourse right now around the capabilities, what you were just saying, have we arrived? Then are we using things the right way? It's almost a kind of capability overhang, right? Like, we have a pretty powerful advancement that is accessible. Like, for free, I can log on to Cloud and I have access to their latest model and in a lot of ways it's used for seemingly kind of simplistic automations like write this email for me or proofread this email, right? Is there kind of a series of use cases that you think people are not thinking about or should be exploring more that would really more tap into what this is enabling or could enable?
Ruchir Puri:
So I really believe we are at a inflection point in the way we've been thinking about AI and let me define that inflection point and that lead me to use cases actually. The inflection point is following. In the recent incarnation and the advancements in AI, it was all about training models, sometimes larger and larger models. And the way we sort of ran these models or inferred with these models was You deploy this model, you type in an input as you said, I can go to cloud, you type in an input, it gives you an output, you don't like the output, you as a human change the input. That's called prompt engineering, I believe that profession will go away. But I think now that those kind of systems in the world are called feed forward systems. You give an input, you get an output, you don't like the output, there's no feedback, there's no automated feedback actually. We are moving in this world of what I'll call sort of roughly now called agents actually. The underlying technology behind agents is following actually. you give a complex task to this system, it looks at it, it thinks, it breaks it down into set of steps and then it basically maps, calls tools in the world and I'll talk more about tools as well because that's very much related to usefulness. It calls tools, it looks at the output, combines them together, looks at the results, reflects on it and says I don't think this is agreeing with what question was given to me actually. Then it basically iterates on its plan, so it replans, it remaps to the tools, acts with the tools and then it again reflects and continues until it believes it has arrived at the right result. Those kinds of systems are called feedback systems. All good systems in the world are feedback systems. They're automated and they're feedback systems. Now I'm going to go to what is so exciting. Why is this an inflection point? This is an inflection point because first time in the recent incarnation of AI, we are moving from creating intelligence at training time only to creating intelligence at inference time. or when we are making decisions with these models, which is very different. In the previous era, at generative AI era, we were just using these models as, I'll give you input, give me output actually, and if I don't like it, I change it actually. In this new era of agentic ones, it is very sophisticated. That's much more applicable to real world because in real world, whether it is business processes or consumer processes, we use all kind of tools actually. Tools that enterprises have trusted for decades. Like, please don't try to recreate that. Simplest example I give is, if you give, now it is better, but if you go back a year and a half, you gave, no chat GPT, please add these two numbers and give some pretty random numbers. It's likely to get it wrong because it treats it as a token prediction problem. Adding two numbers is not a token prediction problem actually. Can please somebody tell it to use a tool called calculator That has been, you know, humans have used it for since, you know, really when stones were invented, actually, people were adding with that, actually, there is a tool, actually. So please use a calculator. Wonderful. And like that, there are many, many tools that we humans use on a daily basis. So this ability to be able to look at something, break it down, call the trusted tools, because I trust the output of those tools. I don't trust next token prediction on addition from chat GPT, even if it got it right. Because I don't know what will happen next time, actually. It may not get it right. So I'm not going to trust that thing. So to me, it is really about, as we continue to progress on this journey, we are moving into a very sort of powerful era, where we are moving from this generative AI era into a agentic AI era. And agents have been like the really hyped up words as well. So there's a lot of hype around them. Everybody knows. But there's something more foundational from a technology point of view under them. which makes them very very powerful and it will take a little while for it to settle down but this is where I believe lot of real world tasks will be automated. because real world tasks are never as simple as input output. They use a set of tools, they combine their outputs, they look at humans who are on those tasks, look at the outputs, reflect on them and until we get to that expansion of this intelligence, and move away from benchmarks only. Some of these benchmarks have interestingly only 100 design data points and everybody is reporting on them. Some of them are leaked as well by the way. So it's like I don't even know what is the purpose of beating your chest so much. It's a good guide post. But don't just get steered by I have achieved AGI because I have achieved to know that benchmark actually. I don't exactly know what that means. It's to me and that's why I like this notion of intelligence at inference time. Because you would know it actually if you don't like on a consistent basis, you're not getting the result. you would know it as well. And because the intelligence can be created and refined at the inference time, it makes it a lot more useful as well from a use case point of view.
