#1: Why We Need to Tame Silicon Valley - Gary Marcus
So there's a lot of ground to cover, right? AI is a really broad
topic. But I want to start with the book, Taming Silicon Valley. And
I specifically want to start with the very end of the book, where in
the acknowledgments, you write how you wrote
this book out of a sense of urgency and disillusionment at lightning speed.
And I'm wondering about, obviously, a lot of stuff has
been going on, but what kind of inspired that sense of urgency?
Well, I think you and I first spoke around the time that I
gave Senate testimony about a year ago. And I think even
then, everybody knew that having a good AI policy
was urgent for the nation. A lot of what we talked about that
day when I was at the Senate was the fact that with social media, the
Senate had not done well. It waited too long, made some bad choices,
and those bad choices became kind of enshrined permanently.
And we don't want to do that with AI. And everybody in the room seemed to understand that.
And yet, I watched the clock and the calendar tick away and
saw that nothing was really happening in the United States. The EU has the
AI Act there. And I think that's to the good. It may not be perfect, but
it's a great start. The state of California is actually trying to
do something now. The federal government, there
is the executive order, which is great, but it's not law. The
way our country works is the president can't make laws. are
actual laws here. Anything a president puts in executive order
can just vanish overnight. And I
increasingly had this sense that nothing was going to happen in the United States
Senate, even though many individual members were in favor. Chuck
Schumer was taking his time with all these listening meetings.
And I sensed that. And what I predicted would happen is
that nothing would happen. And that's, in fact, what has happened. So nothing
has happened. on the policy side, but all
of the things that I worried about at the Senate have kind of materialized. So
I worried that deepfakes would become a huge problem, that misinformation
would become a big problem. And we've seen all those kinds of
things happening. We've seen people manipulate
markets. When I said that, it never happened, but it's already happened
in the US and India. I guess in the U.S.
we're not sure about the motives, but we at least saw a
deepfake move in the market briefly. It's definitely happening
in other stock exchanges around the world. All the kinds of
things I was worried about are happening, and we don't have protections
against them. All the issues of bias. We now know from
an article that just came out that there's covert bias, where if you talk in
African-American English, you get a different response than if you speak
in standard American English. There's so many problems. And we
have no legislation really to address these
problems yet. There are a few bills. There's no kind
of comprehensive approach. And the problems are
just getting worse and worse. We've seen this movie
You mentioned one of those approaches, what's going on in California with SB
1047. Now, that has kind of, to me, been this perfect example
of why regulation is going to continue to be so challenging because
of the reaction to it. the whole lobbying movement against
it, the millions of dollars that are fighting the idea of this. And, you
know, it's this idea that regulation would
And I'm wondering... Yeah, the talking point is a lie. Well, exactly. Like,
sometimes regulation actually fosters
innovation. You know, that's how you get seatbelts, for example, is you have a law
that says you need We need to protect people. We wouldn't have whole industries like
commercial airlines that people were willing to take if we didn't have regulation.
So there's a certain part of Silicon Valley
that wants you to believe that lie, that regulation and innovation
are in diametric opposition. But it's not true. I mean,
we have innovation. In the car industry, this regulation doesn't
stop it. We have regulation in
drugs. That doesn't mean that everybody goes home. It's not like because we
have an FDA, all big pharma just drops out and gives up. It's
ludicrous. And at the same time, we don't want them to put any old
thing out there and have millions
of people take some drug that causes a lot of problems, which we have
seen occasionally. That's why we have a regulatory framework, so we can have innovation,
Right. It's the right kind of innovation, the innovation that
And some of that innovation needs to be towards safety. I mean, a
dirty secret of AI, and maybe it's not even that secret, is nobody knows
how to keep it safe. And nobody actually knows how to rein it in. So
we need innovation on the safety side. And you
have people like Yann LeCun that say, oh, there's no problem. But
even he, when he's pushed to be a little bit more honest, says, well,
there's no problem we can't fix. But we don't have anything encouraging people
You say in the book that the potential upside of AI is enormous,
just as big as the valley says it is. I'm
wondering to you what that upside looks like and what kind
of AI is needed to kind of access that upside. Are
we talking about a general intelligence here for that or is
Not even always machine learning. I mean, look, a calculator is
something that helps innovation in science. Always
has, always will. Just, you know, basic computation, you
know, going back to the 1940s has always helped with science. Not
everything that helps science that comes out of a
computer is going to be AI at all. Not all of it's going to be
machine learning. And, you know, there's every reason
to think that various forms of AI will continue to contribute
to science. Probably the most exciting game in town right now
is AlphaFold or AlphaFold3 from DeepMind, which
has been tremendously helpful. It's changed how people do protein science.
Everybody in the field uses it or at least knows what it is. That's
not a large language model. more complicated kind
of hybrid system with some careful domain engineering. My
concern mostly right now is with so-called large language models and
chatbots, which have gotten all the attention lately and all the
money or most of the money. Tens of billions of
dollars have gone into them. And they're not actually, I think, a particularly good
way of solving any kind of mission-critical problem. They're fun
to play with, but we're putting way too much money into them
relative to other scientific approaches to AI and other aspects
of science. I mean, people, it's crazy, like, if
an alien dropped in, they would say, these guys were doing pretty
great with science on planet Earth for a while. I mean, they were very slow to
get started, but, you know, eventually they kind of figured out the
scientific method and they're doing great. And then in the, you
know, 2120s, or sorry, 20, excuse me, in the 2020s, They
started doing this really bizarre thing where you had seven companies,
or something like that, all working on exactly the same thing, the
same kind of architecture, with the same kind of data, having the same
kind of problems, all just hoping for a miracle. And
of course that miracle didn't happen. And, you know, thank God in
2026 or whatever, they finally decided to do something different because that was
crazy. And these models were starting to be, you know, a billion dollars
a shot. You know, companies were spending five, 10, 15 billion
dollars on chips and they were all getting the same results. Like you
don't want to run the same experiment seven times. Like, you know,
good scientists like seize the trend. But here, oh, no. Like
the people, the desire for money has has overwhelmed scientific
Right. And so part of that, and you talk about this
a lot in the book as well, which is your point that money and power have
derailed AI from its original mission. And
part of that, too, is this idea of the increasing influence of the unelected tech
leaders on all of our lives where, you know, Sam Altman
can choose to put out whatever model, regardless of whatever harms
it. it may be capable of, and we all
just have to kind of deal with that. I'm wondering about that original mission.