Ian Krietzberg:
Definitely more useful, but also more risky, right? So you were talking about next token prediction and how you wouldn't trust that, but you would trust a calculator, you know, don't, don't give me the prediction of two plus two, use a calculator, tell me that. A kind of fundamental, seemingly, component of these systems is it's been called hallucination. Other people prefer the word confabulation. It really just has to do with the next token prediction, and it's not always accurate, and it's hard to, like, the model doesn't know if it got it right or not. So if we're not paying attention, maybe it gets an output wrong in a way that can slip through our notice, and the shift to agentic automation, taking that same underlying technology and having it do stuff. You know, I see Anthropics got computer use, okay, I just put out operator and, you know, the extrapolation of, you know, if you get a calculator wrong, okay, whatever. But if I'm asking the agent to buy airplane tickets or something, and there's still a hallucination going on there, I mean, how do you think about the reliability, the the hallucinatory aspect of these things as we move into what you're talking about, this more agentic era of automation.
Ruchir Puri:
Excellent point you're making, Ian. So assume for the time being, I'll take it from a more system point of view, and let's talk about hallucination as errors, if I may say. Like it's not right. There's something wrong with it. There's an error. And if the errors in a loop, they can amplify. like you know 0.94 raised to the power nth is pretty bad actually. So key is in the loop how do you detect the consistency, how do you verify am I heading in the right direction and I believe use cases that are much more amenable to this consistency checking and verification will be the first ones that will be successful with agentic technologies. And the rules can, the checking, the consistency checking or verification can be even rules as well, by the way. There are some rules we, humanity, have really captured in certain processes and say, well, if this, this, and this is not satisfied, this is wrong. There can be guardrails. around things. On things like software engineering, there are actually tools that are written to check your output and say, well, your syntax is wrong, this is wrong, that is wrong. Computer science has worked on it for like five decades and more. So there are many fields in which there are trusted consistency checkers which need to be deployed as part of that loop. That loop is not a quote unquote open loop. that loop has got gates in it and gates can be guardrails, gates can be, you know, compilers and interpreters in the case of software, gates can be rules in other processes and a variety of, you know, kind of diverse abstractions can be in those gates. But that is critical for the success of agentic technology because left unchecked exactly to the point you made, error is amplified in a loop and you know you are going to, it is almost like I am trying to go to the moon actually and if I am 0.00001% off, well from earth you are not going to land on the moon actually because you are going really long distance and every you know every mile you are veering off actually. So I think that is a reasonably good analogy of you are trying to go to the moon, you correct If you know you're going wrong, you correct actually, oh, I was going there, like I'm off a little bit, I need to correct. And you need to continuously correct that. And that is what we call consistency checkers and verification in the loop itself. And not every use case will be amenable to that. But the ones that will be successful, at least in the beginning, will be the ones that have those characteristics and properties.
Ian Krietzberg:
Yeah, I mean, the idea of gates, I like your moon analogy, you know, if you're consistently checking and if you can trust those checkers, right, if whatever your consistency checkers are, if adding the kind of deterministic more rules-based approach into a full system that, you know, we're moving away from models, we're moving towards systems, and that's where things will be impactful. And as that starts to become real, you mentioned a little bit ago the real world automation, where the generative AI era is not really going to fully automate away these tasks, because like you said, tasks are far more complex than input output. the push toward agentic might start doing that again within budgetary constraints. We don't know. So I mean, how much do you think about, you said at the top that you're your pursuit of intelligence in a non-human form, right? We could do more with our time away from the stuff through automation. But there's also risk factors in terms of job loss and economic disparity. Some impressions of that are very dramatic. We don't really know what's gonna happen. I think the consensus with everything in AI is no one really knows what's coming next. But on that side of things, on the impact of being able to reliably and successfully automate real world functions. How much do you think about what that might do and how much do you think that could have a negative labor economic market impact or might it go in the opposite direction?