And as you've kind of seen, it seems like AI in
that pure form of trying to help people has maybe lost its
I mean, it hasn't totally lost its way. Like, DeepMind is still, I
think, really trying to do some AI for good. But
it's largely lost its way. And I mean, it's just a
story of money and corruption and A story, I
think, of how AI went from good technical
leaders who really understood the science to people that are better at
marketing and hype and so forth. And they have been rewarded for
that marketing and hype. They've been rewarded by investors. They've
been rewarded by politicians who don't know any better. Now
they're being rewarded by Oprah. It's a
true story. the wrong things are
being rewarded. What's being rewarded right
now is people making crazy claims like that AI is
going to solve physics, which doesn't even make sense. It's not even a coherent claim.
But there's a certain group of people that wants
to feel like we're having this religious moment, we're being in this magical
thing. And so much too much power is being given to
a few people that were in the right place at the right time that can sell
those stories. And the field
of AI, I think, has suffered. It used
to be more of a research endeavor, but now it's a commercial endeavor.
And it also happens that the kind of AI that
people are building right now is very expensive to operate. And that has changed things.
So even you can see within OpenAI's history,
for example, I never loved them, but they were a more
reasonable company early on. And then they realized
that they could make progress, not that it was the only way to make progress, but that they
could make progress by making these very big models and that they were very expensive.
Yeah. And that also, uh, that endeavor kind of pushed
the idea of large language models into everyone's lives in a way of,
you know, dealing with issues of copyright, right. And
The copyright stuff is out of control. I just posted a video
yesterday. Somebody said, wow, look at this amazing new
thing. It's kind of like Dolly, but with videos out
of China. And it showed a lightsaber battle. And
the thing is that these people have obviously not paid for licensing
of Lucasfilm, or I guess maybe Disney, or whoever owns the
properties. But they've trained on it. And so it looks
very much like Star Wars characters. That is Darth Vader.
That is a lightsaber. And it's total copyright violation. Probably they
will eventually get sued. All of this stuff is actually built
on essentially all the data that companies can
scrounge, and that includes copyrighted data. And so you
have these companies that talk about, you know, they want to do AI
for the benefit of humanity, and then they sit here and
steal the property of artists and writers left and right with
no compensation. go around and they say, yeah, we want everybody to
have a universal basic income. But they don't pay licensing rights
Right, and part of that, right, is this idea that, and
you say this in the book, that the view in Silicon Valley is
that anything out there is mine for the taking, right?
And it's kind of... Yeah, and a new line which I don't
have in my book is, let all the lawyers clean it up, which is something Eric
Schmidt said in a private meeting at Stanford. I wish
they could add that in the book because I think it really captures
this whole Silicon Valley ethos right now
of Just move fast, break things, have the lawyers clean it
up. There's no attention to ethics anymore. One
of the things I talk about in the book is how Google used to say, don't be evil. That's
not even an official part of the motto anymore.
And things have really shifted in the last couple of years. I
think the general public doesn't understand how much it's shifted.
So you always had some companies that I would say were marginal on
the ethical side. Meta has never been on top
of my list of ethical companies. But Google, I
think, was more ethical than it has been of
late. Same with Microsoft. They used to talk a
lot about responsible AI. And now everybody's just trying to get market share,
Right. And that necessary shift that we're talking about towards
more ethically minded behavior and the idea of
responsible AI, is regulation the
only way to get that? Because we're talking about such a big shift in culture.
Yeah, exactly. Shifting culture. So part of the reason I wrote the
book is I would like the public to hold these companies accountable. If
the public is like, yeah, I understand that this cool
new software I'm using is pillaging artists, but I
don't really care. It's fun to use. Well, I mean, that
sets one expectation. Another expectation we
could say is, I don't want you ripping off artists, because artists are my
friends. And I don't want you ripping off writers, because writers are my friends. And I
don't want you ripping off anybody, because eventually you're going to come from me. And
so I'm going to take a stand right now and say, if
you're not going to ethically source the stuff that you're training on,
I'm not going to be part of this. Come back to me when you've got your licensing squared
away. And you know what? The companies would. It's not that all of these magical products,
and in some ways they are magical, would disappear. be
a little bit more expensive. But look what happened with Apple Music
and Napster and so forth, right? And there was
a brief period in the beginning of Napster around, I guess, around 2000, 2001, where
everybody was getting music for free. And it was really fun for them. And the artists and musicians
were getting totally screwed. And the court said the right thing, which is you need to
license. And you know what? We moved to a model where you
could have what you had in Napster, which was access to everything, almost
everything, but you paid a little bit each month so you pay
ten dollars a month or whatever for apple music and now you can listen
to almost anything and the artists get something they don't get as much as
before and i think that's unfortunate but at least they get something and
we can do that here we can have licensing
there's no reason that that's impossible the companies even
know it you know behind the scenes they are negotiating licensing deals
but their official line is like, you
have to give us this free so we can make the magical AI. We should
not believe that. And the consumers should not believe that
either. The consumers should say, hey, wait a minute, I want to be ethical
here. I want to support artists. I want to support writers. I'm not
going to use this stuff until they sort that out. That would have
Right. And part of the reason that this feels to
me like such a important moment is what you're talking about has
been built up for 20 years. You're talking about the Internet companies
that evolved into the social media companies and now they're the A.I. companies. Right.
And people didn't read the terms of service. They don't want to. A
lot of people don't care how their data is used and how other people's data
is used because data has become the kind of cost of admission to
I think we're like boiling frogs. People have come to accept way too
much. OpenAI just bought a webcam company. They want to be able to
have access to all your files, to all your calendars. And
they just put someone from the NSA on there, or used to be at the NSA, on
their board. I mean, OpenAI is,
in my view, possibly headed towards becoming a surveillance company that
knows everything about you, and then, you
know, monetizes your data. And you get nothing. You
get some free services and that's all you get out of it. And
the world has, I think, been too quick to accept those
kinds of things. And the consequence is that they're
going to, for example, be able to target political advertising to you and manipulate you.
There's new research showing, for example,
that large language models can implant false
memories in people. Like, we have no control, no checks
and balances over what the large language models are
going to teach you, essentially. And they will do it subtly, you
won't notice it, and you can, for example, wind up with false memories. This
is terrifying stuff. Everything that George
Orwell imagined in 1984 is kind of actually happening, and
we're not being loud enough about it. The reason I wrote the book is
to get the public to realize we need to say this is not acceptable. And
yet here we are, it's two months from the election, nobody's even talking about
AI policy. And the next administration is
going to set AI policy in a way that's going to last. You know, just
like once Section 230 was set in
place for social media, the rest of the movie was written at
that moment. So, like, we have to get this right, and we have to get
Speaking about the public's, the importance of the
public in this space and understanding the space, there's
been, and we talked about it, so much hype, so much
marketing, that's not really accurate to the science, right?
What are the most common, I guess, or most frustrating misconceptions about
Maybe the most frustrating is people just think that chatbots are a lot smarter than
they actually are. So they assume, for example, that
chatbots know what they're talking about. And kind of imagine
that they would like fact-check their work or something, but they don't, right?
They hallucinate constantly. In the book I have the example of
one of the systems just making up the fact, making
up the claim, that I have a pet chicken named Henrietta. And I
don't have a pet chicken named Henrietta. It would take two seconds to look
up and do a web search and see if there's any valid source,
because it's such a crazy thing. I mean, Like, I
live in a city. Where am I going to put the pet chicken? Like, it just made no sense
whatsoever. And who names their pet chicken Henrietta? And so
forth. The systems don't actually fact check. But people are
surprised. Like, every day somebody posts on Twitter, I can't believe how dumb this machine
is. Like, somehow we are not communicating enough to the general public
how dumb the machines actually are. And people get taken. Big
businesses all, you know, last year invested massively in
large language models, and then all realized it was disappointing. Over
and over again, people are learning that lesson. It's
been hard for the public to accept. And that's partly because most people have
no training. Like, how do you recognize what
a machine does, or how good it is, or that it's a machine at all? We're
very good at recognizing lions and tigers, because in the
environment of adaptation, we had to run from lions and tigers. We're not
very good at realizing we're being scammed by a bunch of
chatbots that don't know what we're talking about. Most people don't understand how
to resist that. I mean, partly the book is
Right. I think the most interesting thing
to me is kind of watching... The reason chatbots seem to
have grabbed people on and scammed people in the way you're talking about is
they seem to communicate in language. And we're just primed to
assume that if you can talk, you're a you. You're intelligent. And
And so... And then they do little things like they type words out one at
a time. The answer is actually pre-computed probably,
or could be pre-computed, but they do that to make it look more human.
Developers are trying to trick you. The people
who most want to be tricked are most seduced. The more cynical
among us might reach back at it, but
some people want to be seduced by the machines. They want to fall in love with
Yeah, the whole AI girlfriend apps is a whole thing,
but in terms of the limits of the current architecture,
large language models, the backing of generative AI,
you've talked a lot about how deep learning is hitting a wall, which we're
seeing a decent amount of evidence for, and
the idea that... Yeah, probably a better phrase would be reaching a point of diminishing
We've shifted from a regime where there was spectacular progress
over a period of about three years, like 2020 to
2023. We shifted from that to a much more incremental thing. So,
you know, GPT-4 was trained over two years ago.
Most people don't realize that. And everybody was like talking, oh,
exponential progress, AI gets better and better every day. But
we've only seen minor improvements relative to GPT-4. There's no, you
know, GPT-5 worthy thing. Every time OpenAI comes out with a
new model now, They sit there and like, well, we better not call this
GPT-5 because it's really not that much better than GPT-4. So
what should we call it? And they come out with these answers like GPT-40. Like, what
is that? You know, I think a bunch of these are efforts or
GPT-Turbo, I think was an effort probably to
make GPT-5 and then came out and they're like, yeah, this isn't
really that great. Like, it's a little bit better, but it's not that great. And
so we have hit, I mean, I interrupted your question, but I would say we
haven't absolutely hit a wall overall. There's still little improvement.
We have hit a wall on certain things. So these systems still are
not reliable. They still hallucinate and make up stuff like Henrietta,
the pet chicken. Like that is a wall. Like we have not gotten
past the wall that you don't know what you're getting out of these systems. that
we're just stuck. And then other things like, you know, the graphics get
better. So now we can make movies instead of just still shots.