Ruchir Puri:
So the way I look at it is it's a good idea to learn from history always. because it can be quite brutal if you ignore it. Every time we have thought that, and that was true in industrial revolution, transitions can be hard. Transitions can be hard for economies and we have to be careful about how we roll that out. In every revolution, when we have tried to automate and have been successful at automating the task, we have ended up creating more economic opportunities, broadly speaking. And that's how we have grown. Now this time around, it's more on the information side. Having said that, I'll give a very concrete example. Assume for the time being, and we are further away from it, that agentic technology is able to automate a decent amount of work. Let's say it that way. You're going to need people who are subject matter experts, by the way. Who's going to build these agents? Somebody should be building the agent. Agent doesn't create itself, actually. Somebody should be building that agent. And since work is everywhere, agents will be everywhere as well. And there will be people needed to train these agents, maintain these agents, operate these agents. And that becomes sort of a, I would say, intellectual exercise that your work will shift from just doing the task manually left and right to really maintaining, operating, and guiding these agents, actually, on a continuous basis. So your pursuit will shift from exactly doing that task to potentially doing a lot. Think of this as a powerful tool, actually. When hammer was invented, somebody was banging with a stone, actually. And hammer could bang a lot more nails, actually. Wonderful. Did we get rid of people who were really banging nails? Not exactly. We have a lot more of them. We want more use of nails, actually. So to me, it is really about, and I personally believe, I think history will again be a guiding factor on it, a lot more opportunity will be created overall. Also another interesting factor to watch is that I think this is the first time around that kind of the information worker if I may say sort of they are like oh like a software engineer actually as an example like post you see like what is the role of software engineers and so on and you know like nobody ever thought that will be ever possible actually is that like oh well I'm gonna send him my kid to computer science actually both my daughters are studying computer science and like okay The person who is in the job of a waiter who comes and serves you in a restaurant, his job is not going away, actually. You're going to need a vapor. If there's a leak in the house and you need a plumber, that leak needs to be fixed in your house. You can't have a LLM fix that leak, actually. It's not possible. So I think we are in a very interesting mix of how the work is getting automated. But from an information worker point of view, which is really the topic of this very hot debate right now, I believe you are going to have many, many jobs more actually. Because if assume agentic technology or its variants, nobody knows exactly how it will evolve, but all its variants are going to automate more and more work. Because work is everywhere, agents will be everywhere, you're going to need people to create these agents, maintain, operate, refine, feature function, that job is going to shift for an information worker. And it's going to multiply those jobs, actually, at least from what I can see, taking into account what we have seen for information workers before. That's my prediction, actually. If you ask me, my mental model's token prediction, that's my token prediction.
Ian Krietzberg:
Well, that's what I'm asking. So, you mentioned the transition phase, and if you look at history, transitions are rough. Do you think that, AGI aside, you know, like, legitimate, the reality of advancement, we are in the midst of a transition phase that is similar in scale to an industrial revolution or would you put this more of like the rise of the internet or the rise of social media? Is this a transition more along the path of where we were already going or is this a huge kind of focal point shift and are we ready for that?