So there's some progress. But on the core reliability
and reasoning and so forth, it's at best diminishing returns now.
At the same time as we're kind of noticing that, there is so much,
or seems like such a large number of people, right? And
I guess if you're on Twitter like I am, maybe the numbers are
not quite accurate, that think, as you
mentioned, that it's moving exponentially. Every new release
You know, AI influence say that stuff on Twitter and
they got like a million views for the things. Most of them would not know their
ass from an exponential. I mean, an exponential is actually a mathematical thing
where you plot a curve, you fit it and you see like that's
what it would actually mean to be exponential. They don't know
how to do that. Like they're talking about AI and it's like basic, you
know, like grade school math thing or high school math thing that
they just don't understand. Exponential does not mean
that every two months it's a little bit better than last
time. Exponential would be like what we saw before,
where you have these giant qualitative changes every few
Now something that's kind of gone in hand with
all the hype that we're seeing is the investment side
of things and the investment hype and the crazy valuations in
the markets. And as investors have started to realize that
there are diminishing returns, maybe it's not worth all this money, it's
led to the idea of the AI bubble. And you've called OpenAI
possibly the WeWork of AI, that the bubble
I was one of the first people to say there's a bubble. And by that, I
mean a bubble in the valuations. I don't think large language models
will disappear. They're like a tool. We have a lot of different tools for AI.
We need a lot more that we haven't discovered. An analogy would
be like the minute that somebody invented a power screwdriver.
They thought power screwdrivers were going to change the entire world and that
they'd be worth billions. And no, eventually the price would come back down
and, you know, people would pay 30 bucks for them. They'd still be great. People would still love them.
But, you know, they'd be a $30 tool. They wouldn't be like, you
know, constructing your entire home all by itself. And
so that's what's going to happen here. Large language models are not going anywhere.
They have some use. They're not super reliable, but they're good for
brainstorming and autocomplete when you type computer code
and stuff like that. That'll stay. But the idea that open
AI is worth $100 billion is really marginal.
So open AI, right
now it's valued at something like $80 billion. Someone's about to maybe
put in money at a $100 billion valuation. Usually
valuations are relative to profits. They actually have none.
They've not made a profit yet. This is very expensive to operate. These
tools unlike traditional software. You need these expensive chips. You
need very expensive staff You need enormous amounts of
electricity. There's no clear evidence that
they're ever going to make a profit You know if you have to pay 20 billion
dollars a year in order to stay ahead of meta
that's giving a steam stuff away for free Which is also a huge
problem for them and you're making two billion dollars a year. The math just
does not add up And so what I would imagine is at
some point, people are going to stop putting the money in. Right now, OpenAI
is a name brand, and maybe they can get
away with it for a little longer. But they have not transformed the
world in 2024 like they did in 2023. They have
demoed a lot of products that they have not actually delivered. Or
they've delivered products like GPT-4.0. Some
of the things they promised are there and some not. Sal
Khan talking about how you can use it as a tutor. There's no actual tutor
software out there. They talked about Sora, but you can't use it
at home. We don't know how much it would cost. Um, you
know, so they're making demos and now they're going to make an
announcement. Maybe it's going to be called GPT next. They make demos cause
they have learned that making demos drives up the price. But
it doesn't forever, right? So what's gonna, it's a little bit like a Ponzi scheme.
At some point, somebody's gonna say, where is
the money? And in fact, you know, I wrote an article about this a
year ago called, is generative AI
a dud? Or what if generative AI turned out to be a dud or something
like that in my sub stack? So I wrote this article at the time, I
was somewhat alone. There were a few other people maybe saying the same thing. Now, like
every week, somebody's saying that. And the thing about bubbles
is they're all about psychology. So what is a tulip actually worth?
Well, a tulip was mostly worth what people will pay for it.
And so you can, you know, for a few years, people were paying, I don't know, thousands,
tens of thousands of dollars for tulips. And then they all woke up
and said, you know, this is a little crazy. It's just a tulip. It's just a flower. You
can buy another flower for five bucks. Why are you spending, you know,
$10,000 for a tulip? It's not really worth it. And then everybody runs
for the hills. And that's what's gonna happen here, is everybody's gonna
run for the hills. So you could ask, well, who made money and who gets screwed? So the
early employees still make money because they've sold some of the stock, right?
So early employees of OpenAI do well. The
early investors make money because they take a cut of the money that's invested.
And then the people that invested in them get screwed. So you
have, for example, pension funds. And the people who operate the
pension funds make money because they've invested the pension funds
money. But the people who are actually invested in the pension funds,
the employees of California or whatever, are in the end going to get screwed
because OpenAI is most likely not going to make back
the $100 billion valuation. So like, okay, let's say they issue
stock now at $100 billion. And then next year people are
like, yeah, they're still not really making any money. And
now they need more money. They need it badly. So they take money at
a $50 billion valuation. Well, the people who put in money at
$100 billion valuation just lost half their money. So we're going to
see. And then, you know, what happens with these things is once One
thing goes down, then a lot of them go down. Everybody runs
for the hills. And so I think that's going to happen. Again, the
technology will remain, but people are going to be like,
yeah, maybe NVIDIA is not worth $3 trillion after
all, because NVIDIA has a problem. They make a good product. They
really do. I met Jensen Huang. He's a brilliant CEO.
Everything they do is good. But it's all
kind of premised on the notion that the people who buy their chips,
which are very expensive, tens of thousands of dollars, are
going to make a lot of money with those chips. And if that premise turns
out to not be true, like there's still no killer app
for generative AI. There's just conversation. If that conversation doesn't
become profits, then eventually even NVIDIA winds
up in trouble because eventually people stop placing big
orders for chips because they can't quite figure out what to do with them. I mean, you
know, NVIDIA is not a charity, right? I've
made jokes about Jensen Huang's pension fund being a
charity, but it's not, right? I mean, it's just a joke. You
know, eventually, like open AI, if they don't get
enough money to keep buying all of these chips, then NVIDIA suddenly
loses a big order and then the stock goes down. And so this is
how I think it's going to happen. I don't know if NVIDIA will be first or
last. The psychology of all of this is complicated. But the psychology is
it's not really sustainable at these valuations. It just isn't
because the profits aren't there. Now, some form of AI might be worth
trillions of dollars, which is what everybody's imagining. But generative AI
has technical problems. I don't think it's ever going to be worth that. And so
generative AI, you know, people are eventually going
In terms of those other forms of AI that you were just kind of mentioning there, I
really want to talk about the idea of new paradigms, new architectures, neurosymbolic
AI. I know you've been talking about neurosymbolic AI for a while. What
does that architecture look like and why is it different from
We don't know is the first part of the answer. We
know a general direction to look. There's every reason in the world,
and I will explain that reason. But we haven't really done the work yet, so
we don't really know exactly what it's gonna look like. And in
fact, the thing I'm gonna describe to you, neurosymbolic AI, is
part of an answer, but it's not an answer by itself. I mean, everybody is looking
for AI to be solved magically, and it's just not gonna happen.
Intelligence is, as Chas Firestone and
Brian Shull once said, not one thing but many. There's many different facets
to intelligence. And we've kind of solved one of them, which is
kind of pattern recognition. And there are others we just haven't solved
at all, like reasoning. We don't have a good answer to how to
get a machine to reason. We especially don't have a good answer about how
to get a machine to reason over open-ended problems. So in
very simple things like logic puzzles, machines are great. We've
been able to do that for 75 years pretty well. So
some of the kinds of things that like You know, your 10-year-old reads in school,
you know, A believes in B and doesn't know C, blah,
blah, blah, blah. Those kinds of things we can actually solve pretty well. But
logic in the real world where there's a lot of kind of incomplete information, reasoning
in those cases, we're not that good at. We have
some sense from this classical tradition of AI of how to do
it, but we don't know how to do it at scale when there's a lot of knowledge involved.
Many of your readers or listeners might know Daniel Kahneman's
System 1 and System 2 distinction from his book Thinking Fast
and Slow. System 1 is stuff that's automatic, fast,
reflexive, intuitive, data-driven, and System 2 is
more deliberative, more abstract, more reasoning-like. it's
pretty clear that current AI is good at system one, older
forms of AI are pretty good at system two, not perfect, and
we don't have a good way for those to communicate with each other. That's really
where the advances in AI have to come, I think, is how do you
bridge these traditions? And it's not trivial, because even once you do,
you probably have to put a lot of knowledge in machine interpretable form,
and you have to do something called extracting a cognitive model from a
real-world scene. Nobody knows a general way to do
that. So, you know, some people are like, my timeline for
AGI, meaning when I think general intelligence will come, is
like 2027, or Elon Musk says the end of 2025. And
it's so crazy I offered him a million dollar bet, which he didn't get
back to me on. And it's so crazy that a friend of mine actually upped it to
10 million dollars and still, and there's a Wall Street Journalman after Elon,
and still his people wouldn't respond. It's a completely crazy claim
to say that we will have artificial general intelligence by the end
of 2025. The more you understand the artificial intelligence
at all, the clearer that is. My training was in
how human intelligence develops. And once you look at that, like
what a child learns in the first few years of life, it just becomes
obvious that we're not there yet. We have something that sort of like vaguely approximates
it some of the time and makes complete absurd errors that no human
would ever make a lot of the time. So yes, we need to bring these
two traditions together. That's what I would call neuro-symbolic AI. Part
of the book is advocacy for that, saying, for example,
that people think that whoever has the biggest large language model
is going to win the race to AI. I would say it's whoever sponsors
the most innovation to find new things is going to win the race to
Now, in terms of general intelligence, right? And Musk
has his timelines. Musk is a hype man for everything. And,
you know, everyone seems to have their own timeline. And some of
that is tied to the idea of existential risk and
P-Doom, right? And a lot of kind of pseudoscience that
I mean, P-Doom is a real thing in a way, right? audience,
PDoOM means the probability that machines are going to kill us all. My PDoOM is
very low. Some people think it might be like 50% or
even 50% in the next three years. Strangely, some
of those run companies that might cause that if you're to believe their
story. It's a form of hype to say, oh my God, what I'm building
is so amazing and dangerous that might kill
us all. My view is we're not going to annihilate the
human species anytime soon. That that would take considerable work
that is not likely to happen. So I
don't think that scenario is going to happen. But
there's all kinds of other risks associated with AI around misinformation,
disinformation, deepfake porn, bias. I
have a list, as you know, in the book of a dozen different risks There
could be accidental escalation of war. There's many many things that
could happen short of actual extinction Right and the whole
environmental side of things as well. The the massive environmental side
the costs are huge I mean, nobody really knows where this is going that people
are talking about. Well, I have this big model I trained it on the internet didn't
really work So I'll train it on ten times the Internet or a
hundred times the Internet. Well, that's gonna cost a lot of energy I
think one training run might be I'm just making up these numbers might be
like a all the power that the country of Germany uses for
a year at some point. If you keep scaling it up, you're just using insane
amounts of power, insane amounts of water. There's lots of emissions. So
I mean, there's some work, I would say some of the more successful work, to
try to minimize that. But as the models get bigger and bigger, even
Part of what you were saying about the element of human cognition
and human intelligence, where if you study that, we are where we are.