Ruchir Puri:
I put it in the sort of the latter category. We are in a fundamental shift actually. The reason is that exactly the point we were discussing. If I may say the future of work could be redefined. The way work gets done could be literally rethought and redefined as well. Are we ready for that? That I would say is to a lot of extent is upon us. I do see a lot more debate, a lot more focus. You go to World Economic Forum. It's all about AI for several years now. This is not the only year, actually for several years now. And governments across the world, whether they are superpowers or otherwise, every government is focusing on this, trying to understand the technology, trying to understand the implications of technology, how to get access to it, how to democratize it, how to use it for the benefit of their citizens. This is different than sort of recent era of social media and internet and others. I mean, I equate it to sort of industrial revolution or the modern version of industrial revolution. where it literally changed the notion of work, what it meant to work from factories to information. And you're going to change it from really information to automation of that information, which is a fundamental shift actually in what has happened. Internet democratized the information. Information we never thought we could automate directly, that was always prized and I think we are figuring out many of these functions we can do a lot better and automate and our pursuit as humankind will be more on how to manage, operate, engineer, evolve these technologies or these, call them agents, but it's an overused and abused word actually, to what will happen to it. But I believe very strongly that we are at an inflection point equivalent of electricity, industrial revolution, and intelligence.
Ian Krietzberg:
Interesting. So it'll, you think, change work, but not erase work, just change how people kind of what they do. Not that they do.
Ruchir Puri:
No, I don't think it'll change what, like the task that we do. It'll change, certainly, how they do it. We didn't, like, let's go back to Industrial Revolution. There were cloth mills. People were building this by hand. We did not change wearing clothes. Like, we still, somebody manufactured. That was a fundamental shift, how things got done, actually, in a significantly more efficient ways, so that we can reproduce more of it at a cheaper cost, so that majority of the world can have it. You go to agriculture. automation brought in a lot more food could be created and distributed to more of the world as well. So I don't think we will change fundamentally the work we want. And also now I'll be a little bit philosophical as well. Work is fulfilling actually. like in your day you need work actually like this notion of I'm just going to sit on the beach and they're like okay you could do it for a while actually work is very fulfilling whatever that work might happen to be actually you know some I clean my house that's work actually I know somebody does gardening that's work but what I'm saying is that work is fulfilling and I don't think, and this is why this debate is so important that automating everything and removing humans out of this loop is like kind of very strange to me actually. Like okay, what are we going to do actually? Assuming somebody gave me a paycheck, just assume for the time being, this universal income, somebody gave me a paycheck, you are looking for still a very strange notion of this society actually, where like somebody just paycheck came and okay, that's interesting. I do not, this is only personal for me. I'm not speaking for directly IBM at this point. This is a very strange notion of society for me. I think work is very fulfilling for humans and we will change the notion of, certainly we will change the notion of work, but it is a good idea for us as humans to be involved in fulfilling work, whatever that might happen to be actually. and do it a lot more efficiently as well. Now, you may have self-driving cars, but that doesn't mean to say you will stop going from point A to point B, actually. No, until, of course, quantum transportation comes across. You don't need to go anywhere. We're quite far away from those points, actually.
Ian Krietzberg:
Yes, quite far. Quantum transportation would be interesting. It's a really interesting point of the right amount of automation. The whole universal basic income thing, I don't know. I don't think there's anything there. I think that there's so many existing ethical and to wax philosophical issues with what we have today and you think about how a universal basic income would work and just elements of control. It doesn't, it seems dystopian, not anything else. So it's all very interesting and it does, well, and here's an interesting point. I was reading an article the other day from the free press that my sister had sent to me where The writer does not come from the field, but basically his point was, and I'll link it down below, that he doesn't get AI. You see they're able to do certain things. We're on the verge of some types of automation. But if you look at other technological innovations throughout history, like plumbing, and flight, and air travel, accessible electricity and innovations in solar power and stuff. We see very targeted, applicable, life-changing things. Refrigeration, I think, was something he mentioned, that we have refrigerators and now people live longer because their food doesn't get diseased and sick. And considering the context in which AI lives, this evolution of internet and social media, the companies behind it, the scale of data, the cost of operation, the ethical issues around surveillance, potentially, and algorithmic bias and these other things. Is it of a positively transformative level of something like having a toilet inside? These basic things that did change our lives. And I wonder what you think about that. kind of perspective because I feel like a lot of people might be in that camp but you come from inside this field and so I'm sure you have an interesting eye on what that looks like.