A lot of what I've seen- And we don't just want to replicate it, by the way. Sorry
to interrupt your question, then you can go back. We don't want AI to be just like people.
People have lots of problems. You don't want a calculator to do arithmetic like I
do. I forget to carry the one. I don't pay attention. We're
not here to replicate human intelligence, but we do want to get from
human intelligence the resourcefulness. you know, you can have a
conversation with me in a conversation with someone else on a different topic and
understand them both. Or you can, you know, after we talk, you
can build an Ikea bookshelf or whatever. Like, human beings can do many
different things, even sometimes when the instructions are poor,
you know, you eventually figure it out. It's that resourcefulness of
the adaptiveness of intelligence that we would like to
get into our machines so that we can trust them when we give them new tasks
I don't know if I could do the IKEA bookshelf. It might take me a little while. But
I guess the idea of a general intelligence being possible, right? There's
a lot of debate about whether it will be possible at
I think it's certainly possible. I mean, look, your brain is
just some kind of machine, just like your heart is, you know, a
mechanical pump. Your brain is an information processor. There's
got to be some kind of Um, information processing, we haven't
got the right one, but, you know, I see no principled reason
why we can't build a general intelligence. I just don't think we're doing it
right. I mean, it'd be like, you know, if somebody had
argued in da Vinci's era, you're never going to have a helicopter. That would have been a
wrong argument. It would have turned out you couldn't do it with the materials he
had available. Right. We didn't know enough about internal combustion engines.
We didn't know enough about material science and so
forth. But it wasn't that it was impossible. It was just we we
weren't really ready to do it. That's where we are with AI is like Da Vinci and
helicopters. Like we have the vision now of what this would be like and
why it would be amazing. And we have no reason to think
it's impossible. There was no proof that you couldn't build a helicopter. There's
no proof that you can't build a general intelligence. And I'm
sure that, you know, within 200 years, we'll do it may well
do it in 20 years. I'm also sure that, you
know, we're not going to have it next year that Elon is, you know, either diluted
He did not take it. I mean, that tells you a lot, right? I mean, We
got the bet up to $10 million. It could have been like a symbolic thing. I
mean, obviously, he doesn't need the money. But the fact that he wouldn't even
respond, it doesn't bespeak
Now, with AGI, we're dealing with a hypothetical thing. As you
said, we don't know what it will look like when it will be here. And
there's been a lot of concern on the regulatory front of, you
know, there's so many active harms from AI now.
We shouldn't lose focus on that to regulate for a
potential AGI that we don't know if or when it's coming. How
important is it to kind of balance
that and prepare ourselves in legislation for
What we need to do now is to deal with the current harms
that we know about, most of which are not well handled under American law.
And we need to plant a stake in the ground so that when things
do you know, advanced, we have more intelligent systems
that we have a framework for dealing with them, which includes like communicating with
other countries about risks and sharing information about bad
incidents and so forth. So, like, I think of where we are
right now as a dress rehearsal. It's not the AI that
we're going to have 20 years from now, any more than like the first flip phone
is an iPhone, right? I mean, like the first flip phone was just as
a preview of, you know, what phones could be. And
the AI that we have now is just a preview of where AI is going.
And we don't fully glimpse it yet. But
we already see that there's pressing need to know
what to do with it. And we've already seen how the
companies have gone from publicly saying, oh, yeah, we need
regulation. We want this to be safe for everybody, saying
those things in public, like when Altman said exactly those
things next to me at the US Senate around the time we met, like
privately and sometimes even publicly opposing actual legislation coming
up with excuses like OpenAI said about the California bill what
we need this to be federal. Well that's true we do need federal
law here but like we got to start somewhere and the state law
is here it's not really going to make the federal law Harder the federal law
can supersede the state law if we need to do that like this is
a bogus argument It's an argument of the company that doesn't actually want
to be regulated despite whatever they might tell the public and like
we should be suspicious and we should start to view these companies like cigarette companies
that you know would downplay risks and try
to play the public with expensive lobbying campaigns and
you know, secretive research that, you know, they would put out
under other names and all that. Like, we should think these
guys have a lot of money at stake and they are playing us.
I mean, a whole section of the book is really about how they tend to do that,
Towards the end of the book, you answer a question that
I have asked myself many times, and you say, we
should not stop AI. We should insist that it be
made safe, better, and more trustworthy. At the same time,
there's been a lot of other, I guess, discourse on
that idea from a bunch of other people, right? There's all these categories of
folks within AI, and the kind of Doomer argument, right?
And the pause AI, and then those kinds of folks saying, shut it down now, oh
my god. And I'm wondering, like, that side of the argument, and
part of that argument is not all existential AGI. A
lot of it is focused on the current harms that we're seeing and there's no redress for
them. Is there anything of value in the idea of slowing
I think I would start by making a distinction between research and deployment. So
there is no research that I know about right now that
seems, like, so deadly that I would say, like, just don't do it. I
could imagine such a thing, but I don't... I don't see it
from what I read about. On the
other hand, are these things problematic? Should they be deployed now?
I can see a real argument saying, no, until you get your house in
order, don't deploy it. So this is what we do with
medicines, right? We say, make a safety case and
make a case that the risks outweigh, sorry, that the
benefits outweigh the risks. And if you can't do that, we don't say,
never make your drug, but we say, come back, tell
me how you're gonna mitigate the risks. Um, you
know, maybe it's a simple thing. You can just tell people, don't take it on an empty stomach
and we're good to go. And now, you know, we have a solution here. Um,
don't trust, you know, let's have a big public campaign and say, don't
trust these chatbots. I mean, that's what you need for, you know, one particular thing.
I think there are other problems that are not so easily solved. Like these systems are
clearly discriminating and they're clearly being used in job decisions
and that's not cool. And you could make an argument that the cost of
society for discriminatory behavior and hiring is
pretty high. and you could say that's a reason
why we should slow down on deployment or pause on deployment and
say look you're perfectly well in the ship software of
this sort but we need a solution here figure this out you know
it takes you six months great if it takes you two years then so be it
like come back to us when you have a handle
on this what about misinformation can you make a watermark that
actually works so we'll know what's generated by a machine can
you guarantee your stuff will be labeled you know what can you do for
us here so that Society doesn't pick up all
the costs. Another thing I keep thinking about is all
these companies that used to pump toxic chemicals into
the water and society had to pick up the cost. There's this phrase, I
don't know who made it up, I wish I had, which is to privatize the
benefits and socialize the cost. We've seen that happen a
lot of times in history and I don't want to see that with AI because I'm
part of the field. I built an AI company, I researched it
a lot. I don't want to see AI privatizing the
benefits and socializing the cost. And that's exactly what I'm
seeing. And that's why I wrote the book, is because I don't think that's fair.
In terms of the benefits, too, which part of this equation, as
we've talked about, is the benefits in terms of great
stock valuations and tons of money that's pouring into this kind of small chunk
of people running things. But on the other side, you
know, looking through the chatbot curtain to the
applications of AI and often just
machine learning that are being used, what
stuff is going on there that has you excited
or hopeful, I guess, for the positive impact that
My hope is more around things like DeepMind's AlphaFold than it
is around chatbots per se. Chatbots, to
me, have some value, but not great value. They're
good for brainstorming. You have a human in the loop who can check it. They're
good for computers, basically
for a form of autocorrect that helps coders write
faster. Even there, there's some risks, like quality
of security may go down, some studies have shown. I
worry about their use in medicine. AlphaFold
is a tool built for a problem, which is take this protein
sequence, the sequence of amino acids,
and tell me what its three-dimensional structure is. That itself turns
out to be an incredibly valuable problem because so much of biology is
really about three-dimensional jigsaw puzzles. So having that
tool, which is not a chatbot, is super helpful. And
we should be putting more money into those kinds of things. And I'm glad to see that
Google slash DeepMind has put money into those things. Chatbots themselves,
I'm just not that excited. And I see all of these negative
consequences. Or another study I saw this morning was about
tutoring. It was a math problem
thing with Turkish students, a thousand subjects, and
it was very helpful when kids were working on the practice problems,
but by the time the actual exam came, whatever benefit was there
was lost. Probably, I would hypothesize, though I'm not sure, because
chatbots sort of like, you know, give you some memorized problems
you remember in the moment, but they're not really teaching you the conceptual stuff very well,
and they're also giving you an illusion of understanding things better
than you actually do, which is not particularly healthy. But whatever reason,
like the data there on the educational benefit was actually very,
very weak. And so, you know, over and over we're seeing people
get excited about applications and they don't pan out as well as people thought.
I'm just not sure chatbots are, to use that phrase from
Star Wars, the droids we're looking for. Like there's,
undoubtedly huge benefit from AI. Two other things I love
in AI are GPS navigation directions. I
travel all the time. That's fantastic. I'd be really disappointed to
give that up. I actually love maps, but it's a pain
to work with a map when you're in the city and it's not on a grid and
whatever. And so, you know, I love GPS directions and
I love Google search or I use DuckDuckGo for privacy reasons
or whatever, but, you know, using
machine learning to help you do web search is fantastic. So
like, it's not that I don't like AI, but chatbots, you
know, if you ask me their positive value, I'm just not sure. Like
if chatbots disappeared from the planet Earth, at least until we could figure out
how to make them work as well as they pretend to work, you
know, that'd be okay with me. On Twitter the other day, I said,
I won't remember the exact word, but I basically said, chatbots answer
everything with the apparent authority of an encyclopedia. They
sound like they know what they're talking about, but the reliability of
a magic eight ball, like, I don't need that in
my life. And, you know, I'm surprised as many people do. I'd
I also read the study of the Turkish students. Very fascinating. I
would hope for a lot more studies about that kind of thing. And we're seeing a
lot of integrations of
these current systems, which, as you said, are not reliable. They do hallucinate. We're
seeing it pushed into education in a lot of concerning ways. And
you kind of talked about what's lost, right, in students learning through
whatever this unreliable tutor. Now, in
the goal to get better AI, to get AI that can reason, that is
reliable, that doesn't hallucinate. I've
been wondering if that would be such a good thing, because
right now we kind of have the backstop of we know, or if you know, you know
these systems are not reliable, don't trust them, right? The
idea of this complete and further integration of my GBT
You know, I always get painted as hating AI, but in principle I
love things like AI tutors. Like, if
they could work, you know, they didn't hallucinate anything,
they could reliably build a model of what the student
understands about the problem, you could make them in
principle cheaply enough, then you could help all kinds of
people who can't afford individual tutors right now. I think that'd be
great. Like I see no moral reason not
to do that. I could see arguments that like if you had that technology would
it be dangerous in some other way and we obviously have to look at those things
and understand the whole, but like in principle, I love these
use cases. I love the use case of, you know, home
doctor because every doctor is overburdened and many people in
the world don't have enough access to doctors. I love the idea of
a, you know, dermatology app that you can really trust
because not everybody has access. So, you know, I really want
to see this stuff work, but I just think that
what we have right now doesn't work that well. We're overselling it, that
the overselling, it has multiple problems, including the fact that
people get bad answers. The fact that there may be, I think there already is starting
to be public pushback. You know, I think in some ways that Silicon
Valley is its own worst enemy right now by constantly overselling things.