Ruchir Puri:
So I would say I'm going to relate it to something that I think probably will be personal to a lot of people. Let's take healthcare. I think healthcare is probably one that will impact every human being at one point of their life or the other actually, given that our lives are finite. If somebody can tell me the diseases that have ailed us for very long actually, and we took the example of food and people will eat food or they will, you know, put massive amount of salt in it so that it doesn't go bad or the refrigeration was so fundamentally transformative. I think we as humans have an innate desire to live long fulfilling lives. And healthcare is one of the more fundamental pursuits of that. There are so many fundamental diseases to be cured if somebody like the whole art of medicine is statistical. Like who says you know 30 milligrams of this medicine is good for me and Ian both. like somebody just came up with a rule of thumb and just rolled it out actually. For all I know, you may need 45 and I may need 15 actually as an example, personalized medicine. That is really related to your data, your body, intelligence can be very useful actually. No doctor has time. They have no time. Your health care will go up to like a million dollars a year if we started rolling something like that with the help of a doctor, actually. Because you're going to need a dedicated doctor for you all the time. I believe technologies like AI can be transformative in those areas. Discovery of new materials, discovery of new pharmaceuticals. And I can go on, actually. Now, there might be other so near term use cases that one may argue, well, I'm not very sure. It's not as transformative as the as the technologies we have seen before. But on the other hand, there are fundamentally transformative technologies, which can literally make human lifespan longer. Those I would call are like fundamentally transformative, because I think this is encoded in our DNAs to prolong our life and pass our genes to the future generations. It's like beyond our control, actually. It's just encoded as what was given to us by the creators, actually, of that DNA. And to me, I think this technology definitely has the potential. to move the needle in the right direction, given this overarching desire of humanity to live longer, healthier, fulfilling lives, actually.
Ian Krietzberg:
And that brings me nicely to the last thing that I want to bring up. So what you were just talking about to me seems like it's the promise, it's the true promise of the technology. We get bogged down in terminology and tons of hype and stuff, but what you're talking about, the pursuit of that fundamental human push toward living longer and happier and healthier, right? That's the true promise of what we're dealing with in my mind. Now on the flip side, this is a technology that has been called by the people developing it a dual-use technology, a double-edged sword, highly risky. There's a whole faction within this field that's focused on and afraid of existential risk posed by the systems that they're developing. There are some people that are not directly developing those systems. It's a very kind of complex faction and how they function, but the idea of, you know, we are moving to general intelligence. If we're not there, things are just going to keep on scaling up because scaling laws is a law of nature in these minds. which is not at all clear, and we'll get an artificial super intelligence and then we won't be able to control it, existential risk. The kind of safety problem, the paperclip problem, it'll just, you know, if you ask something to produce paperclips and in achieving that goal, it kills everyone because people might make it stop, right? What do you think about those concerns about X-risk and the way you perceive the kind of double-edged sword of AI in pursuit of this kind of promise that you were talking about?
Ruchir Puri:
The way technologies can be safe is not by hiding them behind a wall, as some people will either like or say. Don't disclose it. I'm going to build these models and just trust me. Like I'm not going to tell you exactly what I'm doing. Neither I'm going to tell you where the data come from. I'm not going to tell you anything. Just look at the output and trust me. We, and this is a sort of our position from IBM perspective, we believe strongly in open technologies and open innovation and transparency and disclosure. Very strongly, actually. Of course, governments, really industry, academia, research community, all have a critical role to play in responsible production, rollout of that technology, but underpinned by this notion of open technologies. because the moment only a few players, whether they are state players or private players, start having control on that technology and we start moving others out of it, trust us. In the history also, we have seen this actually, how that goes overall. Again, I just go back to history. I'm a huge fan of sort of learning from the history and to me, the fundamental notion of AI itself and how fast it has progressed is actually is almost exemplar in how AI technology has been really underpinned by this open innovation as well. With the recent release of DeepSeek R1, which has been obviously in the news a whole lot and others. I believe in the end, the power of the people in this community will win. It will not be something closed behind a wall. Don't let other people happen. I've got a mansion, you know, I'm going to prohibit any new development actually. Like just keep the price high actually of this and that was exemplified by what happened this week. And in addition to that, open is necessary but not sufficient actually. In addition to that, we must have a joint partnerships across all major stakeholders from governments to industry to academia. for responsible tools and capabilities which are needed for really containing algorithmic bias, for having guardrails around it, for having, I would say, auditable functions. And that's why Open is so much better, actually. And that's why it is proliferating so much. And the innovation builds on top of each other so fast so that we can get to this, hopefully this Nirvana of pursuit of really longer, healthier lives with happiness faster than before actually. And to me and to IBM, this is really all about collaborative innovation in open community and really bringing that technology to the critical set of use cases where more productivity and efficient processes can be had.
Ian Krietzberg:
So to you, the safety cure is open science and the X risk aspect of it is not really a thing that you or IBM are really thinking about.
Ruchir Puri:
definitely we are not in the camp of existential risk. I'll just add one or two more sentences to it. Consciousness is a very not also well understood topic as well, actually for you, for something to be to be existential risk, there has to be consciousness. And as good as this technology may be, as good as we may get to the, I nailed the next token actually, it's still a next token and I do not believe that we are anywhere close to this point of existential risk broadly speaking but we are much closer to the point of making these technologies useful to a broad set of scenarios that matter to all of us, including things that are very close to us, from healthcare to pharmaceutical to real day-to-day world use cases that enterprises care about on a daily basis.
Ian Krietzberg:
Richir, I could talk to you all day, but we got to go. I really appreciate your time. Thank you so much for coming on.
Ruchir Puri:
Thank you, Ian. Really appreciate it. It was a fun discussion.
Creators and Guests
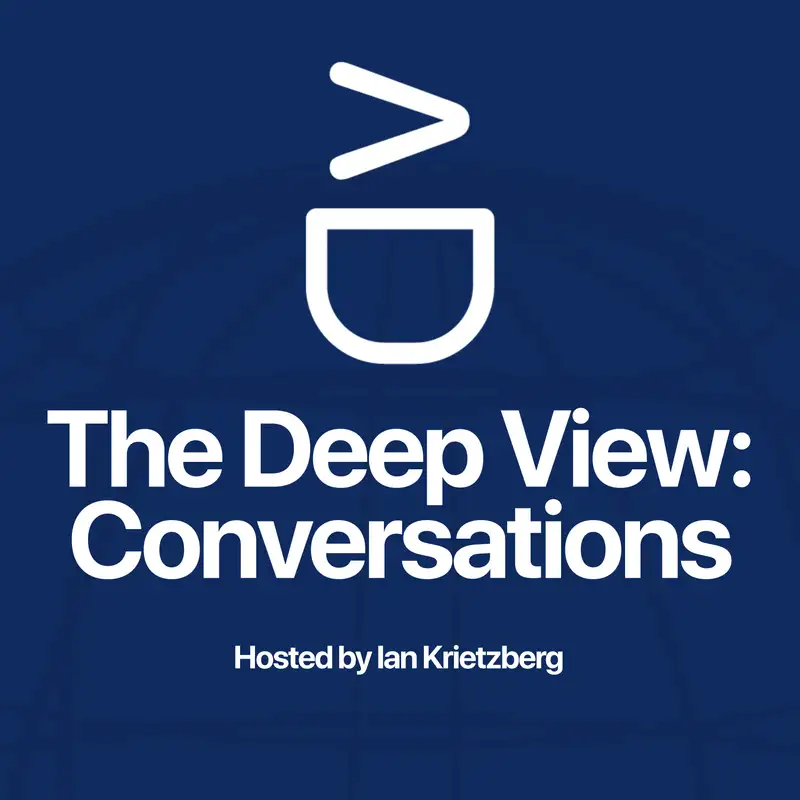