We're getting to like a boy who cried wolf thing. Like if somebody comes
along now with a perfect home doctor in an app, A
lot of people are going to be like, yeah, I don't know. I tried this thing
a couple of years ago, and it told me to take this medicine, and
I got really sick, and forget it. Or with
driverless cars, we're pushing them out too fast. If we suddenly put
millions of them on the road, they would have accidents every day, and people would die, and
people might cancel the whole thing. And it's better that we have at
least somewhat of a phased rollout. overselling
this stuff has a consequence, and it just doesn't work
that well yet. We may see in software, where people have
been using a lot for coding, that the code can't be maintained very
well. So in software, one challenge is to write code that
works, and another is to come back to it a year, five years, 10 years,
20 years later, and have it still work, and be able to change
it, because circumstances change. The most famous example of this is probably the
Y2K problem, where people Program dates
with two digits instead of four, and it cost millions of dollars
of work had to be done. The public doesn't know too much about it anymore because
they actually managed to solve that problem. It was a big mess at the time. Every
piece of software breaks eventually because something changes. You're
calling some other piece of software. And you need, the
thing about programming is like in the moment that you write it, you know how it works. But then
if you come back later, you want your code to be clear, well documented, et
cetera, et cetera. There's a big risk We
call it technical risk or technical debt that the code that people are
going to write with these machines They don't really understand it and it's
kind of like kludge together. I mean hodgepodge together and
You know, we have trouble fixing it. So we may see some downstream costs as
Right, we're talking about all these problems. And there's so many.
And I think for a lot of people, it's really overwhelming and
frightening in ways that range from, I'm
going to lose my job to, oh my God, what's going to happen? I've
seen the Terminator movie, right? Whether or not that's legit. What
should people be doing here? How do people process this?
I would like to see people get loud about
this, talk to their congresspeople, write op-eds,
maybe consider boycotts. So, you know, one that I suggest at
the end of the book, and I'm going to set something up on this at
teamingsiliconvalley.org, is maybe
we should boycott any art that isn't properly licensed.
I mean, any, sorry, any generative AI that isn't, that's
using art that isn't properly licensed. Right? We
should just say, look, as consumers, we want to have all
of this stuff be ethically sourced. And we'll just wait. We'll
sit and wait until you do that. And so I think
collective action here to say, we want AI
to be done in an ethical way, in a way that
is good for everybody and not just a few people. We're going to stand by
that. Like, if we could actually get enough people To
agree on that, we could change Silicon
Valley. Silicon Valley used to, I think, care
more about the consumers. And nowadays has an attitude of like,
yeah, we're just gonna make money off those people. And we
Yeah, absolutely. Well, Gary, I really appreciate your time. This
Creators and Guests
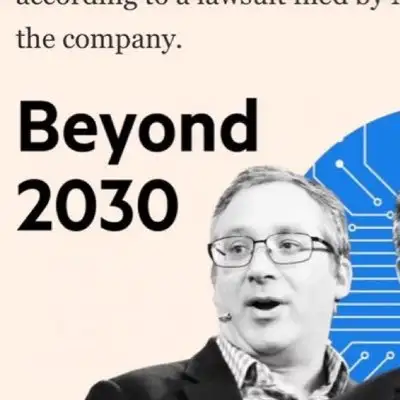
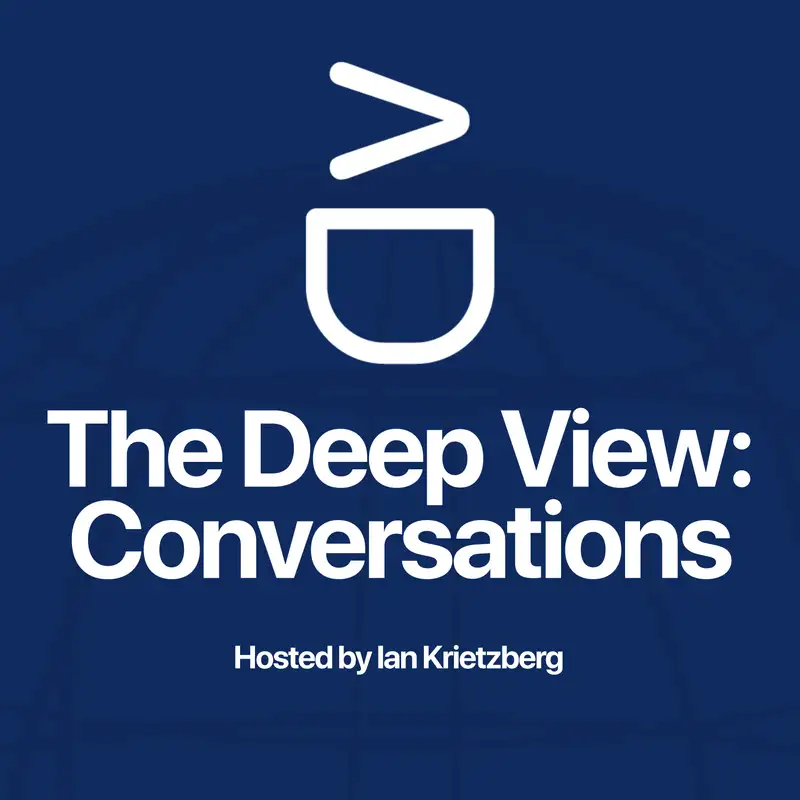